Proving Information Inequalities and Identities with Symbolic Computation
International Symposium on Information Theory (ISIT)(2022)
Abstract
Proving linear inequalities and identities of Shannon’s information measures, possibly with linear constraints on the information measures, is an important problem in information theory. For this purpose, ITIP and other variant algorithms have been developed and implemented, which are all based on solving a linear program (LP). In particular, an identity f = 0 is verified by solving two LPs, one for f ≥ 0 and one for f ≤ 0. In this paper, we develop a set of algorithms that can be implemented by symbolic computation. Based on these algorithms, procedures for verifying linear information inequalities and identities are devised. Compared with LP-based algorithms, our procedures can produce analytical proofs that are both human-verifiable and free of numerical errors. Our procedures are also more efficient computationally. For constrained inequalities, by taking advantage of the algebraic structure of the problem, the size of the LP that needs to be solved can be significantly reduced. For identities, instead of solving two LPs, the identity can be verified directly with very little computation.
MoreTranslated text
Key words
information inequalities,identities,computation
AI Read Science
Must-Reading Tree
Example
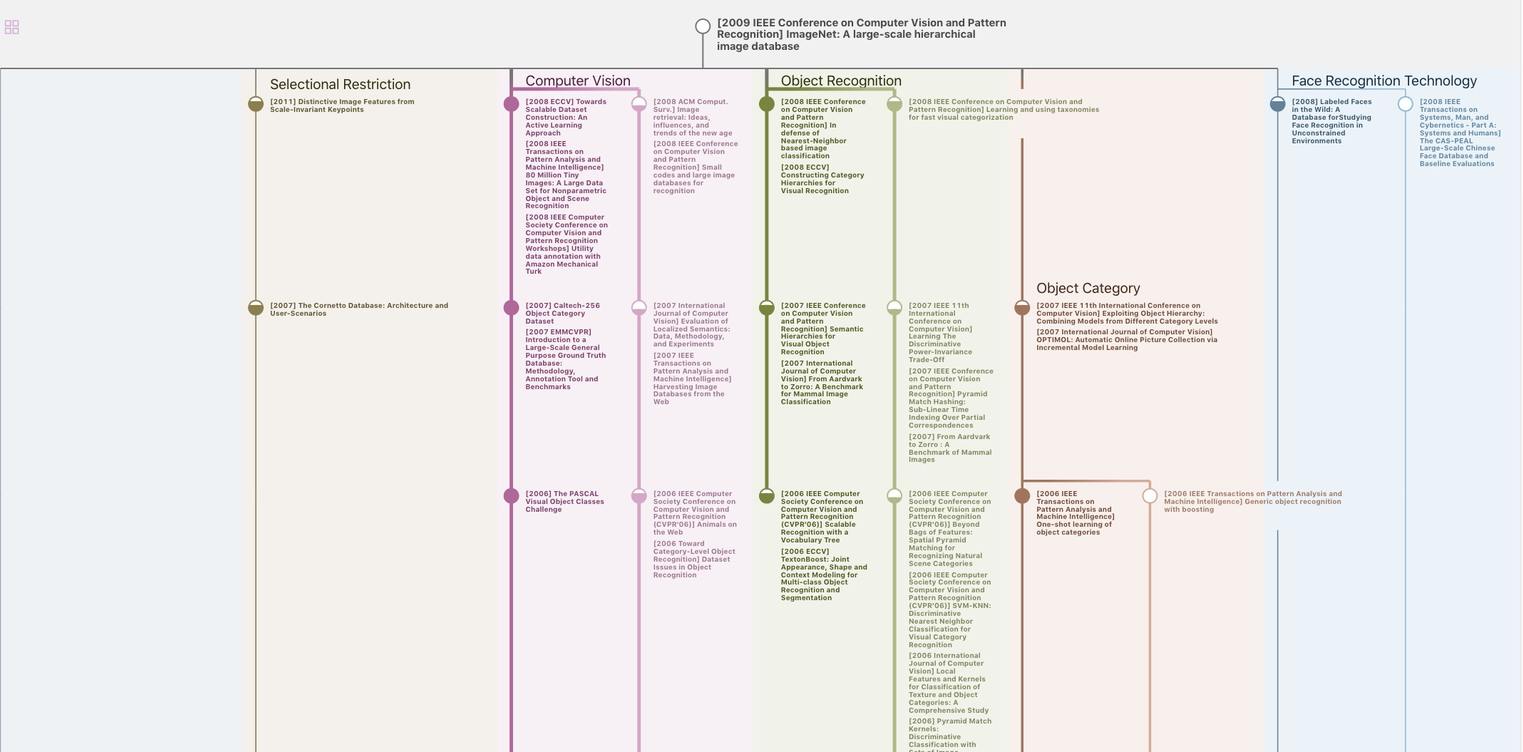
Generate MRT to find the research sequence of this paper
Chat Paper
Summary is being generated by the instructions you defined