Maximum Likelihood Reconstruction of Water Cherenkov Events With Deep Generative Neural Networks
FRONTIERS IN BIG DATA(2022)
Abstract
Large water Cherenkov detectors have shaped our current knowledge of neutrino physics and nucleon decay, and will continue to do so in the foreseeable future. These highly capable detectors allow for directional and topological, as well as calorimetric information to be extracted from signals on their photosensors. The current state-of-the-art approach to water Cherenkov reconstruction relies on maximum-likelihood estimation, with several simplifying assumptions employed to make the problem tractable. In this paper, we describe neural networks that produce probability density functions for the signals at each photosensor, given a set of inputs that characterizes a particle in the detector. The neural networks we propose allow for likelihood-based approaches to event reconstruction with significantly fewer assumptions compared to traditional methods, and are thus expected to improve on the current performance of water Cherenkov detectors.
MoreTranslated text
Key words
experimental particle physics,event reconstruction,water Cherenkov detectors,generative models,convolutional neural network
AI Read Science
Must-Reading Tree
Example
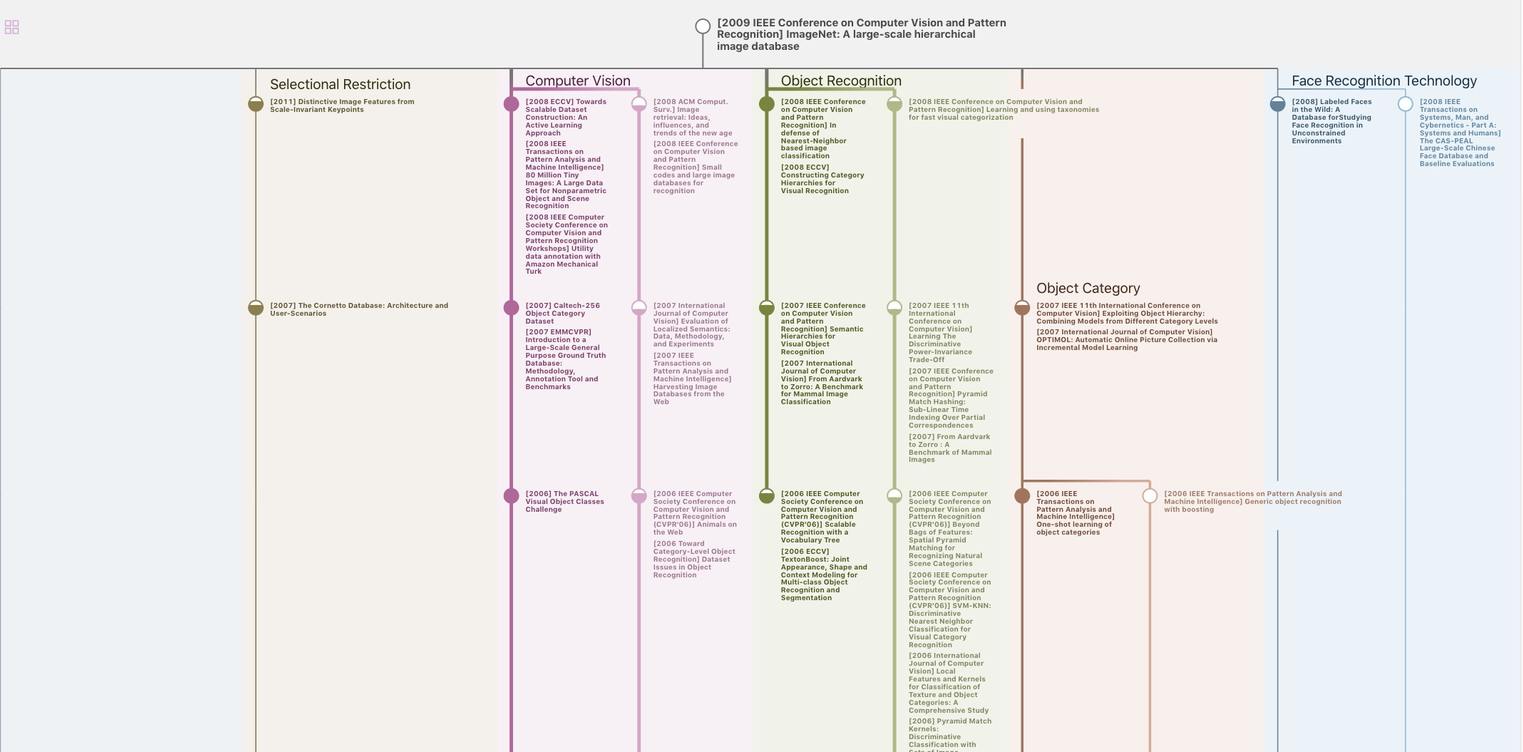
Generate MRT to find the research sequence of this paper
Chat Paper
Summary is being generated by the instructions you defined