An Efficient and Robust System for Vertically Federated Random Forest
arxiv(2022)
摘要
As there is a growing interest in utilizing data across multiple resources to build better machine learning models, many vertically federated learning algorithms have been proposed to preserve the data privacy of the participating organizations. However, the efficiency of existing vertically federated learning algorithms remains to be a big problem, especially when applied to large-scale real-world datasets. In this paper, we present a fast, accurate, scalable and yet robust system for vertically federated random forest. With extensive optimization, we achieved $5\times$ and $83\times$ speed up over the SOTA SecureBoost model \cite{cheng2019secureboost} for training and serving tasks. Moreover, the proposed system can achieve similar accuracy but with favorable scalability and partition tolerance. Our code has been made public to facilitate the development of the community and the protection of user data privacy.
更多查看译文
关键词
forest,robust system
AI 理解论文
溯源树
样例
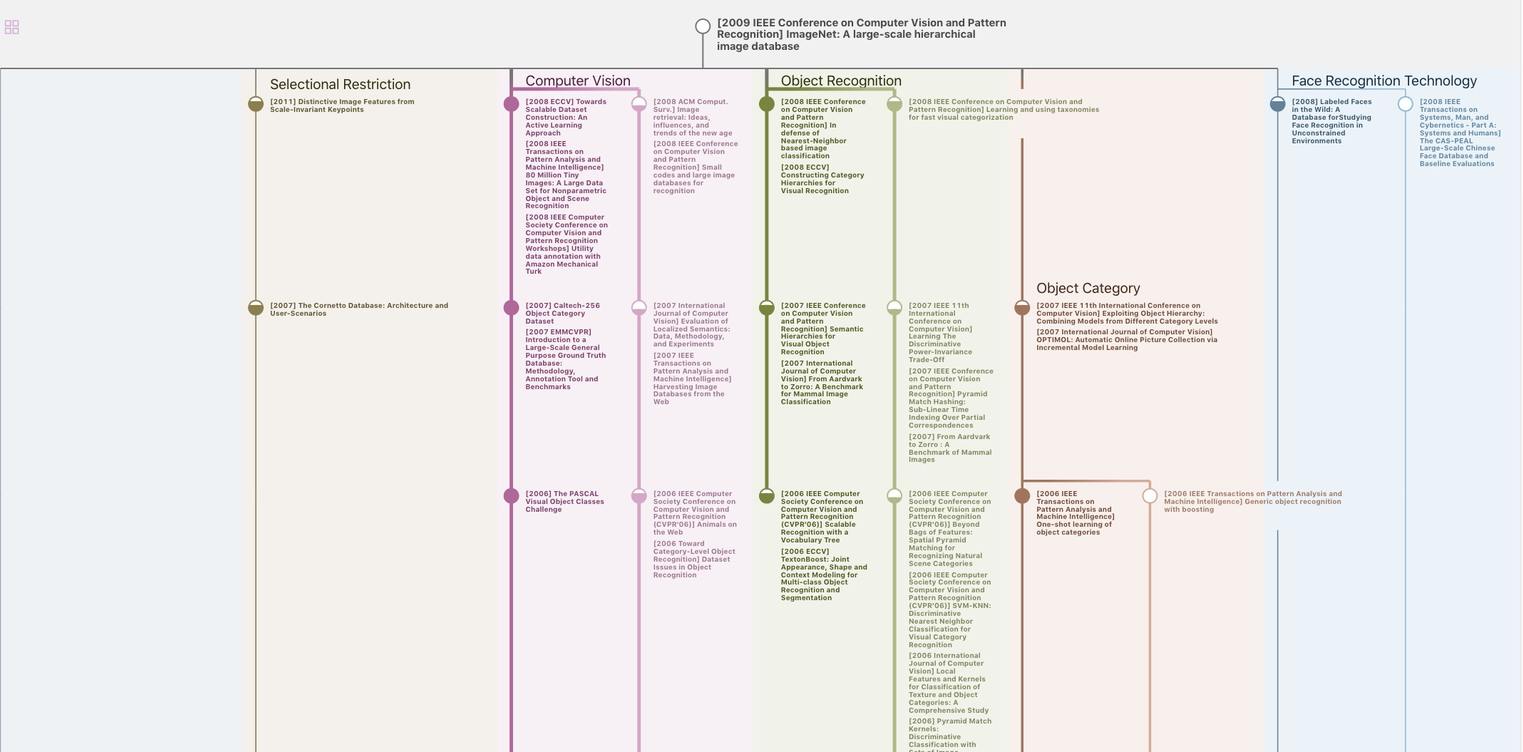
生成溯源树,研究论文发展脉络
Chat Paper
正在生成论文摘要