Dosimetric impact of deep learning-based CT auto-segmentation on radiation therapy treatment planning for prostate cancer
RADIATION ONCOLOGY(2022)
摘要
Background The evaluation of automatic segmentation algorithms is commonly performed using geometric metrics. An analysis based on dosimetric parameters might be more relevant in clinical practice but is often lacking in the literature. The aim of this study was to investigate the impact of state-of-the-art 3D U-Net-generated organ delineations on dose optimization in radiation therapy (RT) for prostate cancer patients. Methods A database of 69 computed tomography images with prostate, bladder, and rectum delineations was used for single-label 3D U-Net training with dice similarity coefficient (DSC)-based loss. Volumetric modulated arc therapy (VMAT) plans have been generated for both manual and automatic segmentations with the same optimization settings. These were chosen to give consistent plans when applying perturbations to the manual segmentations. Contours were evaluated in terms of DSC, average and 95
更多查看译文
关键词
3D U-Net, Automatic segmentation, Radiation therapy, Prostate cancer, Neural networks, Deep learning
AI 理解论文
溯源树
样例
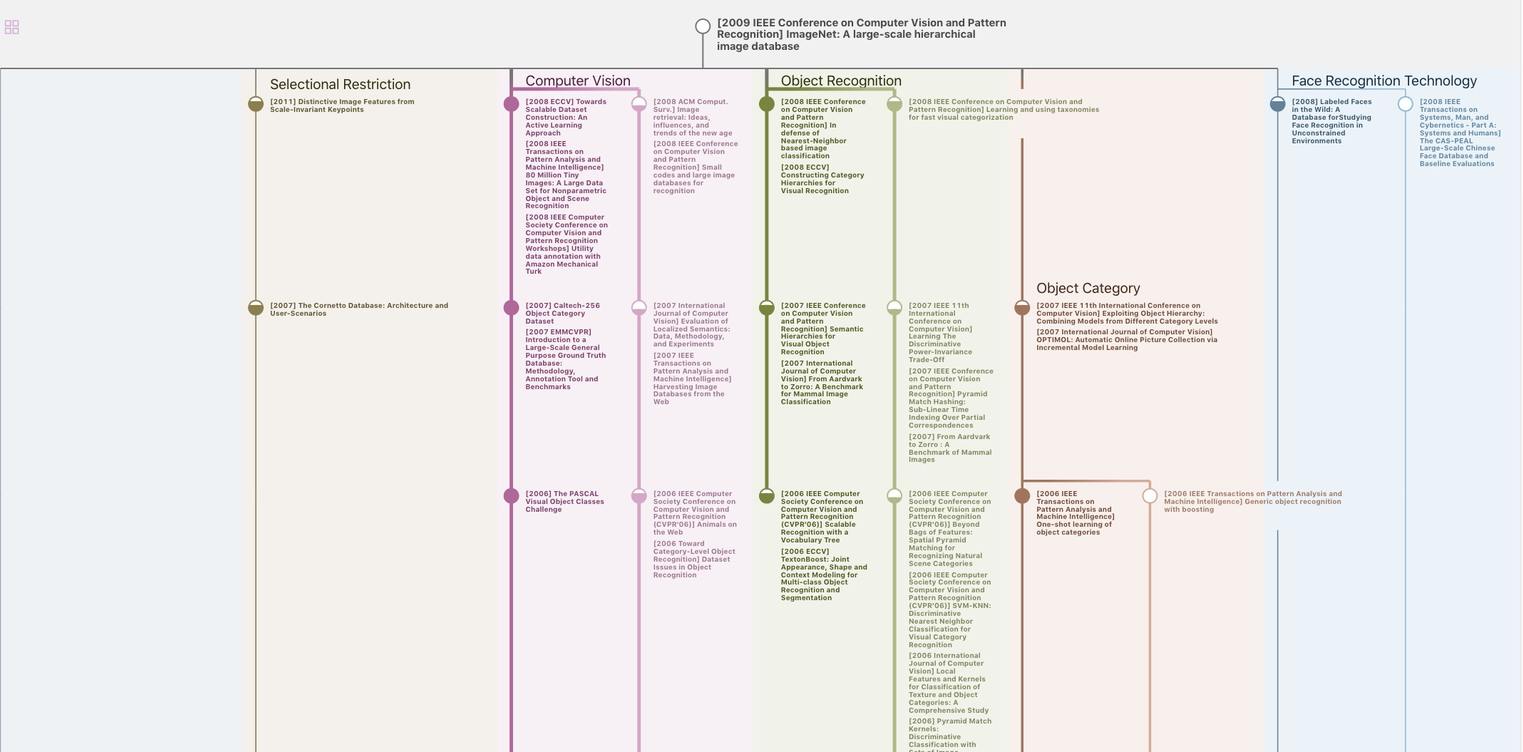
生成溯源树,研究论文发展脉络
Chat Paper
正在生成论文摘要