Adversarial Imitation Learning from Video Using a State Observer
IEEE International Conference on Robotics and Automation(2022)
摘要
The imitation learning research community has recently made significant progress towards the goal of enabling artificial agents to imitate behaviors from video demonstrations alone. However, current state-of-the-art approaches developed for this problem exhibit high sample complexity due, in part, to the high-dimensional nature of video observations. Towards addressing this issue, we introduce here a new algorithm called Visual Generative Adversarial Imitation from Observation using a State Observer (VGAIfO-SO). At its core, VGAIfO-SO seeks to address sample inefficiency using a novel, self-supervised state observer, which provides estimates of lower-dimensional proprioceptive state representations from high-dimensional images. We show experimentally in several continuous control environments that VGAIfO-SO is more sample efficient than other IfO algorithms at learning from video-only demonstrations and can sometimes even achieve performance close to the Generative Adversarial Imitation from Observation (GAIfO) algorithm that has privileged access to the demonstrator's proprioceptive state information.
更多查看译文
关键词
state observer,video,learning
AI 理解论文
溯源树
样例
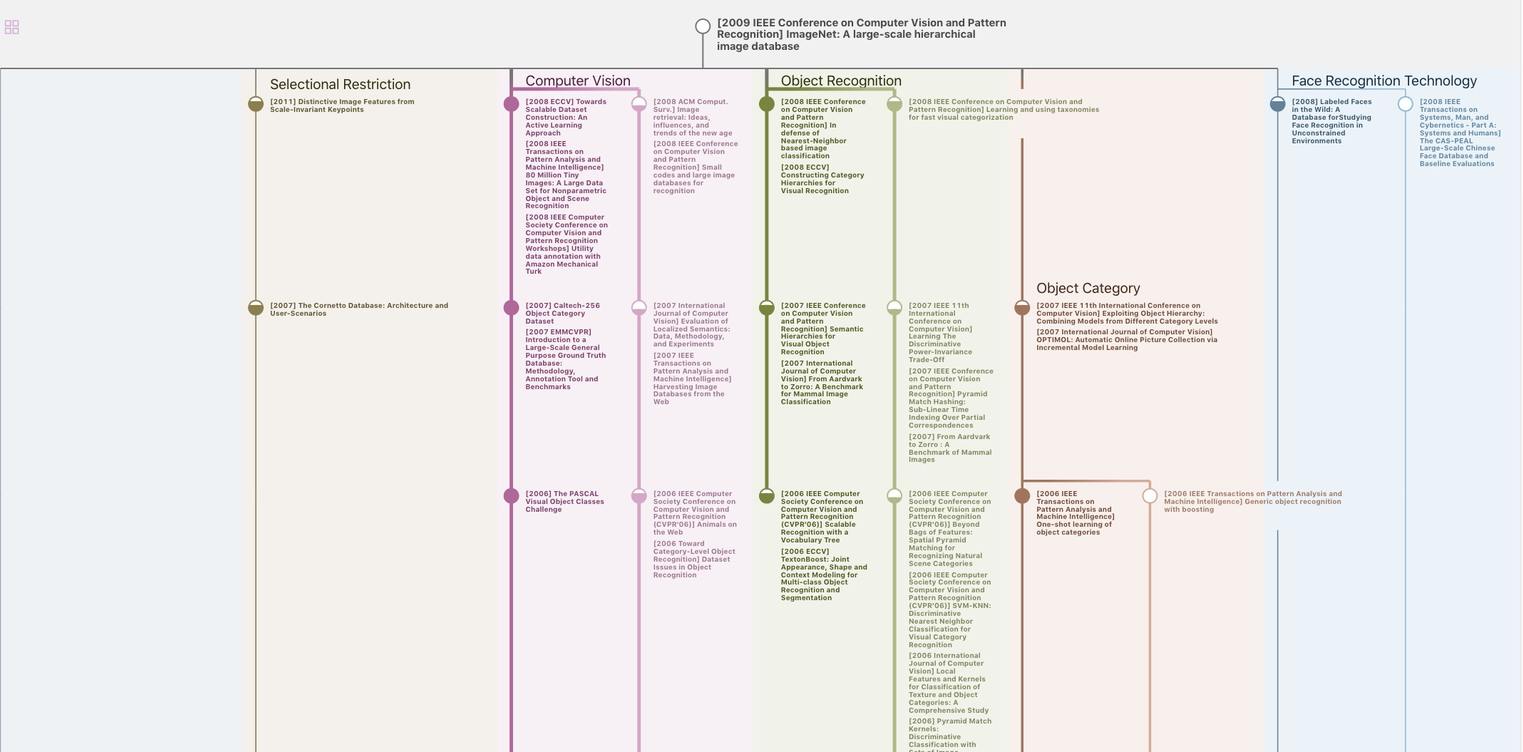
生成溯源树,研究论文发展脉络
Chat Paper
正在生成论文摘要