Performance optimization of support vector machine with oppositional grasshopper optimization for acute appendicitis diagnosis
COMPUTERS IN BIOLOGY AND MEDICINE(2022)
摘要
Preoperative differentiation of complicated and uncomplicated appendicitis is challenging. The research goal was to construct a new intelligent diagnostic rule that is accurate, fast, noninvasive, and cost-effective, distinguishing between complicated and uncomplicated appendicitis. Overall, 298 patients with acute appendicitis from the Wenzhou Central Hospital were recruited, and information on their demographic characteristics, clinical findings, and laboratory data was retrospectively reviewed and applied in this study. First, the most significant variables, including C-reactive protein (CRP), heart rate, body temperature, and neutrophils discriminating complicated from uncomplicated appendicitis, were identified using random forest analysis. Second, an improved grasshopper optimization algorithm-based support vector machine was used to construct the diagnostic model to discriminate complicated appendicitis (CAP) from uncomplicated appendicitis (UAP). The resultant optimal model can produce an average of 83.56% accuracy, 81.71% sensitivity, 85.33% specificity, and 0.6732 Matthews correlation coefficients. Based on existing routinely available markers, the proposed intelligent diagnosis model is highly reliable. Thus, the model can potentially be used to assist doctors in making correct clinical decisions.
更多查看译文
关键词
Support vector machine, Feature selection, Grasshopper optimization algorithm, Appendicitis diagnosis, Opposition-based learning
AI 理解论文
溯源树
样例
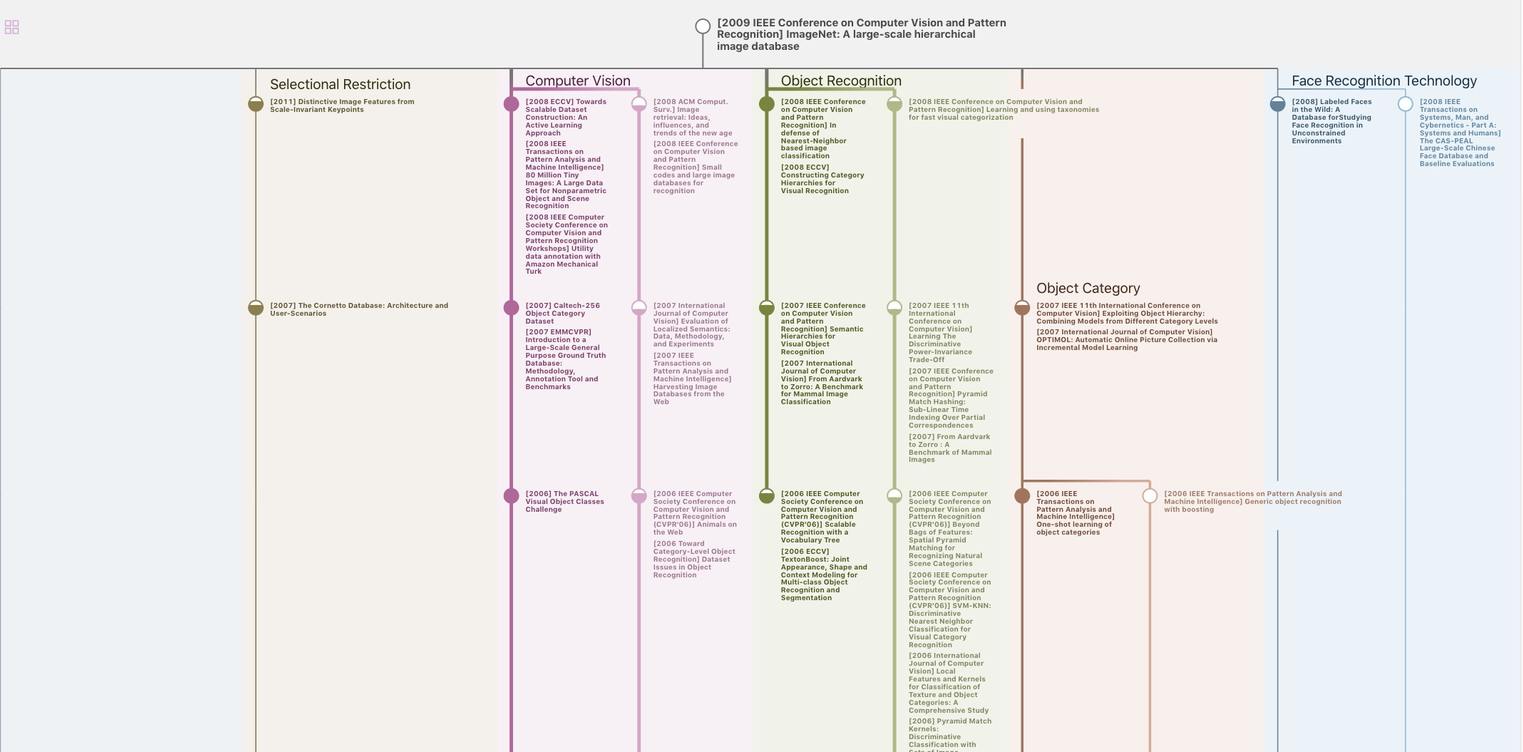
生成溯源树,研究论文发展脉络
Chat Paper
正在生成论文摘要