From Discrimination to Generation: Knowledge Graph Completion with Generative Transformer
International Workshop on Multimodal Human Understanding for the Web and Social Media(2022)
Abstract
Knowledge graph completion aims to address the problem of extending a KG with missing triples. In this paper, we provide an approach GenKGC, which converts knowledge graph completion to sequence-to-sequence generation task with the pre-trained language model. We further introduce relation-guided demonstration and entity-aware hierarchical decoding for better representation learning and fast inference. Experimental results on three datasets show that our approach can obtain better or comparable performance than baselines and achieve faster inference speed compared with previous methods with pre-trained language models. We also release a new large-scale Chinese knowledge graph dataset AliopenKG500 for research purpose. Code and datasets are available in https://github.com/zjunlp/PromptKG/tree/main/GenKGC.
MoreTranslated text
Key words
Knowledge Graph Embedding,Scene Graph Generation,Signal Processing on Graphs,Representation Learning,Sequence-to-Sequence Learning
AI Read Science
Must-Reading Tree
Example
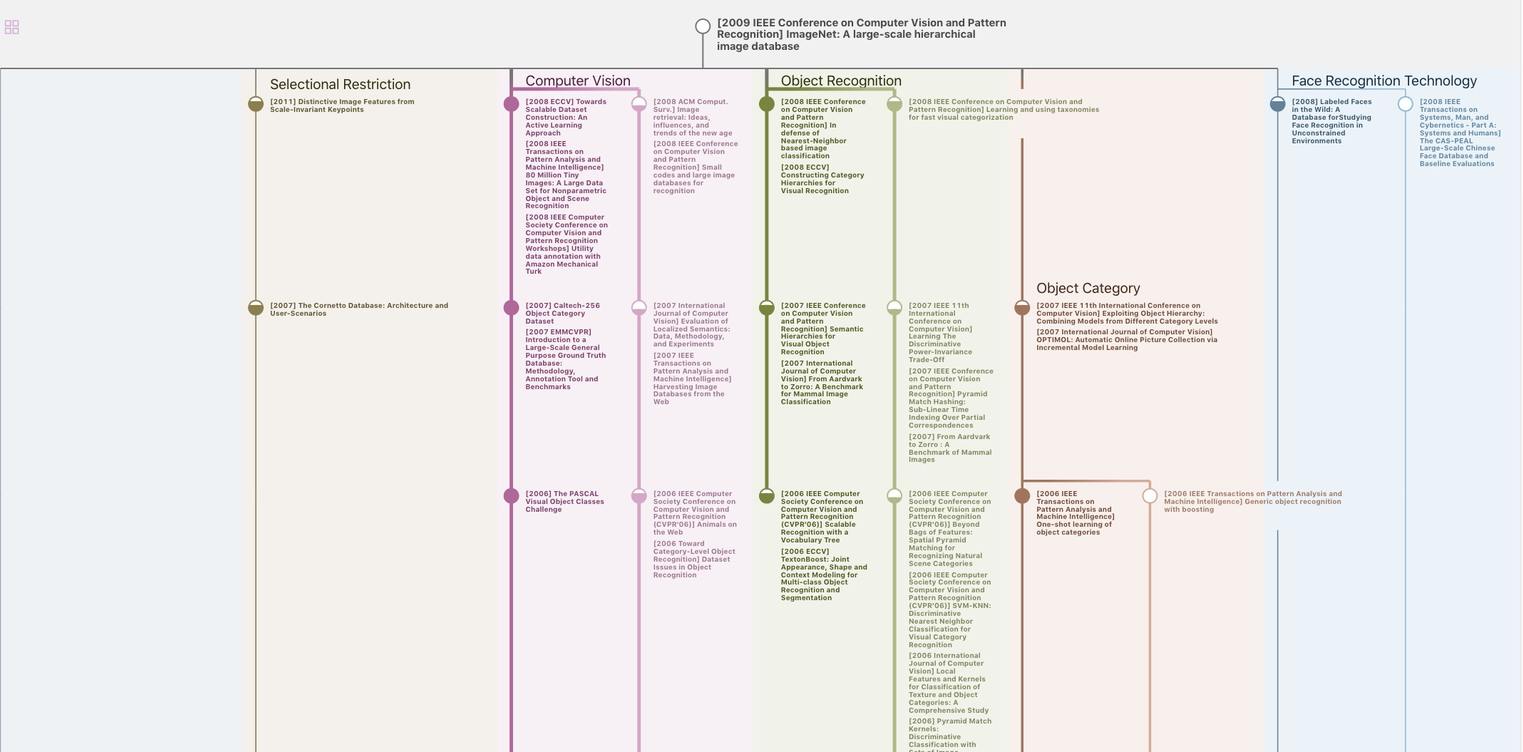
Generate MRT to find the research sequence of this paper
Chat Paper
Summary is being generated by the instructions you defined