Feature selection using co-occurrence correlation improves cell clustering and embedding in single cell RNAseq data.
BIBM(2021)
摘要
Identifying the cell populations present in a single cell RNAseq (sc-RNAseq) dataset is made difficult by the high-dimensionality and sparse aspects of the data. Often the first step at resolving this challenge is to perform feature selection; selecting a set of informative genes in the dataset to use in cell embedding and clustering. The typical sc-RNAseq feature selection methods choose a subset of genes with largest variances in their detected expressions across single cells. Here we show these conventional feature selection methods are susceptible to inflated variances due to inconsistent transcriptomic sampling. As an alternative, we present a computational algorithm that uses the binary correlations (co-occurrences) between genes to perform feature selection. Using multiple sc-RNAseq datasets, we show this co-occurrence based feature selection approach outperforms popular high-variance feature selection methods in terms of cell clustering accuracy and separability. Taken together, these results suggest that the co-occurrence based method may be more appropriate for performing feature selection in sc-RNAseq data, and it can be easily implemented for most sc-RNAseq workflows. Additional details of the co-occurrence feature selection algoirthm and supplementary materials are available at https://github.com/ncsu-penglab/cooccur_feature_selection [1].
更多查看译文
关键词
single-cell,RNAseq,feature selection,PCA,UMAP,clustering
AI 理解论文
溯源树
样例
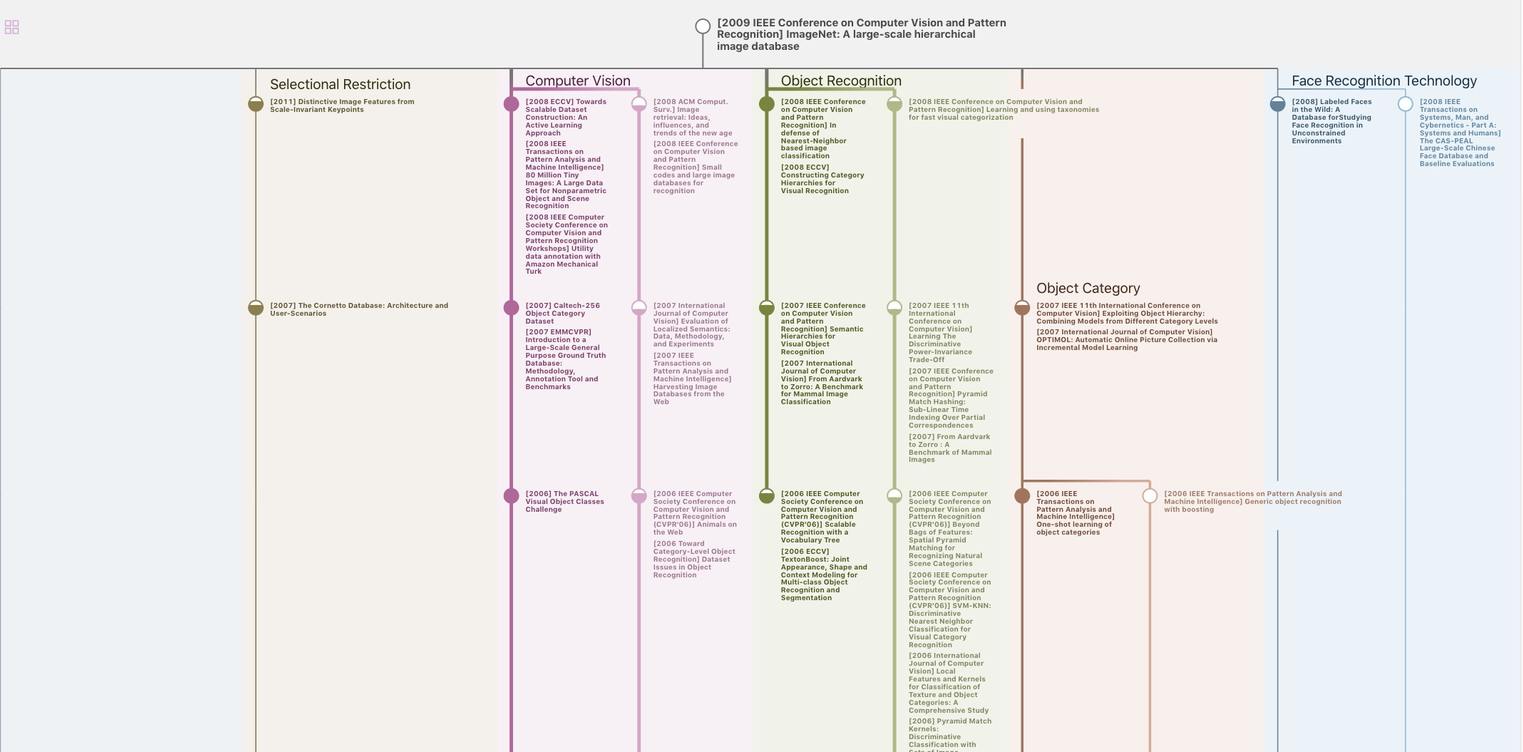
生成溯源树,研究论文发展脉络
Chat Paper
正在生成论文摘要