Auto-Compressing Subset Pruning for Semantic Image Segmentation
PATTERN RECOGNITION, DAGM GCPR 2022(2022)
摘要
State-of-the-art semantic segmentation models are characterized by high parameter counts and slow inference times, making them unsuitable for deployment in resource-constrained environments. To address this challenge, we propose AUTO-COMPRESSING SUBSET PRUNING, ACoSP, as a new online compression method. The core of ACoSP consists of learning a channel selection mechanism for individual channels of each convolution in the segmentation model based on an effective temperature annealing schedule. We show a crucial interplay between providing a high-capacity model at the beginning of training and the compression pressure forcing the model to compress concepts into retained channels. We apply ACoSP to SegNet and PSPNet architectures and show its success when trained on the CAMVID, CITYSCAPES, PASCAL VOC2012, and ADE2OK datasets. The results are competitive with existing baselines for compression of segmentation models at low compression ratios and outperform them significantly at high compression ratios, yielding acceptable results even when removing more than 93% of the parameters. In addition, ACoSP is conceptually simple, easy to implement, and can readily be generalized to other data modalities, tasks, and architectures. Our code is available at https://github.com/merantix/acosp.
更多查看译文
关键词
segmentation,auto-compressing
AI 理解论文
溯源树
样例
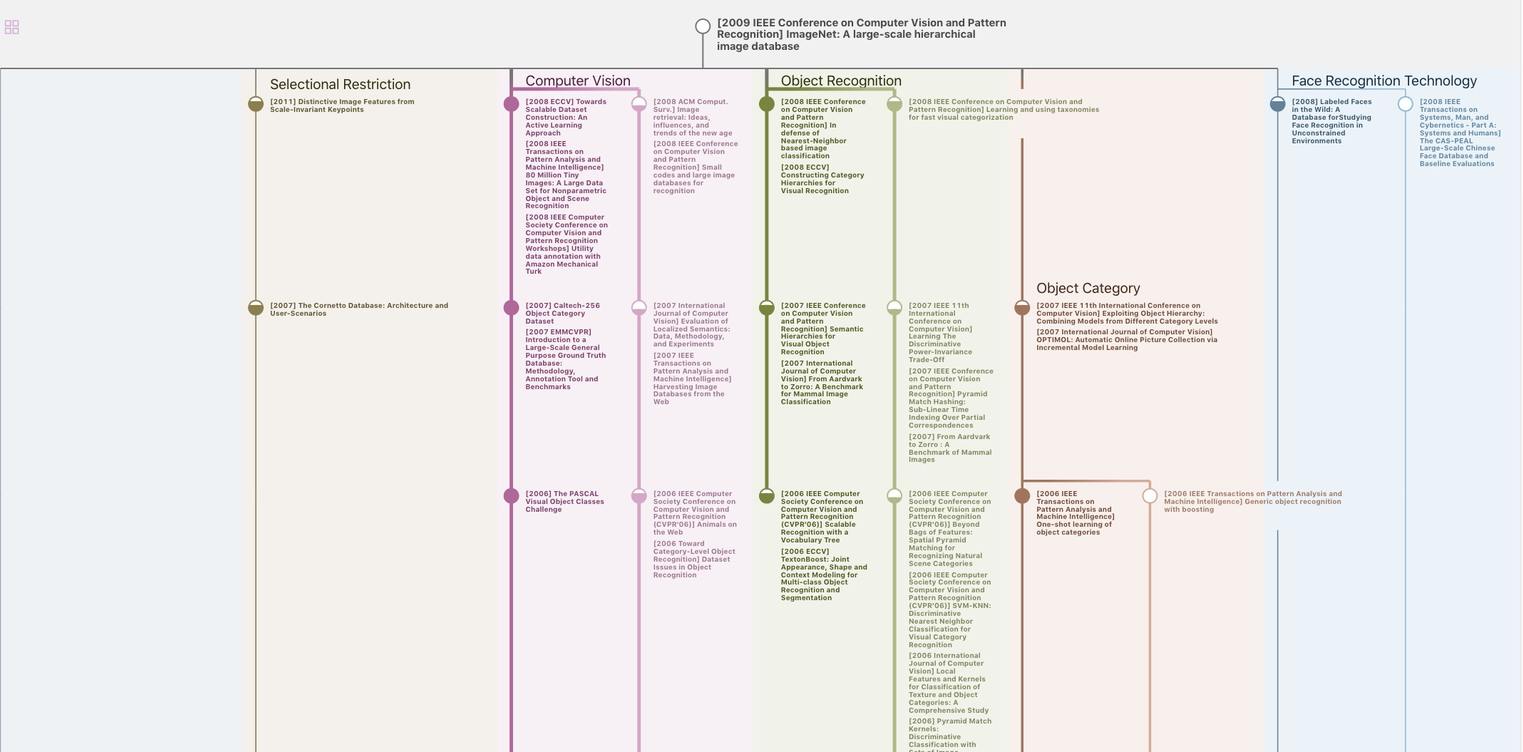
生成溯源树,研究论文发展脉络
Chat Paper
正在生成论文摘要