An Immune Inspired Algorithm for Fault Tolerant Enhanced Multimodal Machine Learning.
BIBM(2021)
摘要
Much like how sentient beings use multiple senses, Multimodal Machine Learning (MML) uses multiple input signals to form an estimation about the environment. An advantage MML has over standard Unimodal Learning is that if one input becomes corrupt or unavailable, a multimodal recognition model can rely on the other inputs by zeroing out the corrupt or missing one. As such, MML can become more robust and fault-tolerant. Unfortunately, it is not always known when an input has become corrupt or not. Corrupt inputs are anomalous in comparison to normal and expected data. We use an immune inspired algorithm, the Negative Selection Algorithm (NSA) as a resilient anomaly detection algorithm. Using the multimodal Smart Gym “MM-FIT” dataset, we evaluate how corrupt inputs affect the accuracy of MML classification. We implement the NSA specifically for a multimodal pipeline of activity recognition with anomaly detection. The results indicate that our recognition pipeline is effective in introducing fault-tolerance to MML.
更多查看译文
关键词
Multimodal Machine Learning,Negative Selection Algorithm,Fault Tolerance,Anomaly Detection,Human Activity Recognition
AI 理解论文
溯源树
样例
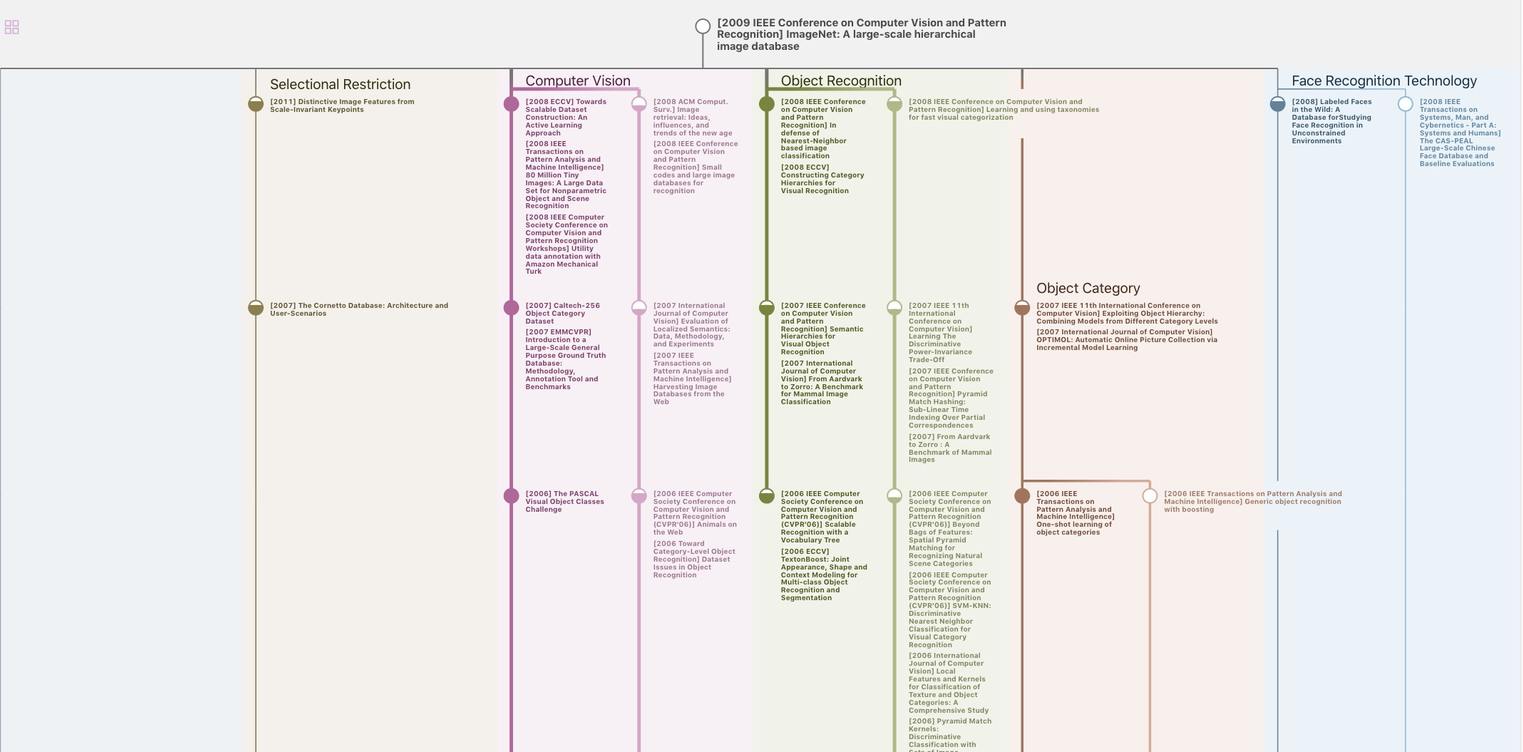
生成溯源树,研究论文发展脉络
Chat Paper
正在生成论文摘要