Automated Pipeline for Infants Continuous EEG (APICE): A flexible pipeline for developmental cognitive studies
DEVELOPMENTAL COGNITIVE NEUROSCIENCE(2022)
摘要
Infant electroencephalography (EEG) presents several challenges compared with adult data: recordings are typically short and heavily contaminated by motion artifacts, and the signal changes throughout development. Traditional data preprocessing pipelines, developed mainly for event-related potential analyses, require manual steps. However, larger datasets make this strategy infeasible. Moreover, new analytical approaches may have different preprocessing requirements. We propose an Automated Pipeline for Infants Continuous EEG (APICE). APICE is fully automated, flexible, and modular. The use of multiple algorithms and adaptive thresholds for artifact detection makes it suitable across age groups and testing procedures. Furthermore, the preprocessing is performed on continuous data, enabling better data recovery and flexibility (i.e., the same preprocessing is usable for different analyzes). Here we describe APICE and validate its performance in terms of data quality and data recovery using two very different infant datasets. Specifically, (1) we show how APICE performs when varying its artifacts rejection sensitivity; (2) we test the effect of different data cleaning methods such as the correction of transient artifacts, Independent Component Analysis, and Denoising Source Separation; and (3) we compare APICE with other available pipelines. APICE uses EEGLAB and compatible custom functions. It is freely available at https://github.com/neurokidslab/eeg_preprocessing, together with example scripts.
更多查看译文
关键词
Preprocessing, EEG, MEG, ERP, Infant, Development
AI 理解论文
溯源树
样例
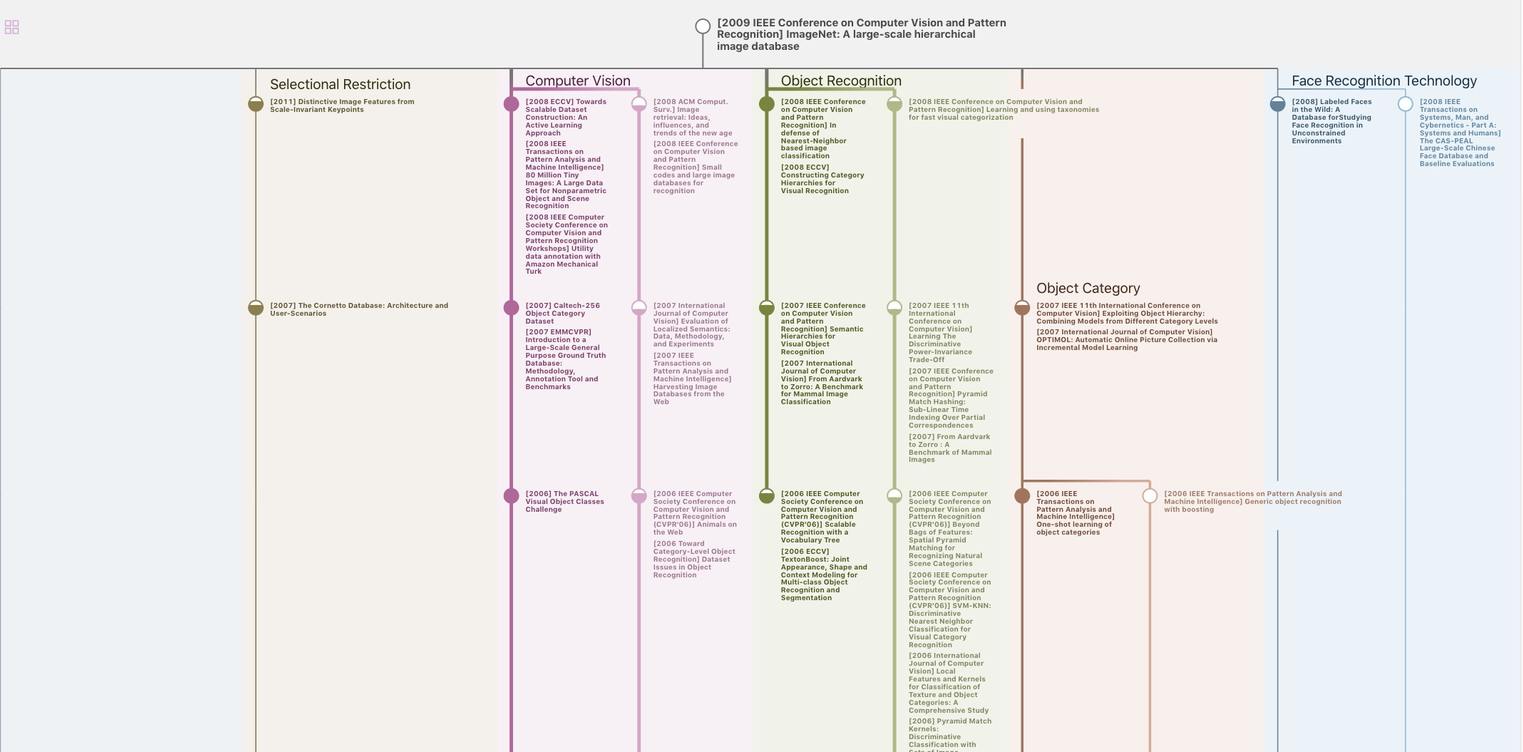
生成溯源树,研究论文发展脉络
Chat Paper
正在生成论文摘要