Hierarchical graph attention network for miRNA-disease association prediction
Molecular Therapy(2022)
摘要
Many biological studies show that the mutation and abnormal expression of microRNAs (miRNAs) could cause a variety of diseases. As an important biomarker for disease diagnosis, miRNA is helpful to understand pathogenesis, and could promote the identification, diagnosis and treatment of diseases. However, the pathogenic mechanism how miRNAs affect these diseases has not been fully understood. Therefore, predicting the potential miRNA-disease associations is of great importance for the development of clinical medicine and drug research. In this study, we proposed a novel deep learning model based on hierarchical graph attention network for predicting miRNA-disease associations (HGANMDA). Firstly, we constructed a miRNA-disease-lncRNA heterogeneous graph based on known miRNA-disease associations, miRNA-lncRNA associations and disease-lncRNA associations. Secondly, the node-layer attention was applied to learn the importance of neighbor nodes based on different meta-paths. Thirdly, the semantic-layer attention was applied to learn the importance of different meta-paths. Finally, a bilinear decoder was employed to reconstruct the connections between miRNAs and diseases. The extensive experimental results indicated that our model achieved good performance and satisfactory results in predicting miRNA-disease associations.
更多查看译文
关键词
miRNA,disease,hierarchical graph attention network,lncRNA,meta-path
AI 理解论文
溯源树
样例
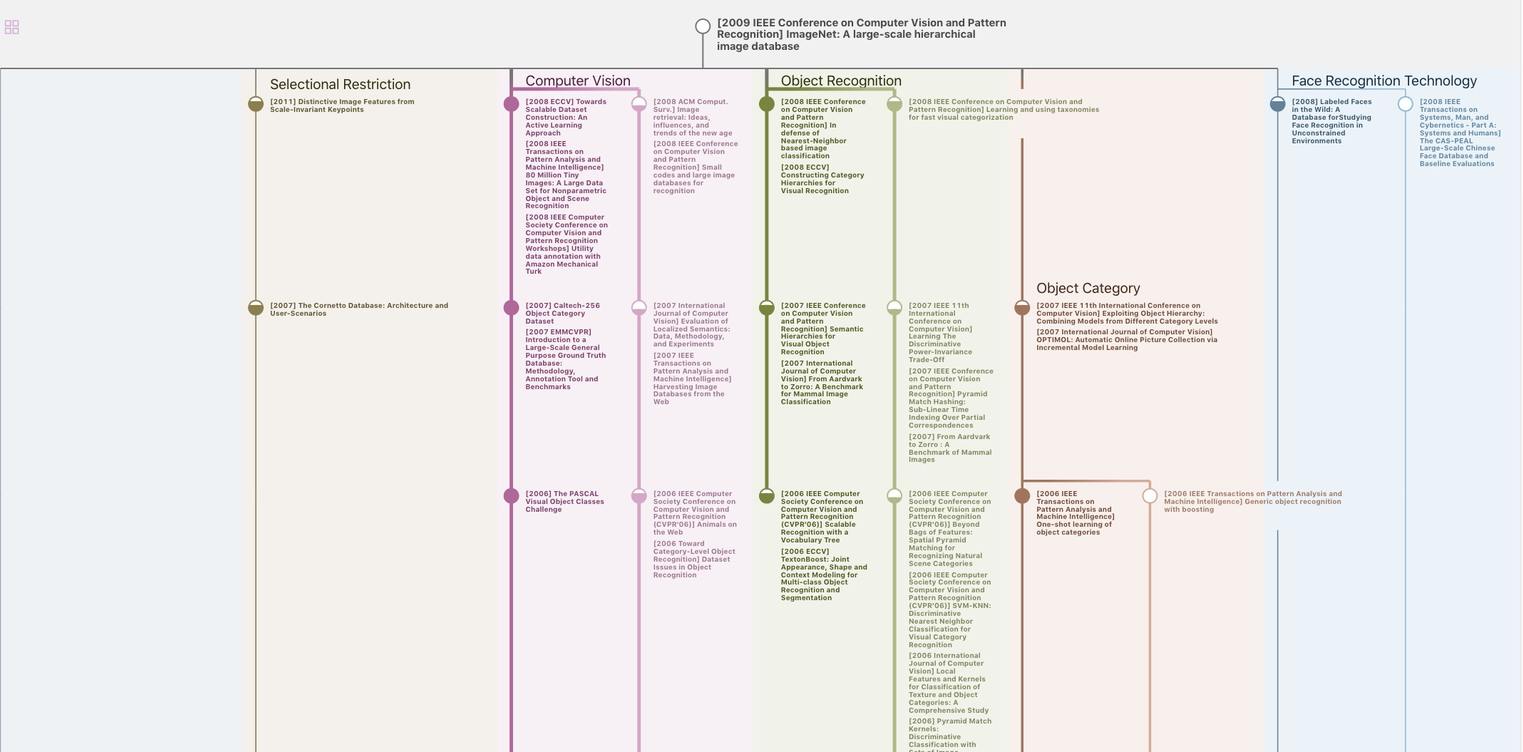
生成溯源树,研究论文发展脉络
Chat Paper
正在生成论文摘要