Low-Rank and Row-Sparse Decomposition for Joint DOA Estimation and Distorted Sensor Detection.
arxiv(2023)
摘要
Distorted sensors could occur randomly and may lead to the breakdown of a sensor array system. In this article, we consider an array model within which a small number of sensors are distorted by unknown sensor gain and phase errors. With such an array model, the problem of joint direction-of-arrival (DOA) estimation and distorted sensor detection is formulated under the framework of low-rank and row-sparse decomposition. We derive an iteratively reweighted least squares (IRLS) algorithm to solve the resulting problem. The convergence property of the IRLS algorithm is analyzed by means of the monotonicity and boundedness of the objective function. Extensive simulations are conducted regarding parameter selection, convergence speed, computational complexity, and performances of DOA estimation as well as distorted sensor detection. Even though the IRLS algorithm is slightly worse than the alternating direction method of multipliers in detecting the distorted sensors, the results show that our approach outperforms several state-of-the-art techniques in terms of convergence speed, computational cost, and DOA estimation performance.
更多查看译文
关键词
joint doa estimation,distorted sensor detection,low-rank,row-sparse
AI 理解论文
溯源树
样例
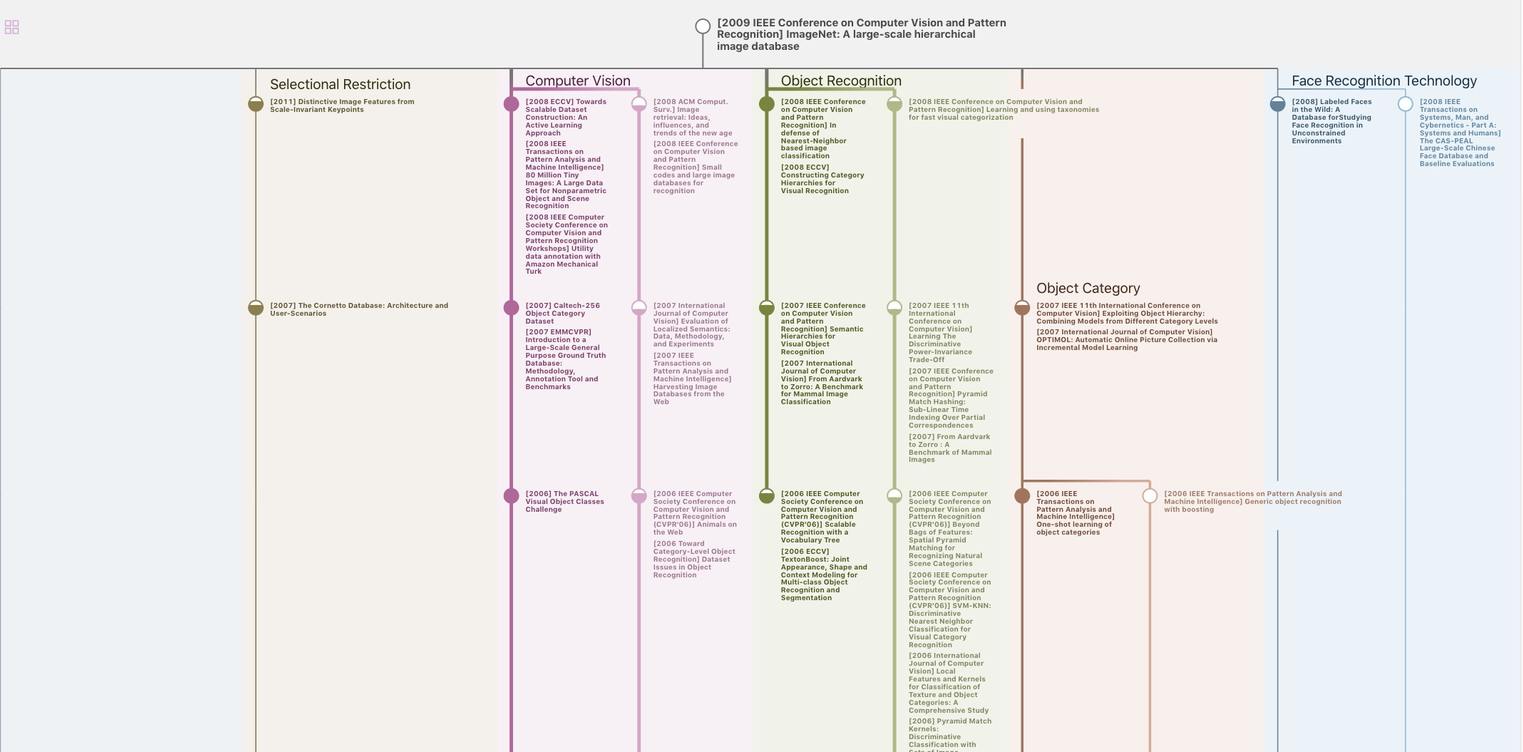
生成溯源树,研究论文发展脉络
Chat Paper
正在生成论文摘要