Efficient Distributed Learning in Stochastic Non-cooperative Games without Information Exchange
arxiv(2022)
摘要
In this work, we study stochastic non-cooperative games, where only noisy black-box function evaluations are available to estimate the cost function for each player. Since each player's cost function depends on both its own decision variables and its rivals' decision variables, local information needs to be exchanged through a center/network in most existing work for seeking the Nash equilibrium. We propose a new stochastic distributed learning algorithm that does not require communications among players. The proposed algorithm uses simultaneous perturbation method to estimate the gradient of each cost function, and uses mirror descent method to search for the Nash equilibrium. We provide asymptotic analysis for the bias and variance of gradient estimates, and show the proposed algorithm converges to the Nash equilibrium in mean square for the class of strictly monotone games at a rate faster than the existing algorithms. The effectiveness of the proposed method is buttressed in a numerical experiment.
更多查看译文
关键词
stochastic,learning,games
AI 理解论文
溯源树
样例
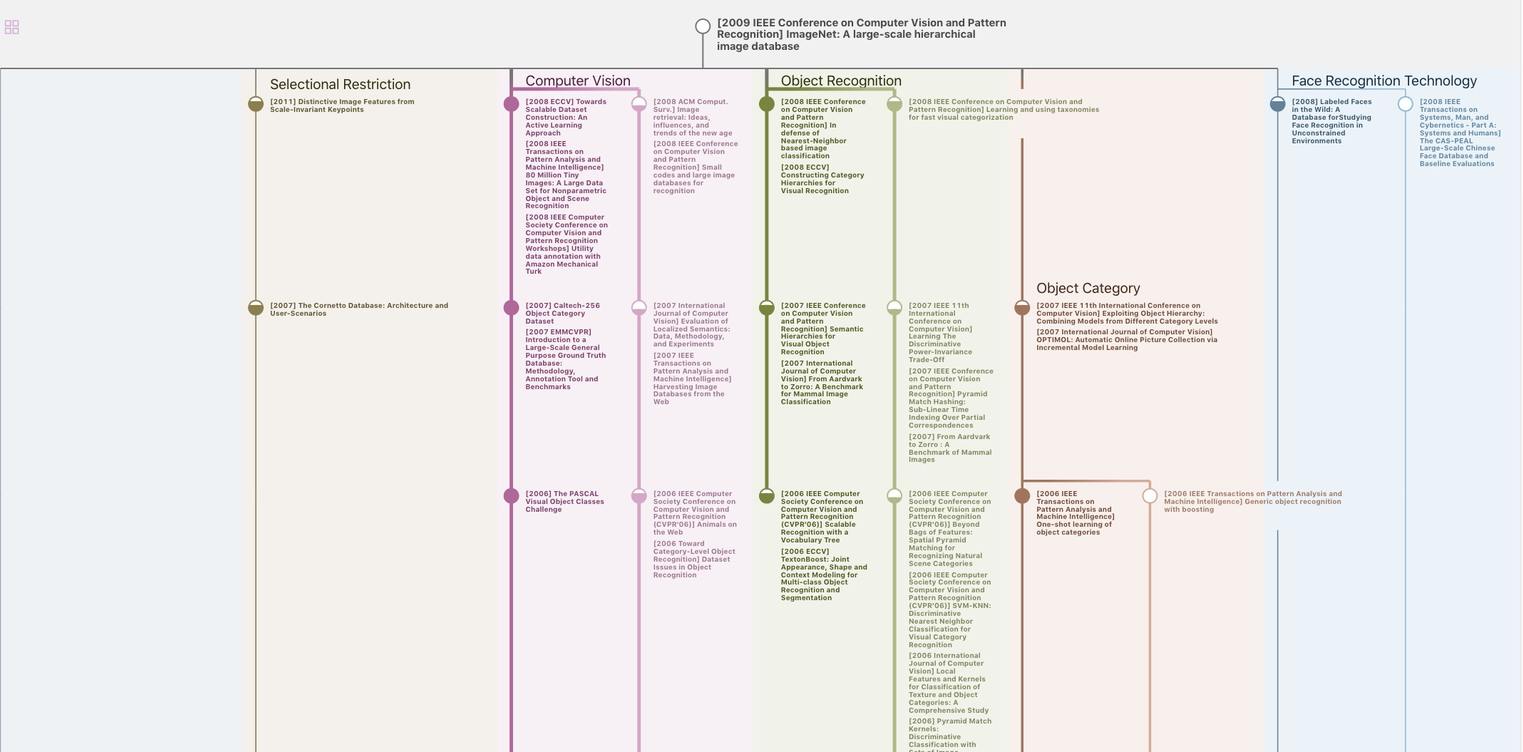
生成溯源树,研究论文发展脉络
Chat Paper
正在生成论文摘要