On the Effect of Data Dimensionality on Eigenvector Centrality
arxiv(2022)
摘要
Graphs (i.e., networks) have become an integral tool for the representation and analysis of relational data. Advances in data gathering have lead to multi-relational data sets which exhibit greater depth and scope. In certain cases, this data can be modeled using a hypergraph. However, in practice analysts typically reduce the dimensionality of the data (whether consciously or otherwise) to accommodate a traditional graph model. In recent years spectral hypergraph theory has emerged to study the eigenpairs of the adjacency hypermatrix of a uniform hypergraph. We show how analyzing multi-relational data, via a hypermatrix associated to the aforementioned hypergraph, can lead to conclusions different from those when the data is projected down to its co-occurrence matrix. In particular, we provide an example of a uniform hypergraph where the most central vertex (\`a la eigencentrality) changes depending on the order of the associated matrix. To the best of our knowledge this is the first known hypergraph to exhibit this property.
更多查看译文
关键词
eigenvector centrality,data dimensionality
AI 理解论文
溯源树
样例
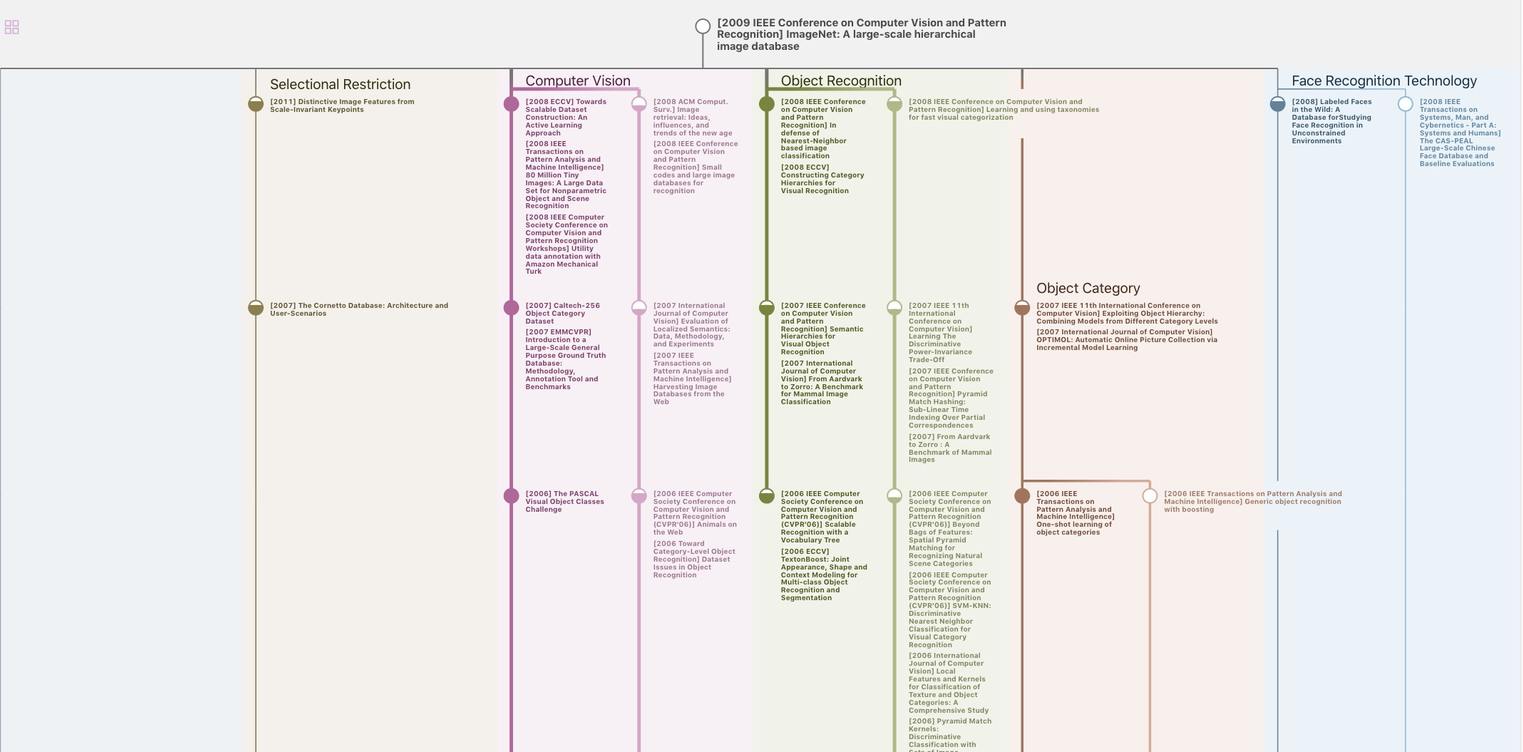
生成溯源树,研究论文发展脉络
Chat Paper
正在生成论文摘要