SGAT - a Self-supervised Graph Attention Network for Biomedical Relation Extraction.
BIBM(2021)
摘要
The goal of relation extraction task is to classify texts containing entity pairs into predefined relation types. Biomedical relation extraction can extract high-quality information from massive medical texts, which plays an important role in biomedical research. In this paper, we propose a self-supervised graph attention network to extract biomedical relations from the complex and noisy biomedical texts. The model incorporates self-supervision within the standard graph attention mechanism. Specifically, the model applies the graph attention mechanism to reduce the influence of noisy words and introduces dependency-based parse trees to construct a self-supervised task. With the supervision of dependency-based parse trees, the graph attention network can not only improve its capacity of learning syntactic information but also alleviate its lack of interpretability. Additionally, we use Gumbel Tree-GRU to obtain sentence information for relation classification. Our model achieves state-of-the-art performance on the DDIExtraction 2013 and ChemProt datasets, respectively, which suggests that our proposed model can effectively improve the performance of biomedical relation extraction.
更多查看译文
关键词
biomedical relation extraction,self-supervision,graph attention,dependency-based parse tree
AI 理解论文
溯源树
样例
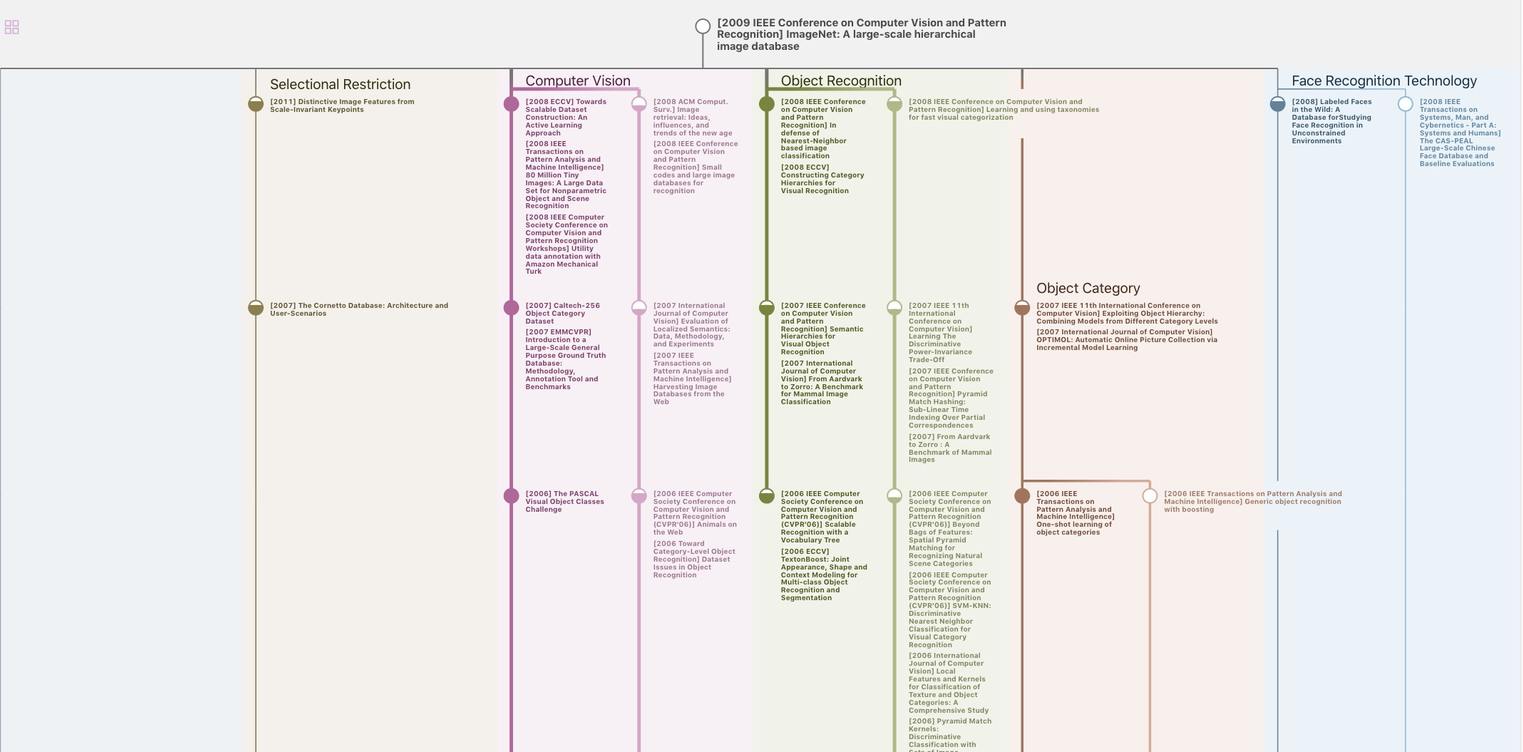
生成溯源树,研究论文发展脉络
Chat Paper
正在生成论文摘要