A multi-task two-path deep learning system for predicting the invasiveness of craniopharyngioma
Computer Methods and Programs in Biomedicine(2022)
摘要
Background and Objective: Craniopharyngioma is a kind of benign brain tumor in histography. However, it might be clinically aggressive and have severe manifestations, such as increased intracranial pressure, hypothalamic-pituitary dysfunction, and visual impairment. It is considered challenging for radiologists to predict the invasiveness of craniopharyngioma through MRI images. Therefore, developing a non-invasive method that can predict the invasiveness and boundary of CP as a reference before surgery is of clinical value for making more appropriate and individualized treatment decisions and reducing the occurrence of inappropriate surgical plan choices.Methods: The MT-Brain system has consisted of two pathways, a sub-path based on 2D CNN for capturing the features from each slice of MRI images, and a 3D sub-network for capturing additional context information between slices. By introducing the two-path architecture, our system can make full use of the fusion of the above 2D and 3D features for classification. Furthermore, position encoding and mask-guided attention also have been introduced to improve the segmentation and diagnosis performance. To verify the performance of the MT-Brain system, we have enrolled 1032 patients with craniopharyngioma (302 invasion and 730 non-invasion patients), segmented the tumors on postcontrast coronal T1WI and randomized them into a training dataset and a testing dataset at a ratio of 8:2.Results: The MT-Brain system achieved a remarkable performance in diagnosing the invasiveness of craniopharyngioma with the AUC of 83.84%, the accuracy of 77.94%, the sensitivity of 70.97%, and the specificity of 80.99%. In the lesion segmentation task, the predicted boundaries of lesions were similar to those labeled by radiologists with the dice of 66.36%. In addition, some explorations also have been made on the interpretability of deep learning models, illustrating the reliability of the model.Conclusions: To the best of our knowledge, this study is the first to develop an integrated deep learning model to predict the invasiveness of craniopharyngioma preoperatively and locate the lesion boundary synchronously on MRI. The excellent performances indicate that the MT-Brain system has great potential in real-world clinical applications.
更多查看译文
关键词
Craniopharyngioma,MRI Imaging,Deep learning,Invasiveness diagnosis,Lesion segmentation
AI 理解论文
溯源树
样例
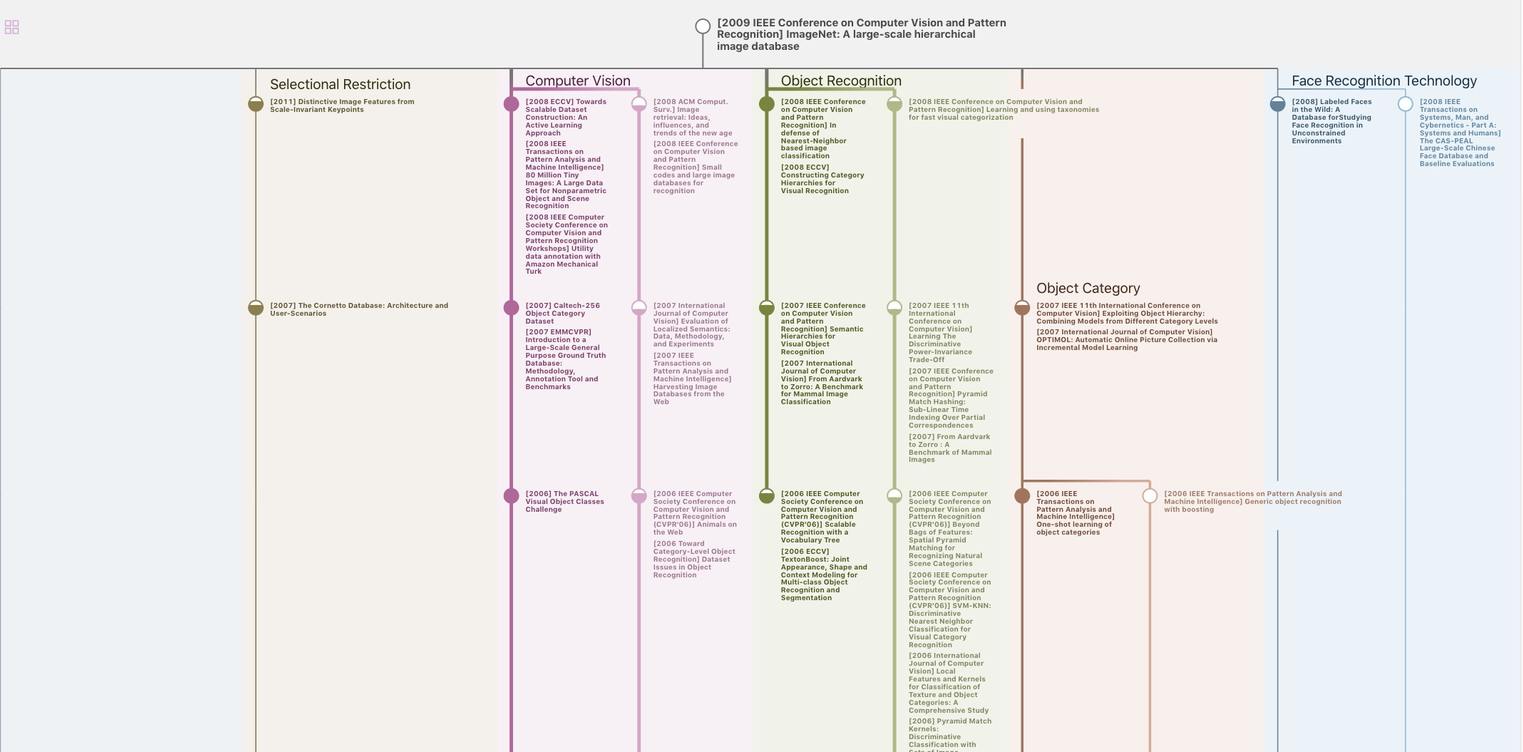
生成溯源树,研究论文发展脉络
Chat Paper
正在生成论文摘要