Lymphoma segmentation from 3D PET-CT images using a deep evidential network
International Journal of Approximate Reasoning(2022)
摘要
An automatic evidential segmentation method based on Dempster-Shafer theory and deep learning is proposed to segment lymphomas from three-dimensional Positron Emission Tomography (PET) and Computed Tomography (CT) images. The architecture is composed of a deep feature-extraction module and an evidential layer. The feature extraction module uses an encoder-decoder framework to extract semantic feature vectors from 3D inputs. The evidential layer then uses prototypes in the feature space to compute a belief function at each voxel quantifying the uncertainty about the presence or absence of a lymphoma at this location. Two evidential layers are compared, based on different ways of using distances to prototypes for computing mass functions. The whole model is trained end-to-end by minimizing the Dice loss function. The proposed combination of deep feature extraction and evidential segmentation is shown to outperform the baseline UNet model as well as three other state-of-the-art models on a dataset of 173 patients.
更多查看译文
关键词
Medical image analysis,Dempster-Shafer theory,Evidence theory,Belief functions,Uncertainty quantification,Deep learning
AI 理解论文
溯源树
样例
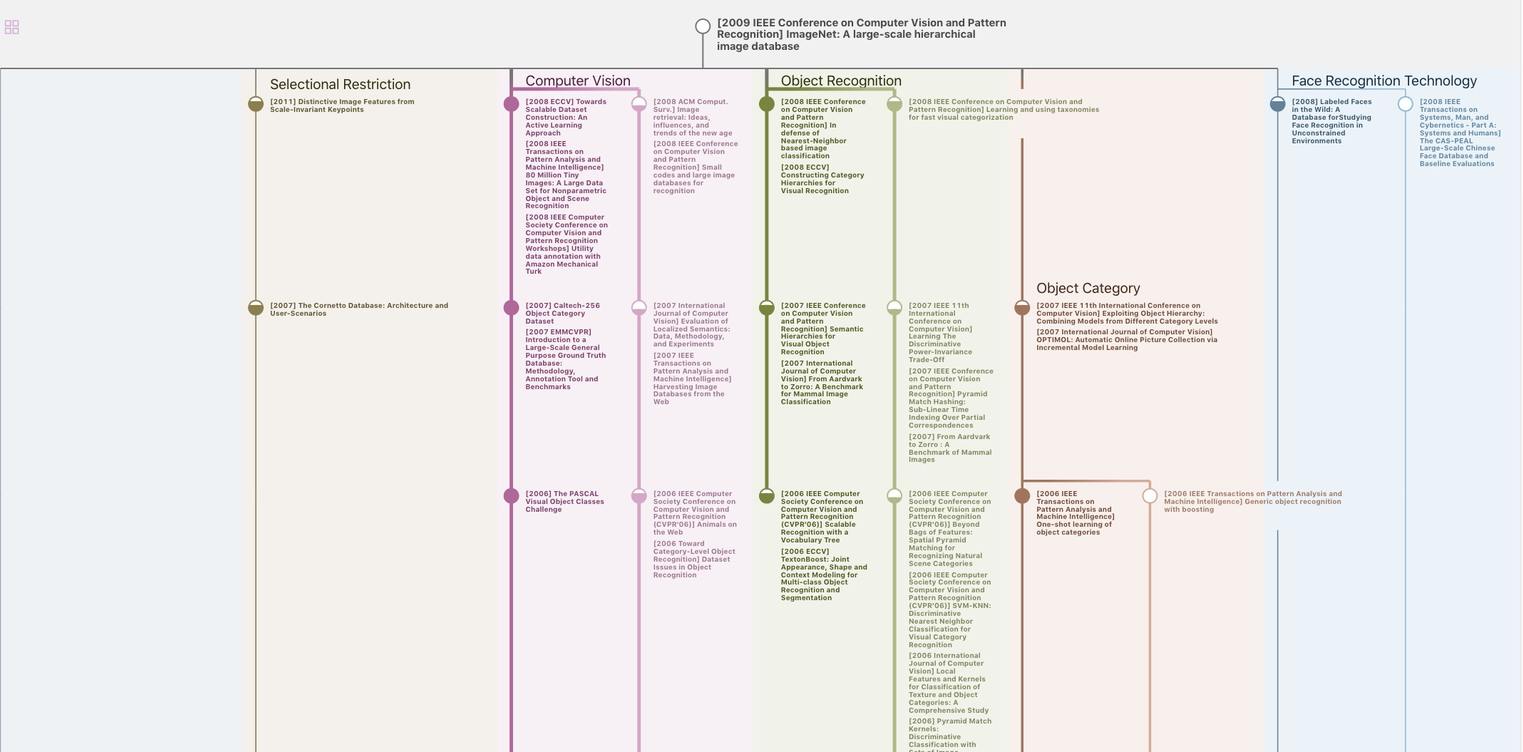
生成溯源树,研究论文发展脉络
Chat Paper
正在生成论文摘要