Impact of baseline drift removal on ECG beat classification and alignment.
CinC(2021)
摘要
Accurate beat classification and alignment is fundamental to any signal averaging method. The objective of this study was to investigate the accuracy of different beat classification and alignment methods, and the impact of pre-processing methods on these algorithms. Experimental data came from a human-shaped torso tank, with 256 body surface ECG recorded during sinus rhythm (SR) and left ventricular pacing (LVP) (n=4). "Gold-standard" classification and alignment were defined from recorded cardiac electrograms. Six different methods of baseline drift removal (BDR) were applied to ECG. Subsequently, 3 different beat segmentation methods were used to extract QRS complexes and align them, and four different beat classification methods. Pre-processing methods had only a small impact on beat classification and alignment compared to the segmentation and classification methods themselves. However, baseline drift removal over the whole QRS does appears to be important in providing the most accurate final averaged beat.
更多查看译文
关键词
baseline drift removal,ecg
AI 理解论文
溯源树
样例
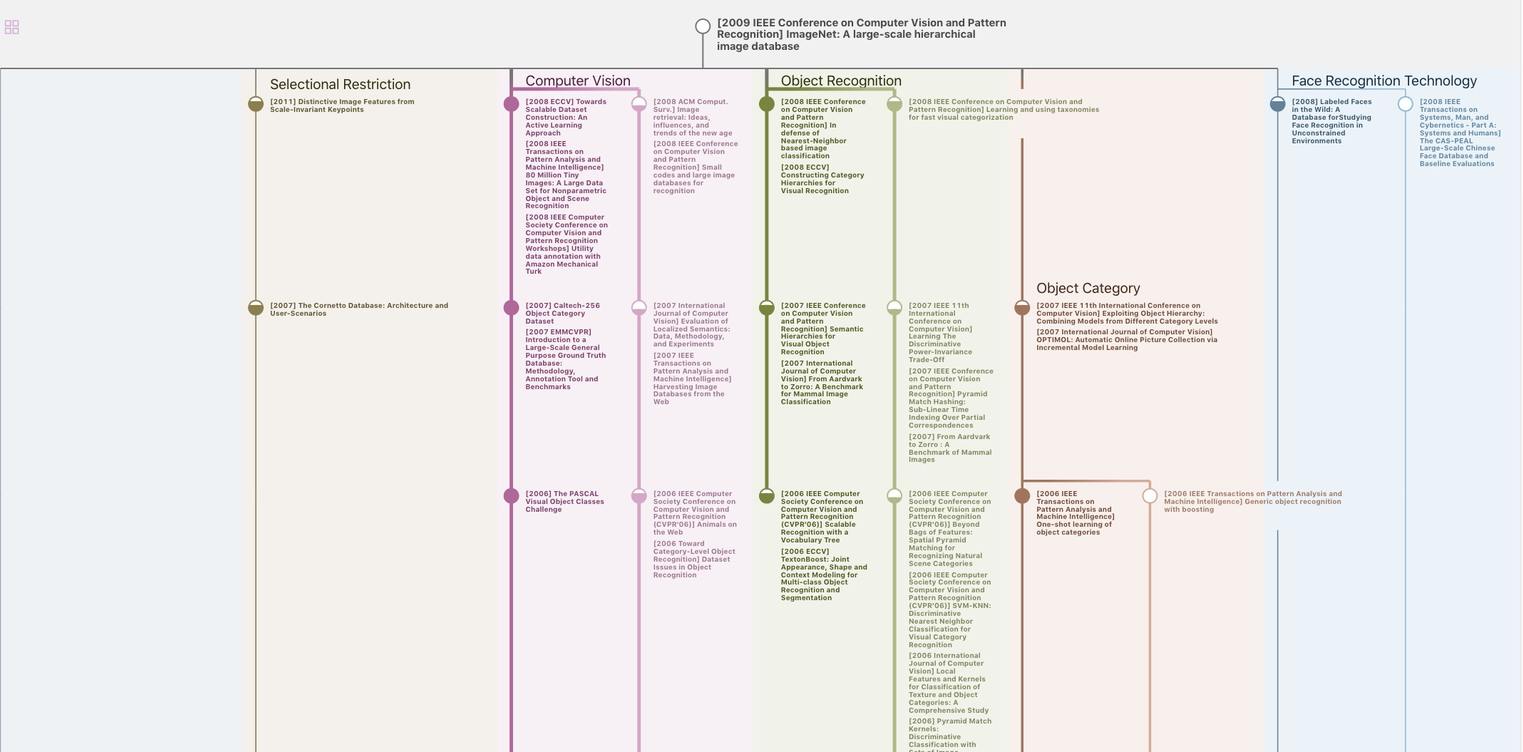
生成溯源树,研究论文发展脉络
Chat Paper
正在生成论文摘要