A preliminary study on early postmortem submersion interval (PMSI) estimation and cause-of-death discrimination based on nontargeted metabolomics and machine learning algorithms
International Journal of Legal Medicine(2022)
Abstract
Postmortem submersion interval (PMSI) estimation and cause-of-death discrimination of corpses in water have long been challenges in forensic practice. Recently, many studies have linked postmortem metabolic changes with PMI extension, providing a potential strategy for estimating PMSI using the metabolome. Additionally, there is a lack of potential indicators with high sensitivity and specificity for drowning identification. In the present study, we profiled the untargeted metabolome of blood samples from drowning and postmortem submersion rats at different PMSIs within 24 h by liquid chromatography–tandem mass spectrometry (LC–MS/MS). A total of 601 metabolites were detected. Four different machine learning algorithms, including random forest (RF), partial least squares (PLS), support vector machine (SVM), and neural network (NN), were used to compare the efficiency of the machine learning methods. Nineteen metabolites with obvious temporal regularity were selected as candidate biomarkers according to “IncNodePurity.” Robust models were built with these biomarkers, which yielded a mean absolute error of 1.067 h. Additionally, 36 other metabolites were identified to build the classifier model for discriminating drowning and postmortem submersion (AUC = 1, accuracy = 95%). Our results demonstrated the potential application of metabolomics combined with machine learning in PMSI estimation and cause-of-death discrimination.
MoreTranslated text
Key words
Forensic medicine, Metabolomics, Drowning, Postmortem submersion interval, Machine learning
AI Read Science
Must-Reading Tree
Example
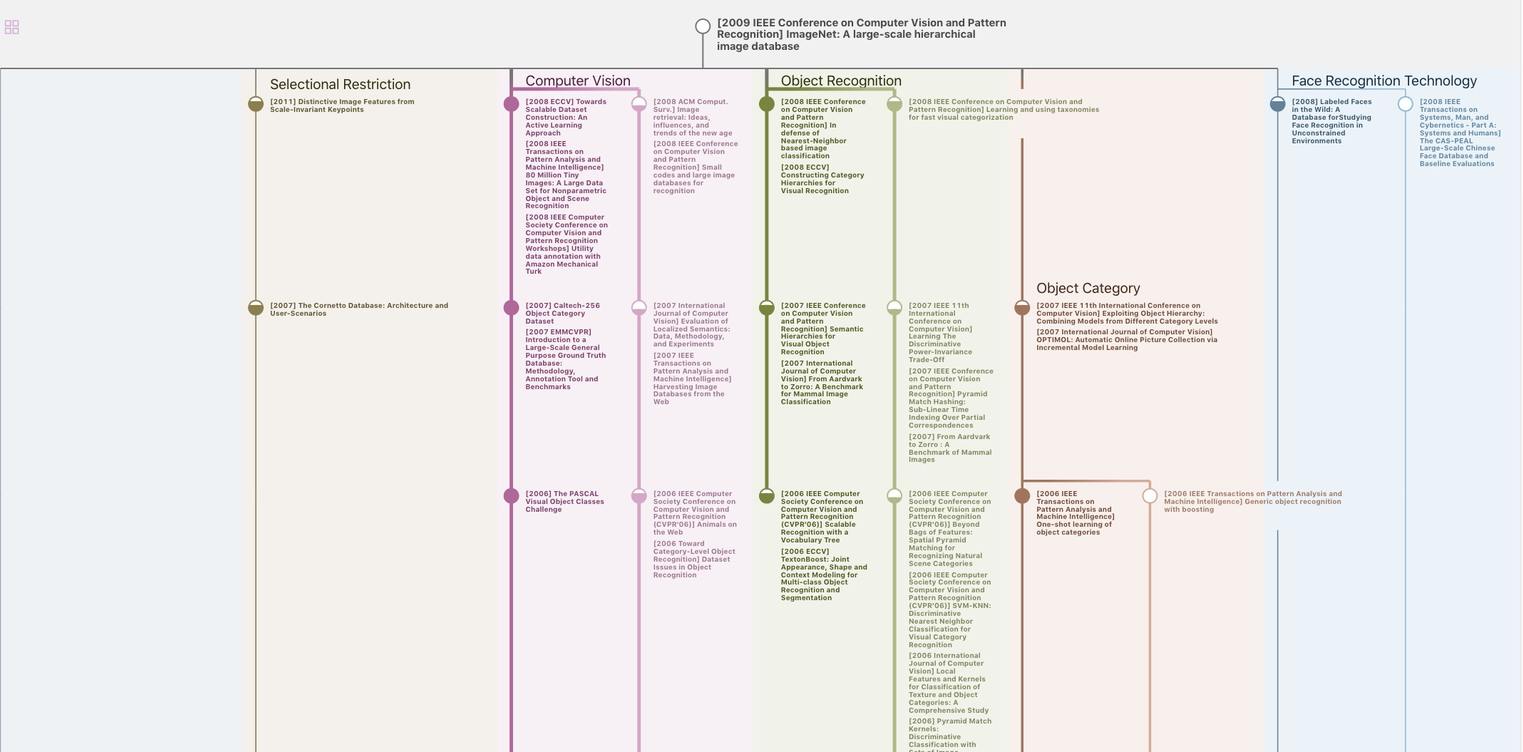
Generate MRT to find the research sequence of this paper
Chat Paper
Summary is being generated by the instructions you defined