Ligand-Directed GPCR Antibody Discovery.
Methods in molecular biology (Clifton, N.J.)(2022)
摘要
Developing affinity reagents recognizing and modulating G-protein coupled receptors (GPCR) function by traditional animal immunization or in vitro screening methods is challenging. Some anti-GPCR antibodies exist on the market, but the success rate of development is still poor compared with antibodies targeting soluble or peripherally anchored proteins. More importantly, most of these antibodies do not modulate GPCR function. The current pipeline for antibody development primarily screens for overall affinity rather than functional epitope recognition. We developed a new strategy utilizing natural ligand affinity to generate a library of antibody variants with an inherent bias toward the active site of the GPCR. Instead of using phage libraries displaying antibodies with random CDR sequences at polymorphism sites observed in natural immune repertoire sequences, we generated focused antibody libraries with a natural ligand encoded within or conjugated to one of the CDRs or the N-terminus. To tailor antibody binding to the active site, we limited the sequence randomization of the antibody in regions holstering the ligand while leaving the ligand-carrying part unaltered in the first round of randomization. With hits from the successful first round, the second round of randomization of the ligand-carrying part was then performed to eliminate the bias of the ligand. Based on our results on three different GPCR targets, the proposed pipeline will enable the rapid generation of functional antibodies (both agonists and antagonists) against high-value targets with poor function epitope exposures including GPCR, channels, transporters as well as cell surface targets whose binding site is heavily masked by glycosylation.
更多查看译文
关键词
Agonist,Antagonist,G-protein coupled receptors,GPCR,Monoclonal antibody,Phage display
AI 理解论文
溯源树
样例
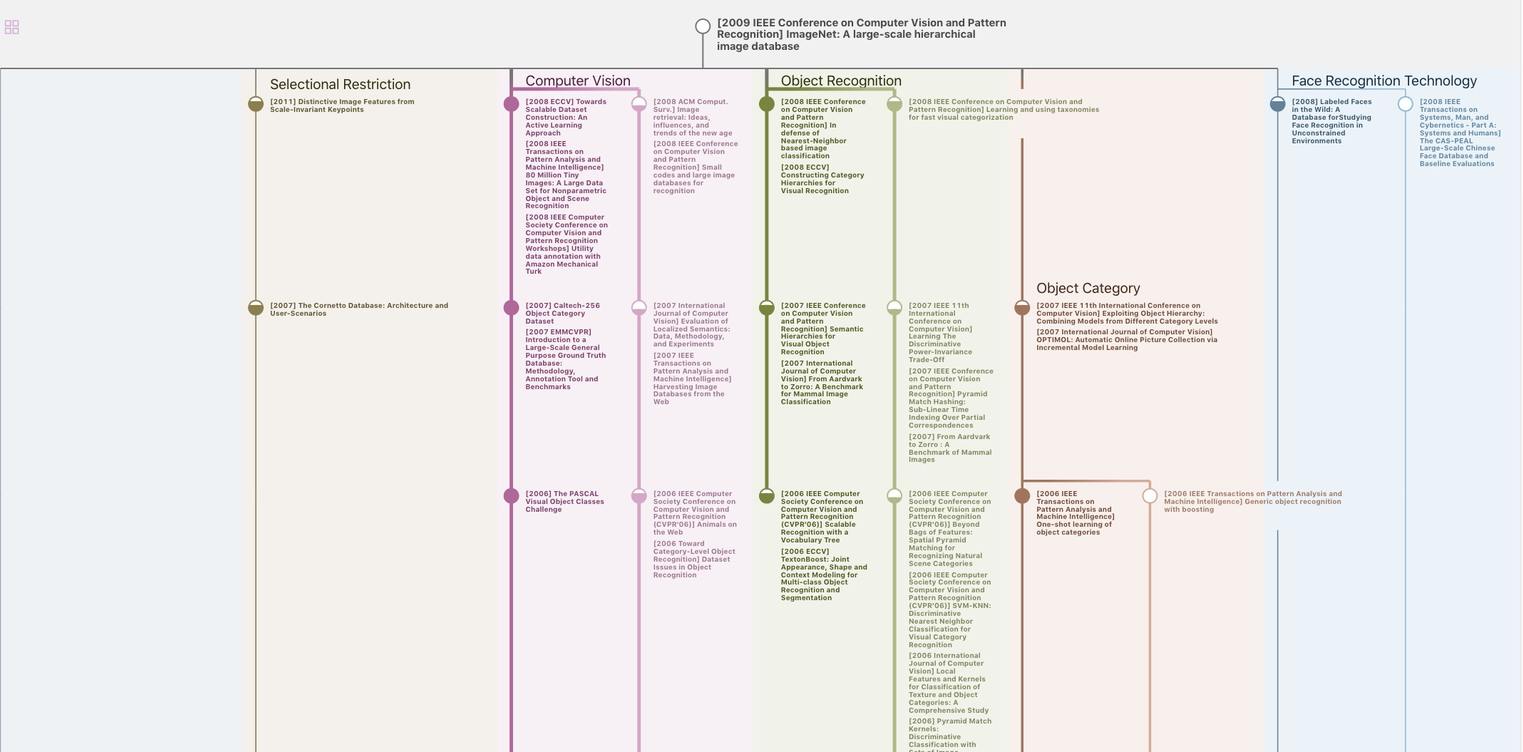
生成溯源树,研究论文发展脉络
Chat Paper
正在生成论文摘要