Few-Bit Backward: Quantized Gradients of Activation Functions for Memory Footprint Reduction
arxiv(2022)
摘要
Memory footprint is one of the main limiting factors for large neural network training. In backpropagation, one needs to store the input to each operation in the computational graph. Every modern neural network model has quite a few pointwise nonlinearities in its architecture, and such operation induces additional memory costs which -- as we show -- can be significantly reduced by quantization of the gradients. We propose a systematic approach to compute optimal quantization of the retained gradients of the pointwise nonlinear functions with only a few bits per each element. We show that such approximation can be achieved by computing optimal piecewise-constant approximation of the derivative of the activation function, which can be done by dynamic programming. The drop-in replacements are implemented for all popular nonlinearities and can be used in any existing pipeline. We confirm the memory reduction and the same convergence on several open benchmarks.
更多查看译文
关键词
memory
AI 理解论文
溯源树
样例
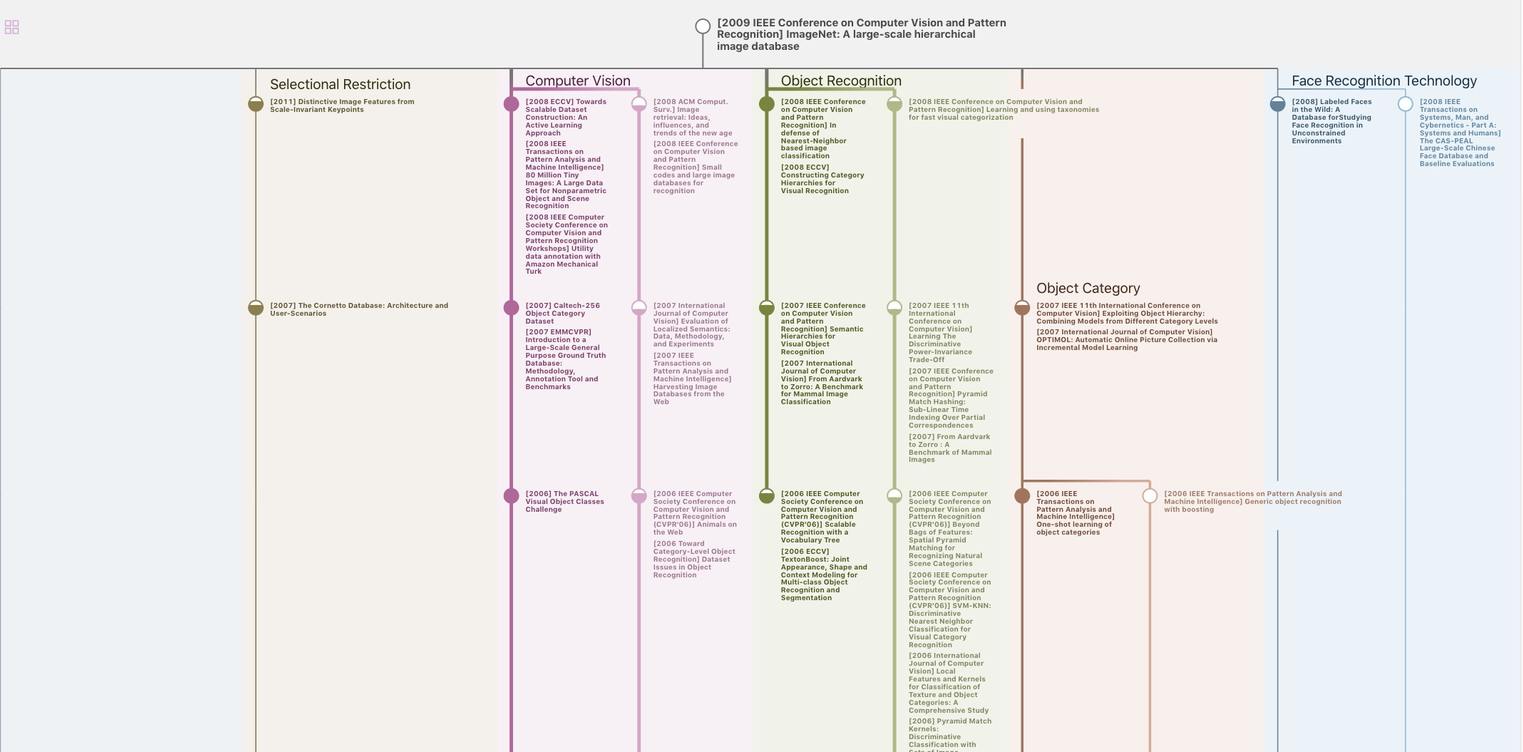
生成溯源树,研究论文发展脉络
Chat Paper
正在生成论文摘要