Bayesian optimization for mixed-variable, multi-objective problems
arxiv(2022)
摘要
Optimizing multiple, non-preferential objectives for mixed variable, expensive black-box problems is important in many areas of engineering and science. The expensive, noisy, black-box nature of these problems makes them ideal candidates for Bayesian optimization (BO). mixed variable and multi-objective problems, however, are a challenge due to BO’s underlying smooth Gaussian process surrogate model. Current multi-objective BO algorithms cannot deal with mixed variable problems. We present MixMOBO, the first mixed variable, multi-objective Bayesian optimization framework for such problems. Using MixMOBO, optimal Pareto-fronts for multi-objective, mixed variable design spaces can be found efficiently while ensuring diverse solutions. The method is sufficiently flexible to incorporate different kernels and acquisition functions, including those that were developed for mixed variable or multi-objective problems by other authors. We also present HedgeMO, a modified Hedge strategy that uses a portfolio of acquisition functions for multi-objective problems. We present a new acquisition function, SMC. Our results show that MixMOBO performs well against other mixed variable algorithms on synthetic problems. We apply MixMOBO to the real-world design of an architected material and show that our optimal design, which was experimentally fabricated and validated, has a normalized strain energy density 10^4 times greater than existing structures.
更多查看译文
关键词
Bayesian optimization,Mixed variables,Multi-objective,MixMOBO,HedgeMO,Architected meta-materials
AI 理解论文
溯源树
样例
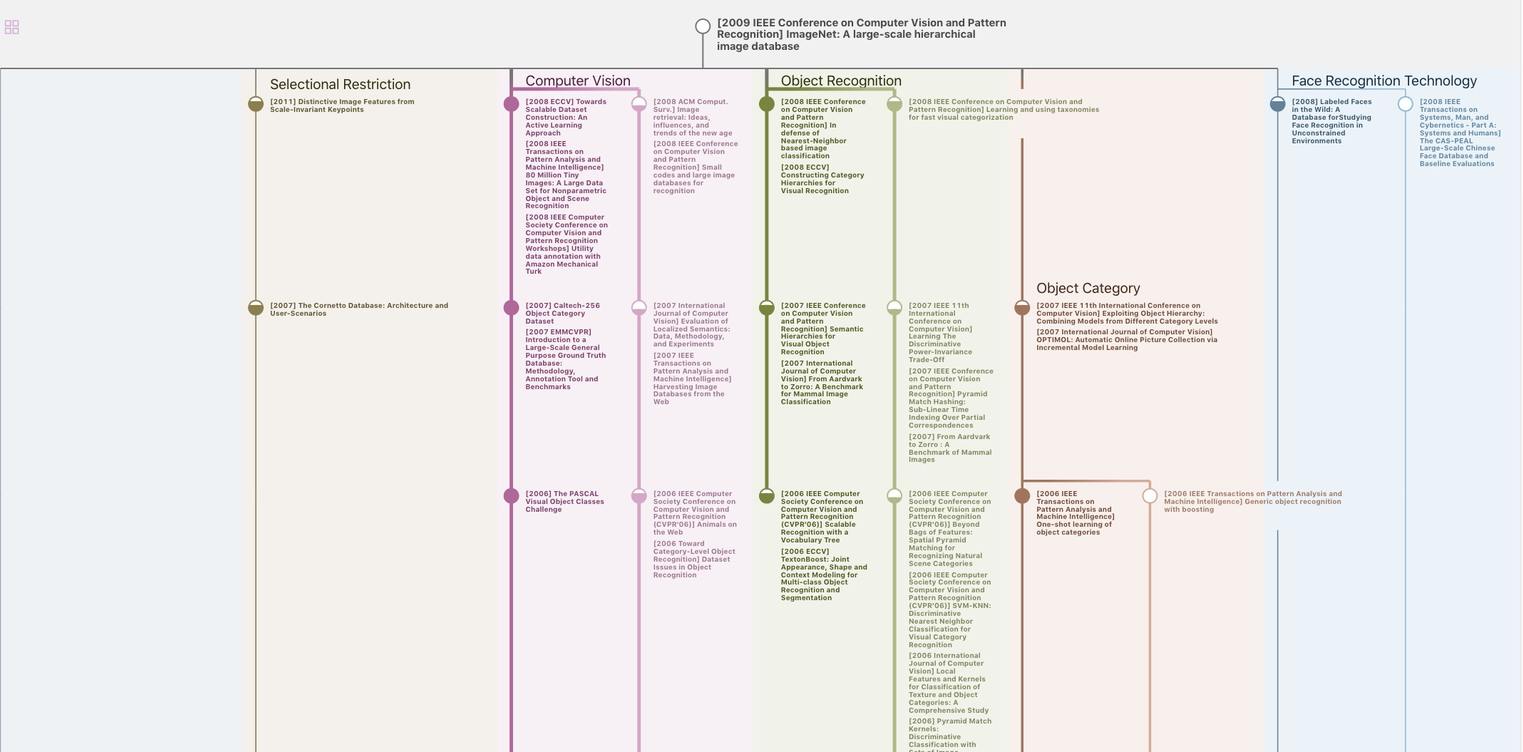
生成溯源树,研究论文发展脉络
Chat Paper
正在生成论文摘要