Wasserstein Generative Adversarial Networks Based Differential Privacy Metaverse Data Sharing.
IEEE journal of biomedical and health informatics(2023)
Abstract
Although differential privacy metaverse data sharing can avoid privacy leakage of sensitive data, randomly perturbing local metaverse data will lead to an imbalance between utility and privacy. Therefore, this work proposed models and algorithms of differential privacy metaverse data sharing using Wasserstein generative adversarial networks (WGAN). Firstly, this study constructed the mathematical model of differential privacy metaverse data sharing by introducing appropriate regularization term related to generated data's discriminant probability into WGAN. Secondly, we established basic model and algorithm for differential privacy metaverse data sharing using WGAN based on the constructed mathematical model, and theoretically analyzed basic algorithm. Thirdly, we established federated model and algorithm for differential privacy metaverse data sharing using WGAN by serialized training based on basic model, and theoretically analyzed federated algorithm. Finally, based on utility and privacy metrics, we conducted a comparative analysis for the basic algorithm of differential privacy metaverse data sharing using WGAN, and experimental results validate theoretical results, which show that algorithms of differential privacy metaverse data sharing using WGAN maintaining equilibrium between privacy and utility.
MoreTranslated text
Key words
Metaverse data sharing,differential privacy,privacy-utility equilibrium,Wasserstein generative adversarial networks,federated learning
AI Read Science
Must-Reading Tree
Example
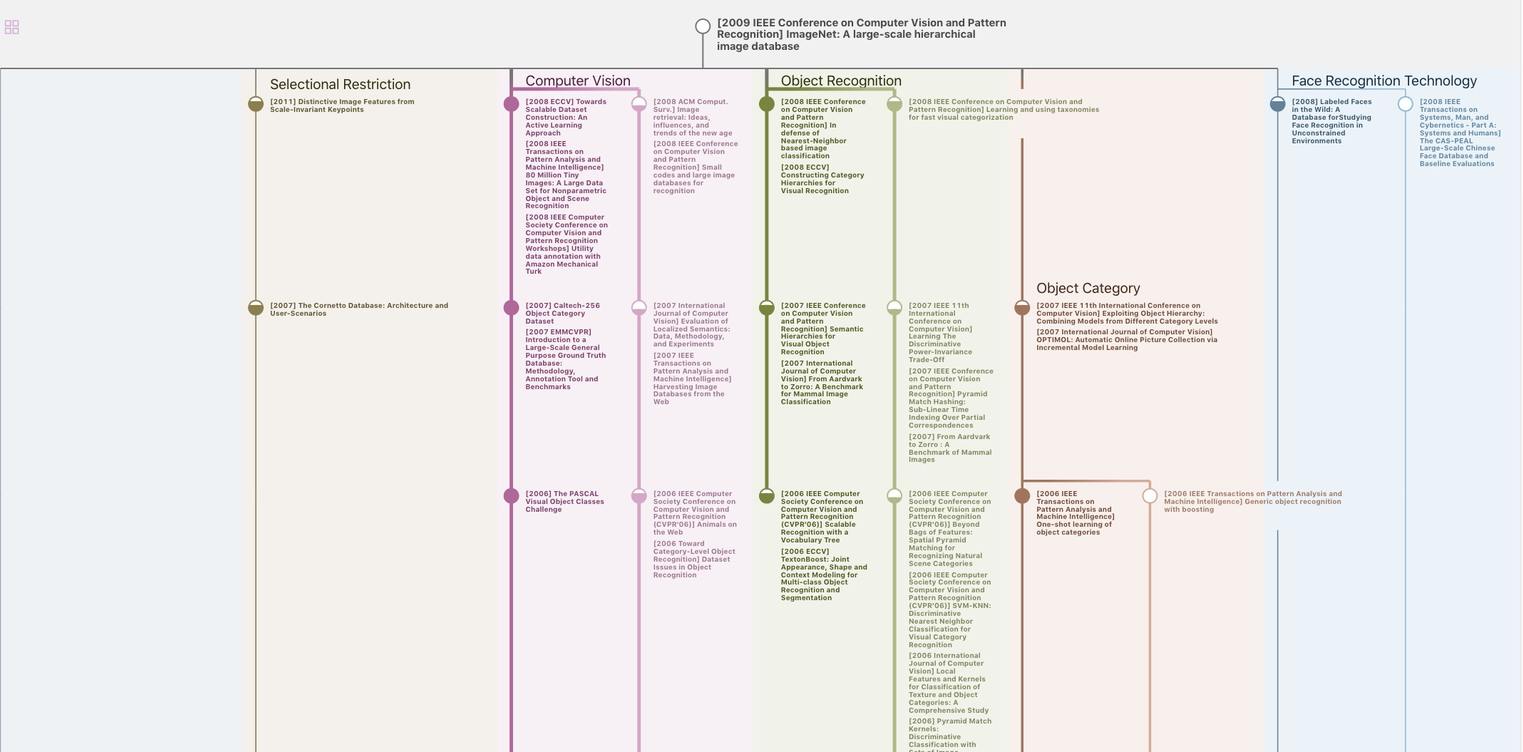
Generate MRT to find the research sequence of this paper
Chat Paper
Summary is being generated by the instructions you defined