Fair Wrapping for Black-box Predictions
NeurIPS 2022(2022)
摘要
We introduce a new family of techniques to post-process ("wrap") a black-box classifier in order to reduce its bias. Our technique builds on the recent analysis of improper loss functions whose optimization can correct any twist in prediction, unfairness being treated as a twist. In the post-processing, we learn a wrapper function which we define as an $\alpha$-tree, which modifies the prediction. We provide two generic boosting algorithms to learn $\alpha$-trees. We show that our modification has appealing properties in terms of composition of $\alpha$-trees, generalization, interpretability, and KL divergence between modified and original predictions. We exemplify the use of our technique in three fairness notions: conditional value-at-risk, equality of opportunity, and statistical parity; and provide experiments on several readily available datasets.
更多查看译文
关键词
Fairness,post-processing,loss functions,boosting
AI 理解论文
溯源树
样例
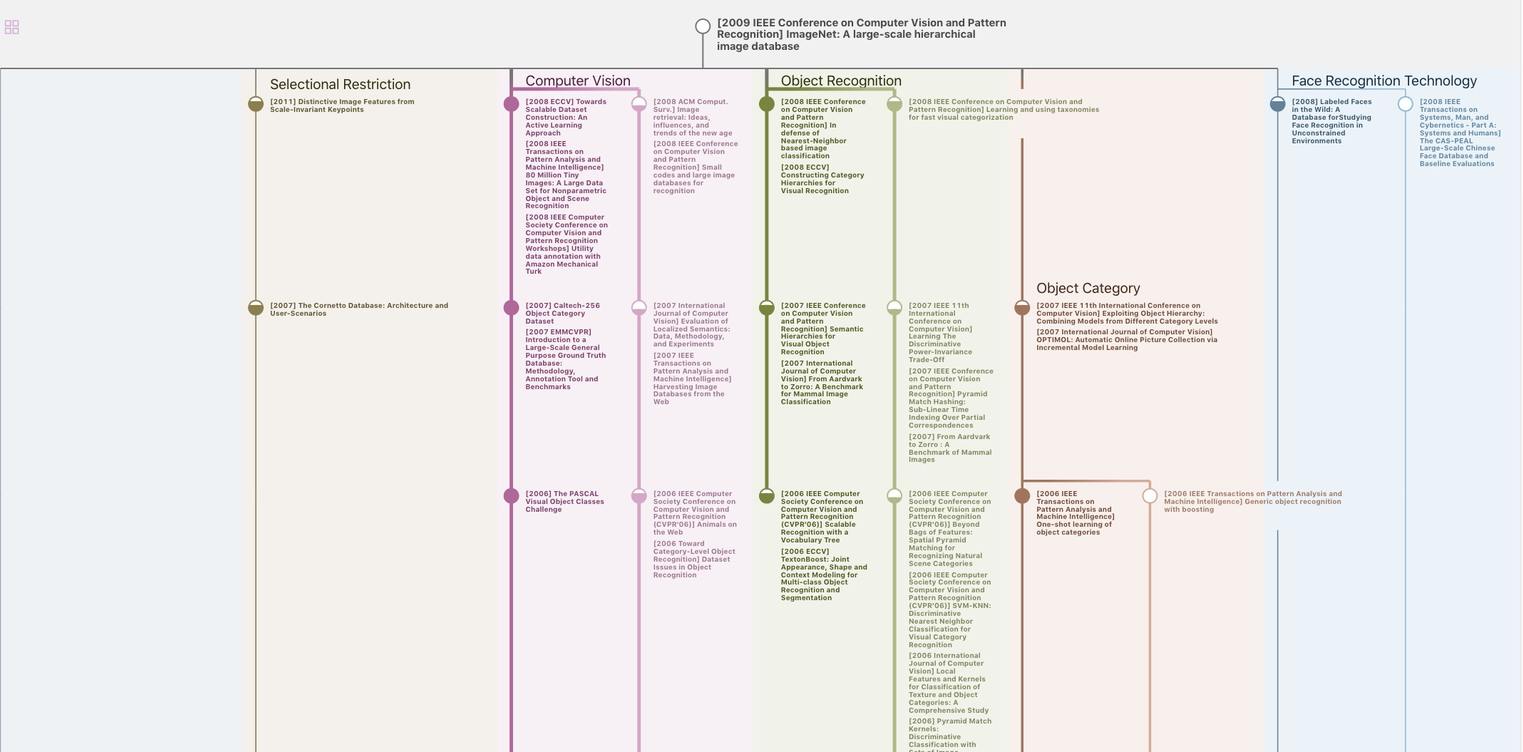
生成溯源树,研究论文发展脉络
Chat Paper
正在生成论文摘要