L-SVRG and L-Katyusha with Adaptive Sampling
arxiv(2023)
摘要
Stochastic gradient-based optimization methods, such as L-SVRG and its accelerated variant L-Katyusha (Kovalev et al., 2020), are widely used to train machine learning models.The theoretical and empirical performance of L-SVRG and L-Katyusha can be improved by sampling observations from a non-uniform distribution (Qian et al., 2021). However,designing a desired sampling distribution requires prior knowledge of smoothness constants, which can be computationally intractable to obtain in practice when the dimension of the model parameter is high. To address this issue, we propose an adaptive sampling strategy for L-SVRG and L-Katyusha that can learn the sampling distribution with little computational overhead, while allowing it to change with iterates, and at the same time does not require any prior knowledge of the problem parameters. We prove convergence guarantees for L-SVRG and L-Katyusha for convex objectives when the sampling distribution changes with iterates. Our results show that even without prior information, the proposed adaptive sampling strategy matches, and in some cases even surpasses, the performance of the sampling scheme in Qian et al. (2021). Extensive simulations support our theory and the practical utility of the proposed sampling scheme on real data.
更多查看译文
关键词
adaptive,l-svrg,l-katyusha
AI 理解论文
溯源树
样例
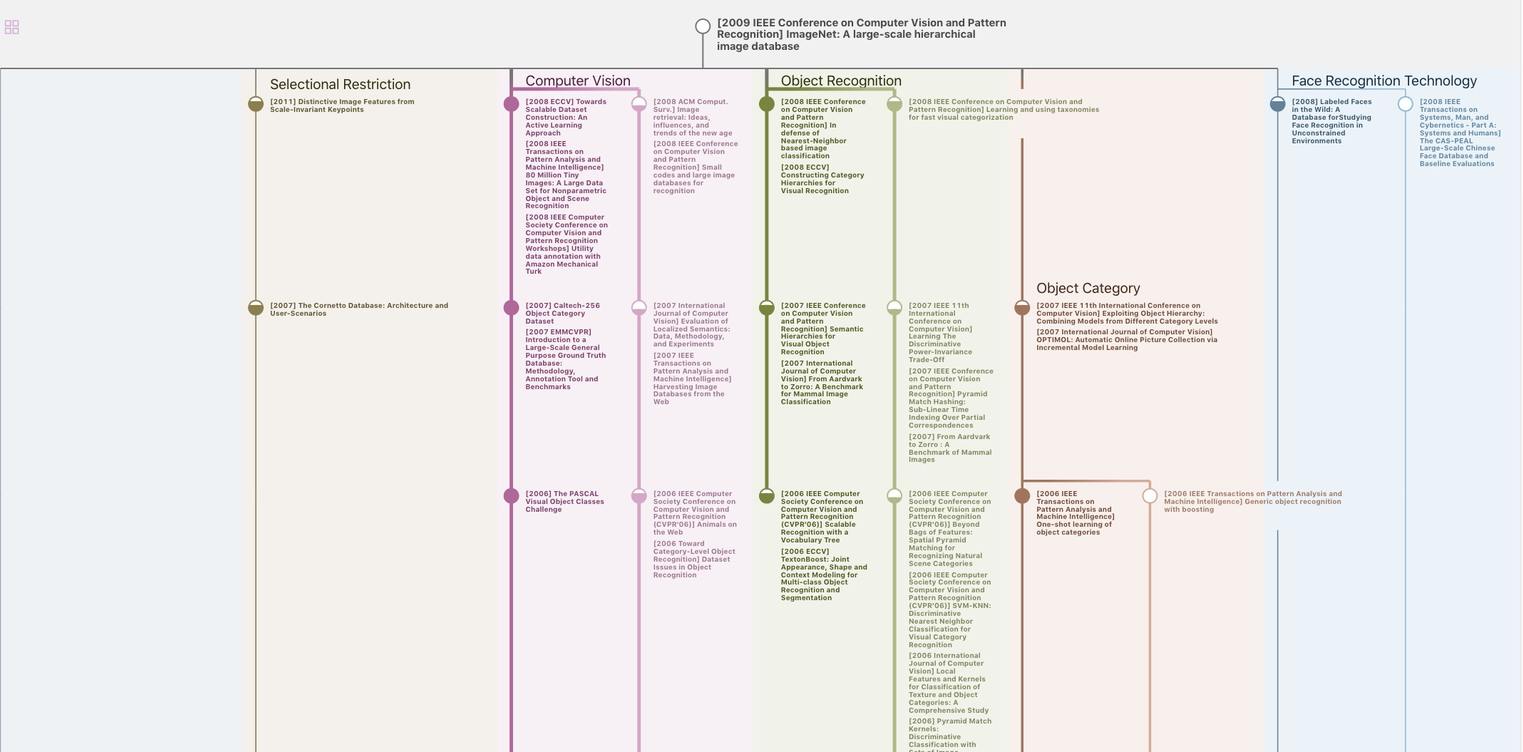
生成溯源树,研究论文发展脉络
Chat Paper
正在生成论文摘要