Multi-omics Cancer Prognosis Analysis Based on Graph Convolution Network.
BIBM(2021)
摘要
Cancer survival analysis is important for patients’ follow-up treatment, and accurate prediction is conductive to improving the survival rate of patients. With the development of gene sequencing technology, multi-omics data is increasingly utilized to predict cancer prognosis. However, it remains challenging to fuse different sources of omics data. To solve this problem, we introduced the interpretable constraint relationship between genes into the model and proposed a new method Graph Survival Network (GraphSurv) by combining the graph convolution network (GCN) with the deep Cox proportional hazard network. Compared with state-of-the-art methods, the average C-index value in 11 cancer datasets was increased by 4.0%. As a case study of liver hepatocellular carcinoma (LIHC), we downloaded GSE54236 and GSE14520 from the GEO database for independent tests. The results showed that our model could significantly distinguish high-risk from low-risk patients (C-index >0.6, log-rank p-value < 0.05). In addition, we performed differential expression analysis based on the divided risk subgroups by GraphSurv Among the top 15 identified differentially expressed genes (DEGs) ranked by log2 change value, 12 (80%) prognostic markers have been confirmed by literature review. The results proved that the method was robust and can identify genes related to the cancer prognosis.
更多查看译文
关键词
survival analysis,GCN,Cox proportional hazards,deep learning,multi-omics
AI 理解论文
溯源树
样例
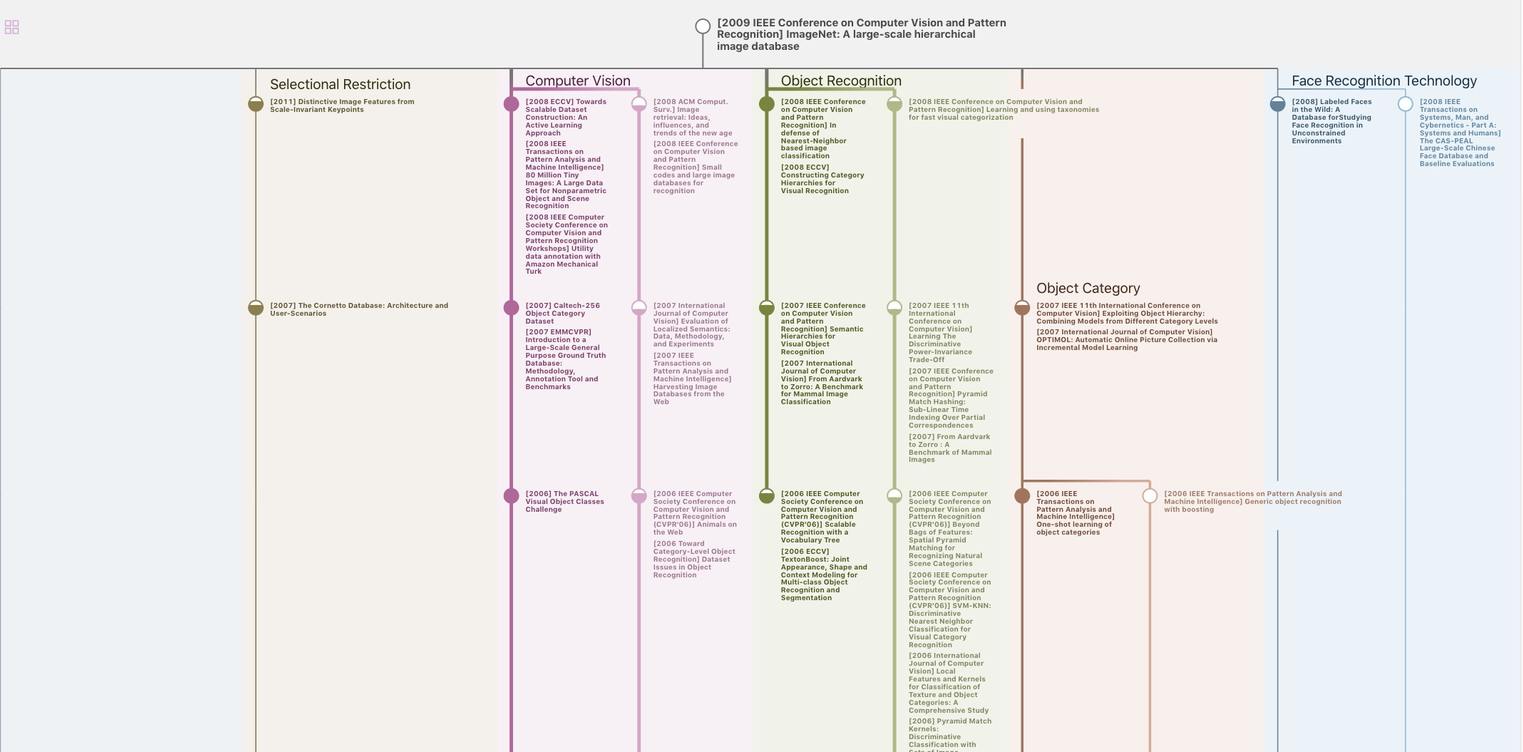
生成溯源树,研究论文发展脉络
Chat Paper
正在生成论文摘要