ECCA: Efficient Correntropy-Based Clustering Algorithm With Orthogonal Concept Factorization
IEEE transactions on neural networks and learning systems(2023)
摘要
One of the hottest topics in unsupervised learning is how to efficiently and effectively cluster large amounts of unlabeled data. To address this issue, we propose an orthogonal conceptual factorization (OCF) model to increase clustering effectiveness by restricting the degree of freedom of matrix factorization. In addition, for the OCF model, a fast optimization algorithm containing only a few low-dimensional matrix operations is given to improve clustering efficiency, as opposed to the traditional CF optimization algorithm, which involves dense matrix multiplications. To further improve the clustering efficiency while suppressing the influence of the noises and outliers distributed in real-world data, an efficient correntropy-based clustering algorithm (ECCA) is proposed in this article. Compared with OCF, an anchor graph is constructed and then OCF is performed on the anchor graph instead of directly performing OCF on the original data, which can not only further improve the clustering efficiency but also inherit the advantages of the high performance of spectral clustering. In particular, the introduction of the anchor graph makes ECCA less sensitive to changes in data dimensions and still maintains high efficiency at higher data dimensions. Meanwhile, for various complex noises and outliers in real-world data, correntropy is introduced into ECCA to measure the similarity between the matrix before and after decomposition, which can greatly improve the clustering effectiveness and robustness. Subsequently, a novel and efficient half-quadratic optimization algorithm was proposed to quickly optimize the ECCA model. Finally, extensive experiments on different real-world datasets and noisy datasets show that ECCA can archive promising effectiveness and robustness while achieving tens to thousands of times the efficiency compared with other state-of-the-art baselines.
更多查看译文
关键词
Matrix decomposition,Clustering algorithms,Robustness,Optimization,Clustering methods,Linear programming,Noise measurement,Anchor graph,clustering,correntropy,orthogonal conceptual factorization (OCF)
AI 理解论文
溯源树
样例
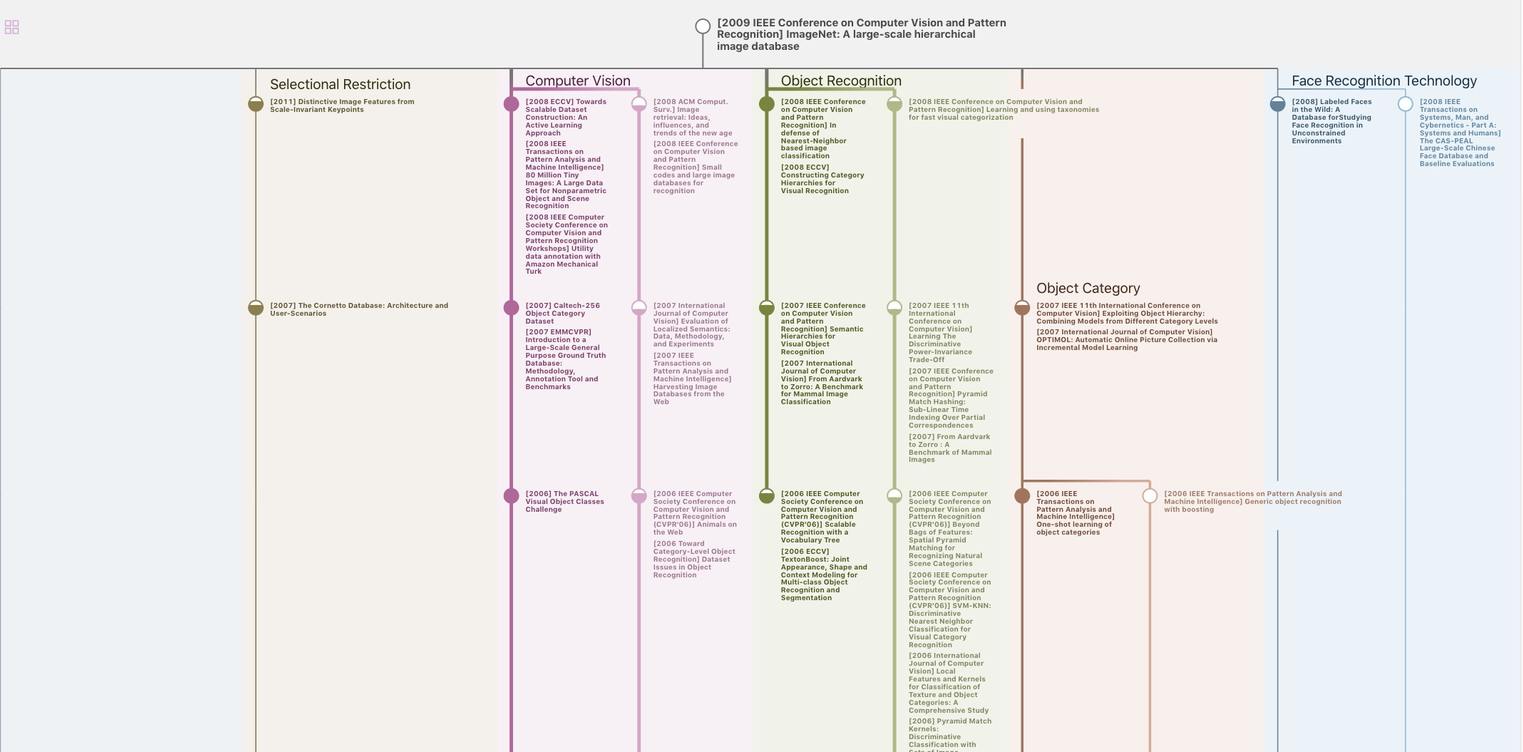
生成溯源树,研究论文发展脉络
Chat Paper
正在生成论文摘要