Anomaly Detection for the Industrial Internet of Things: an Unsupervised Approach for Fast Root Cause Analysis
2022 IEEE Conference on Control Technology and Applications (CCTA)(2022)
摘要
The Industrial Internet of Things is one of the application area where Machine Learning has been most successful recently. In such context, typically Machine Learning-based solutions are consumed by users in the context of Decision Support Systems. Unfortunately, a major drawback of many Machine Learning approaches is that they calculate predictions but provide no explanations, which represent a major limitation in driving decision making: users may find predictions hard to understand and trust. Ultimately, this may prevent them from adopting Machine Learning-powered tools and turning their predictions into actionable insight. In this work, we propose an unsupervised anomaly detection system able to detect anomalies and explain predictions, thus enabling root cause analysis. The deployment of Industrial Internet of Things monitoring solutions through Decision Support Systems requires efficiency and very low latency. To achieve the goal, we combine Isolation Forest with a fast, model-agnostic interpretability approach, called Accelerated-agnostic Explanations (AcME). We evaluate the efficacy of the proposed approach in two real industrial cases.
更多查看译文
关键词
Anomaly Detection,Explainable Artificial Intelligence,Interpretable Machine learning,Industry 4.0,Industrial Internet of Things,Isolation Forest,Unsupervised Learning
AI 理解论文
溯源树
样例
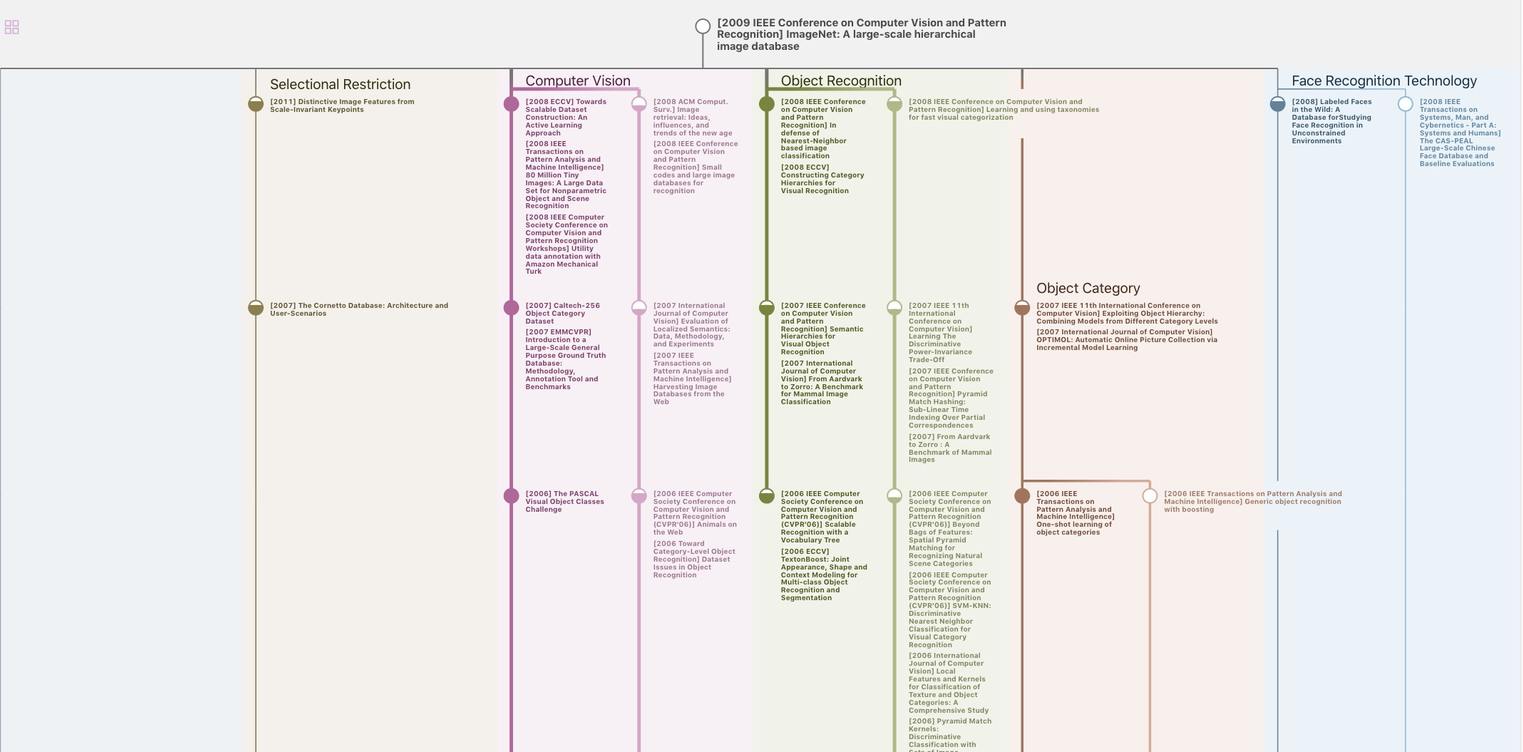
生成溯源树,研究论文发展脉络
Chat Paper
正在生成论文摘要