Physics-informed neural networks to learn cardiac fiber orientation from multiple electroanatomical maps
Eng. Comput.(2022)
摘要
We propose FiberNet, a method to estimate in-vivo the cardiac fiber architecture of the human atria from multiple catheter recordings of the electrical activation. Cardiac fibers play a central role in the electro-mechanical function of the heart, yet they are difficult to determine in-vivo, and hence rarely truly patient-specific in existing cardiac models. FiberNet learns the fiber arrangement by solving an inverse problem with physics-informed neural networks. The inverse problem amounts to identifying the conduction velocity tensor of a cardiac propagation model from a set of sparse activation maps. The use of multiple maps enables the simultaneous identification of all the components of the conduction velocity tensor, including the local fiber angle. We extensively test FiberNet on synthetic 2-D and 3-D examples, diffusion tensor fibers, and a patient-specific case. We show that 3 maps are sufficient to accurately capture the fibers, also in the presence of noise. With fewer maps, the role of regularization becomes prominent. Moreover, we show that the fitted model can robustly reproduce unseen activation maps. We envision that FiberNet will help the creation of patient-specific models for personalized medicine. The full code is available at http://github.com/fsahli/FiberNet .
更多查看译文
关键词
Cardiac fibers,Physics-informed neural networks,Cardiac electrophysiology,Anisotropic conduction velocity,Eikonal equation,Deep learning
AI 理解论文
溯源树
样例
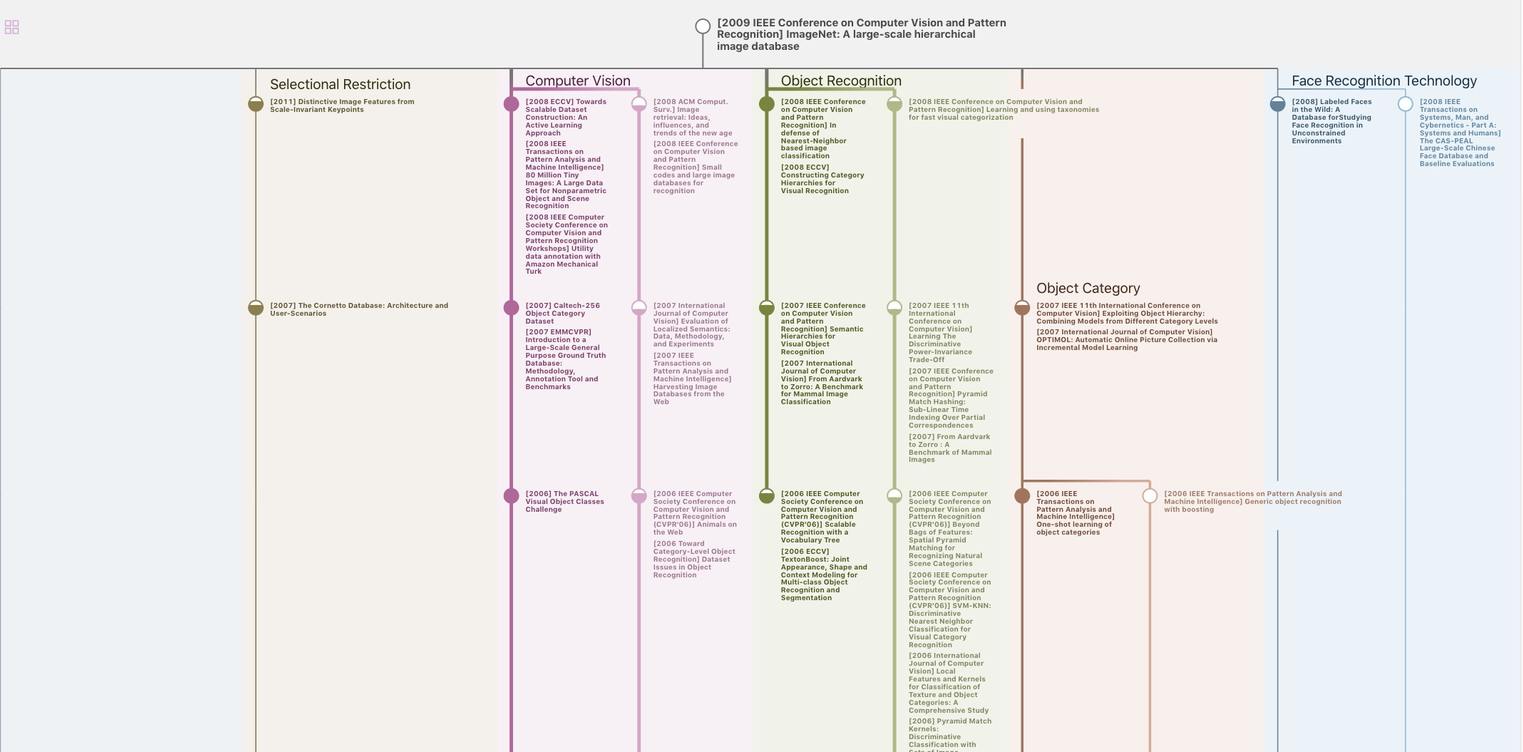
生成溯源树,研究论文发展脉络
Chat Paper
正在生成论文摘要