An Unsupervised Framework for Change Detection in Remote Sensing Images.
ICCT(2021)
Abstract
Generally, the supervised change detection methods with superior performance require a large amounts of labeled data. Considering the lack of labeled training data for change detection in remote sensing, in this paper, we propose a novel unsupervised framework for remote sensing image change detection. We train a convolutional neural network continuously with different pseudo labels. The first training can provide assistance for the second training and the performance of the network will be improved. In the two trainings, we design different loss functions to guide the optimization of the network parameters on different pseudo labels and the pixels with different confidence in the pseudo labels have different weights to inhibit the effect of the noise. The trainings are started by the pseudo labels generated by traditional change detection methods such as change vector analysis and no manually marked labels are used. Therefore, our approach is completely unsupervised. Experiments carried out on real remote sensing image datasets demonstrate the excellent performance of the proposed method.
MoreTranslated text
Key words
change detection,remote sensing,unsupervised framework
AI Read Science
Must-Reading Tree
Example
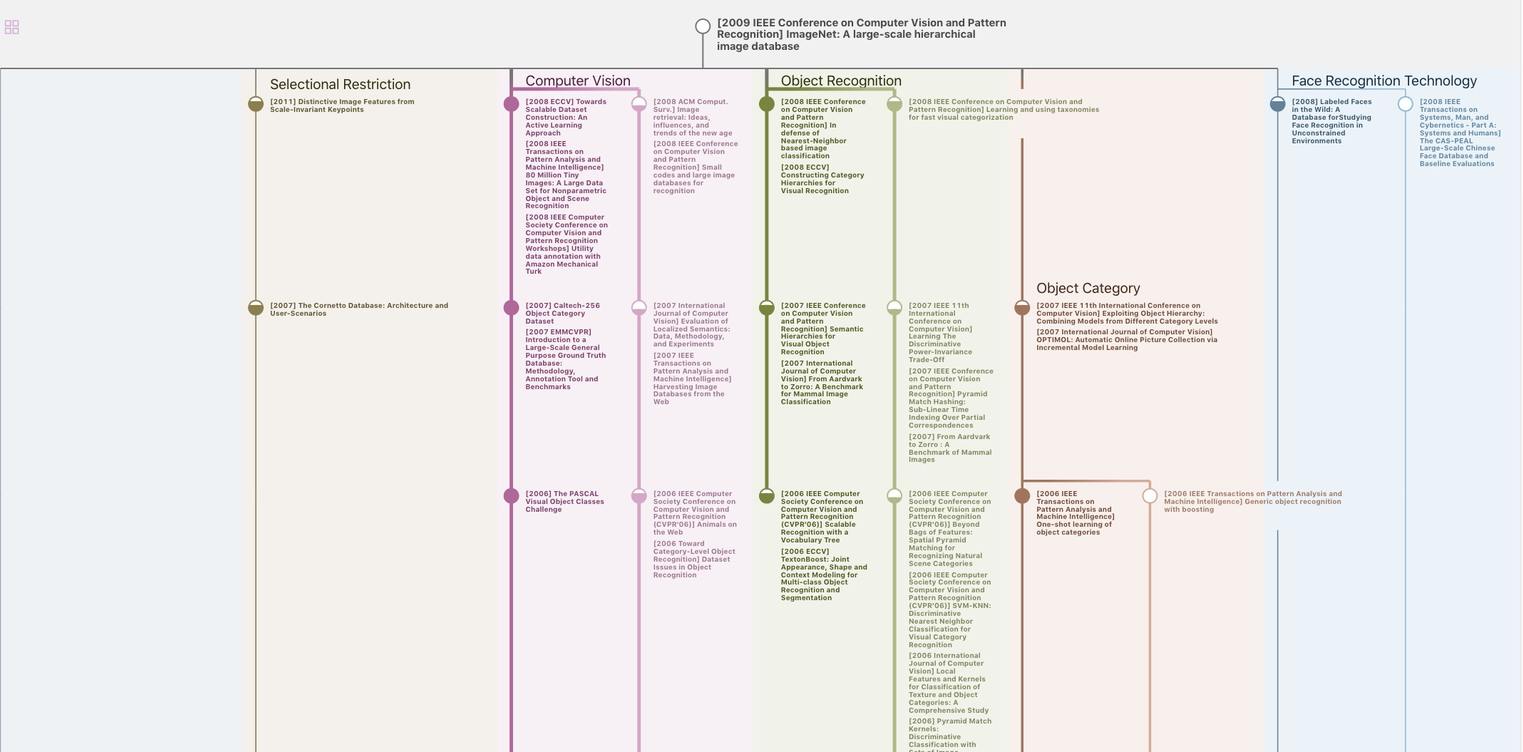
Generate MRT to find the research sequence of this paper
Chat Paper
Summary is being generated by the instructions you defined