Exploiting Independent Instruments: Identification and Distribution Generalization.
International Conference on Machine Learning(2022)
摘要
Instrumental variable models allow us to identify a causal function between covariates $X$ and a response $Y$, even in the presence of unobserved confounding. Most of the existing estimators assume that the error term in the response $Y$ and the hidden confounders are uncorrelated with the instruments $Z$. This is often motivated by a graphical separation, an argument that also justifies independence. Positing an independence restriction, however, leads to strictly stronger identifiability results. We connect to the existing literature in econometrics and provide a practical method called HSIC-X for exploiting independence that can be combined with any gradient-based learning procedure. We see that even in identifiable settings, taking into account higher moments may yield better finite sample results. Furthermore, we exploit the independence for distribution generalization. We prove that the proposed estimator is invariant to distributional shifts on the instruments and worst-case optimal whenever these shifts are sufficiently strong. These results hold even in the under-identified case where the instruments are not sufficiently rich to identify the causal function.
更多查看译文
关键词
independent instruments,generalization,distribution
AI 理解论文
溯源树
样例
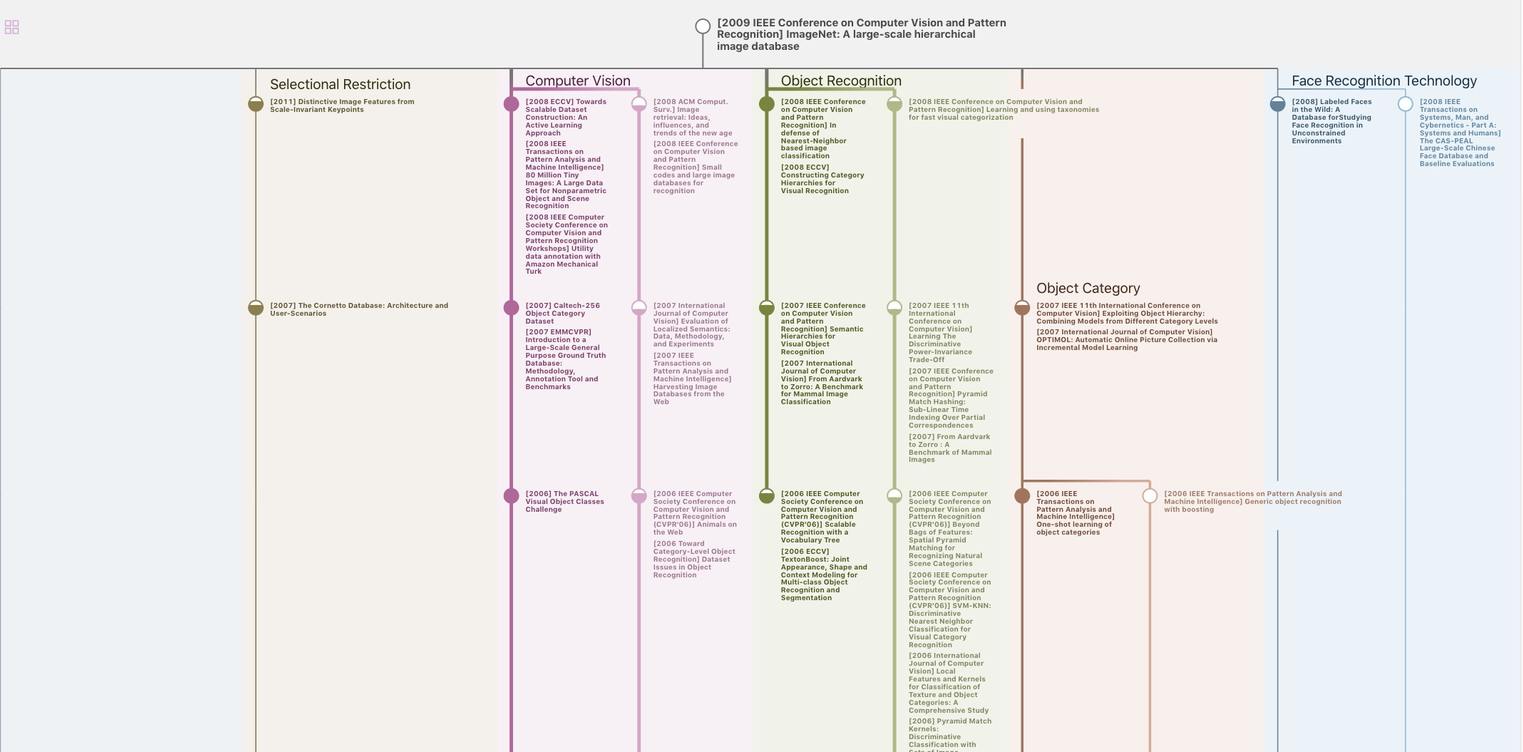
生成溯源树,研究论文发展脉络
Chat Paper
正在生成论文摘要