Predicting Multidrug Resistance Using Temporal Clinical Data and Machine Learning Methods.
BIBM(2021)
摘要
Infections caused by multidrug resistant (MR) bacteria severely jeopardize the public’s health given the inefficiency of current antibiotics to treat them. This results in a major global concern that affects any hospital service and the Intensive Care Unit (ICU) in particular. This paper aims to anticipate the antibiogram outcomes associated with MR bacteria in ICU patients by applying machine learning (ML) techniques. For this purpose, multiple clinical variables obtained from the Electronic Health Record have been employed, as the own patient’s antibiotics consumption and the drugs taken by the remaining ICU patients. A collection of 3476 patients admitted to the ICU at the University Hospital of Fuenlabrada from 2004 to 2020 were considered, 628 with MR bateria. A feature engineering (FE) and feature selection (FS) process has been conducted to extract valuable statistics from the original temporal data. The highest Accuracy and Specificity results achieved were 77% and 82%, respectively, both implementing Random Forest as classifier and without considering any FS method. The highest Sensitivity (69%) and ROC-AUC (76%) were attained with the features selected using the Chi-Square test and with both Logistic Regression and XGBoost classifiers. This work provides a promising approach to support therapy decisions by the early identification of MR infections among ICU patients.
更多查看译文
关键词
Predictive models,machine learning,temporal clinical data,drug treatment,multidrug resistance,ICU patients
AI 理解论文
溯源树
样例
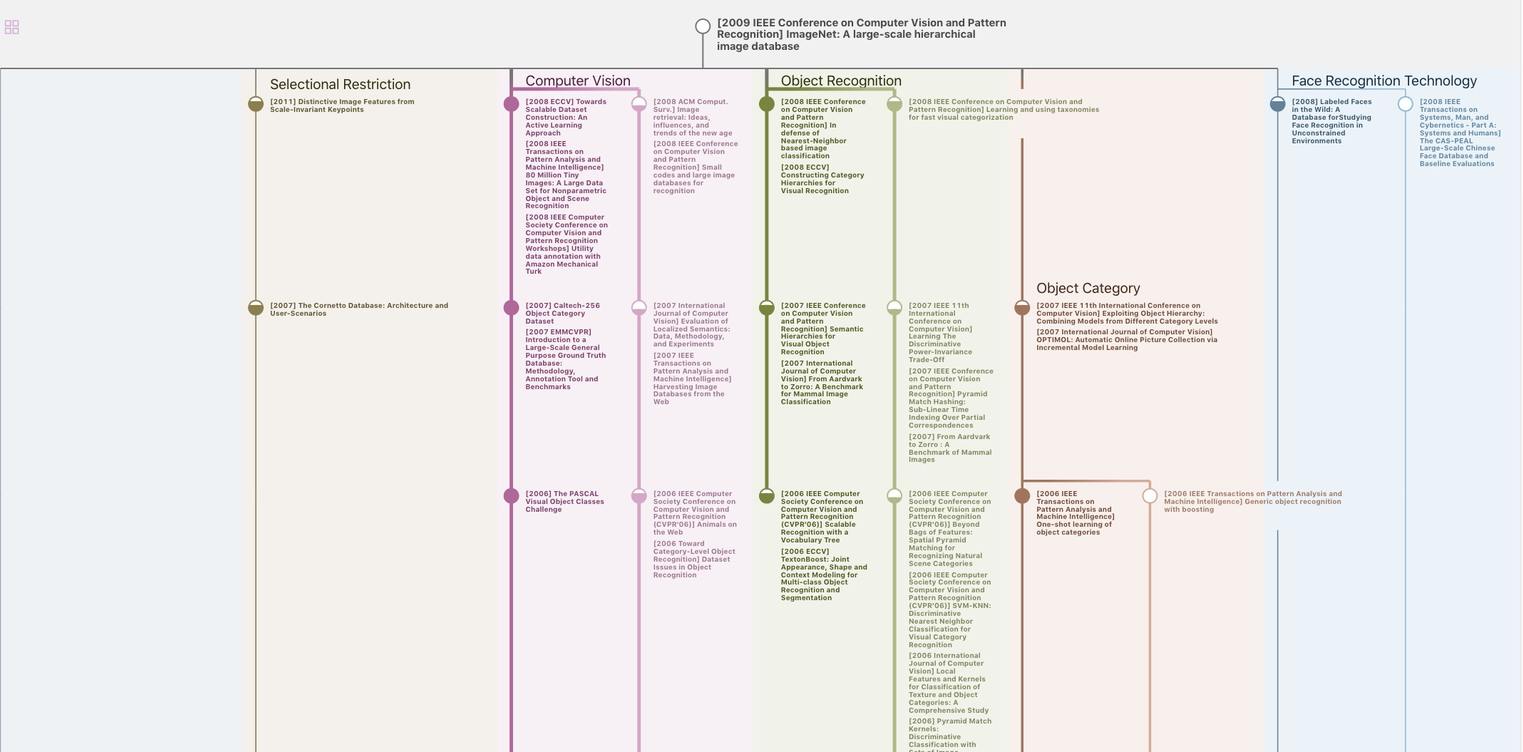
生成溯源树,研究论文发展脉络
Chat Paper
正在生成论文摘要