Neural Network Training With Asymmetric Crosspoint Elements.
Frontiers in Artificial Intelligence(2022)
摘要
Analog crossbar arrays comprising programmable non-volatile resistors are under intense investigation for acceleration of deep neural network training. However, the ubiquitous asymmetric conductance modulation of practical resistive devices critically degrades the classification performance of networks trained with conventional algorithms. Here we first describe the fundamental reasons behind this incompatibility. Then, we explain the theoretical underpinnings of a novel fully-parallel training algorithm that is compatible with asymmetric crosspoint elements. By establishing a powerful analogy with classical mechanics, we explain how device asymmetry can be exploited as a useful feature for analog deep learning processors. Instead of conventionally tuning weights in the direction of the error function gradient, network parameters can be programmed to successfully minimize the total energy (Hamiltonian) of the system that incorporates the effects of device asymmetry. Our technique enables immediate realization of analog deep learning accelerators based on readily available device technologies.
更多查看译文
关键词
DNN training,analog computing,hardware accelerator architecture,learning algorithm,neuromorphic accelerator
AI 理解论文
溯源树
样例
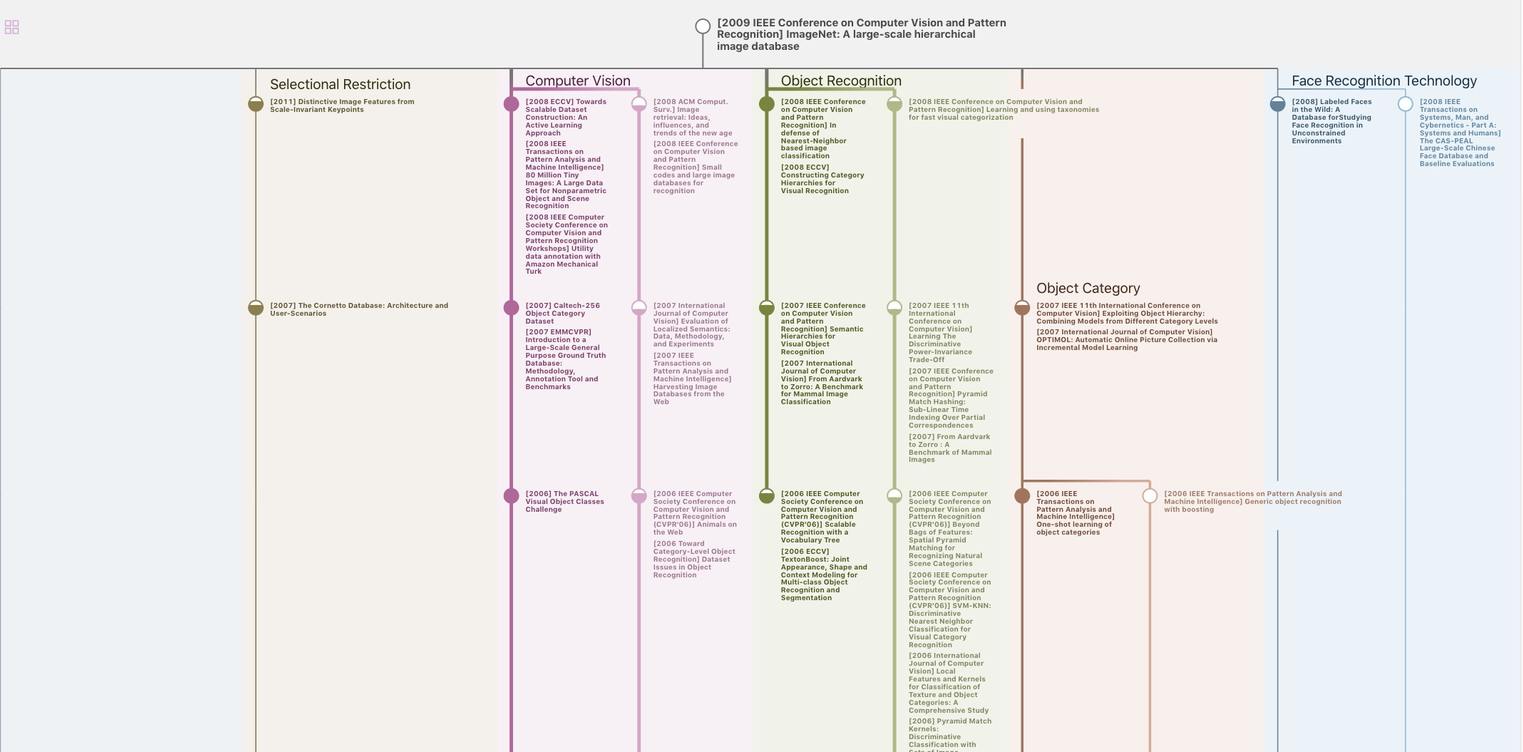
生成溯源树,研究论文发展脉络
Chat Paper
正在生成论文摘要