Adversarial Examples for Good: Adversarial Examples Guided Imbalanced Learning
2022 IEEE International Conference on Image Processing (ICIP)(2022)
摘要
Adversarial examples are inputs for machine learning models that have been designed by attackers to cause the model to make mistakes. In this paper, we demonstrate that adversarial examples can also be utilized for good to improve the performance of imbalanced learning. We provide a new perspective on how to deal with imbalanced data: adjust the biased decision boundary by training with Guiding Adversarial Examples (GAEs). Our method can effectively increase the accuracy of minority classes while sacrificing little accuracy on majority classes. We empirically show, on several benchmark datasets, our proposed method is comparable to the state-of-the-art method. To our best knowledge, we are the first to deal with imbalanced learning with adversarial examples.
更多查看译文
关键词
adversarial examples,long-tail data,imbalanced learning
AI 理解论文
溯源树
样例
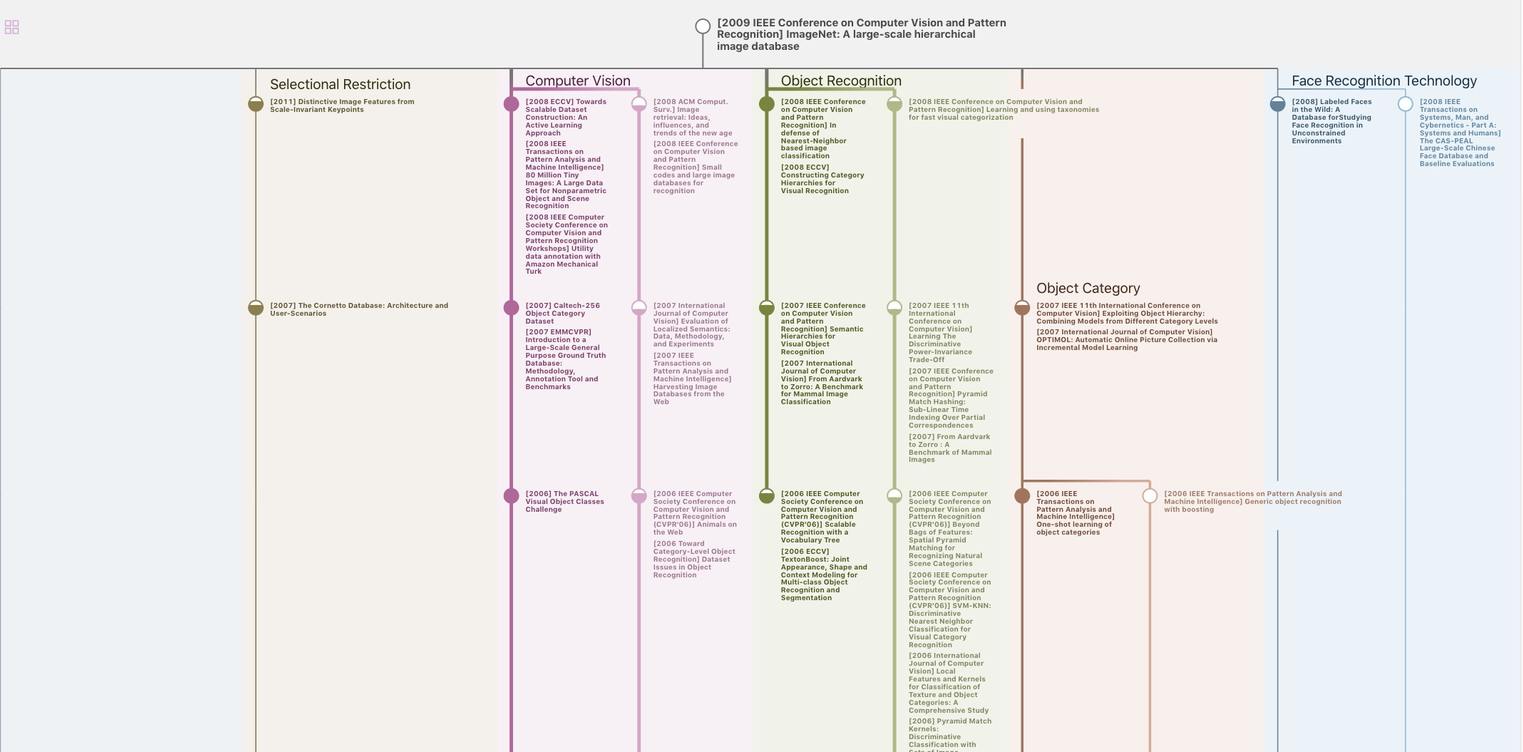
生成溯源树,研究论文发展脉络
Chat Paper
正在生成论文摘要