Acoustic Scene Classification Using Aggregation of Two-Scale Deep Embeddings.
ICCT(2021)
Abstract
Acoustic scene classification (ASC) is a topic related to the field of machine listening whose important role is to recognize and categorize audio data in a predefined label which describes a scene location. In most of the state-of-the-art works for ASC, hand-crafted features and single-scale deep embeddings were adopted as the input of back-end classifiers. Inspired by the success of multi-scale deep embeddings in the field of computer vision, we propose an ASC method by aggregating two-scale deep embeddings that are independently learned by two convolutional neural networks (CNNs). We perform ASC experiments on two official datasets of the challenge on Detection and Classification of Acoustic Scenes and Events (DCASE), i.e., DCASE-2019 and DCASE-2017. Experimental results show that the proposed method using the aggregation of two-scale deep embeddings improves the performance of the ASC system. The proposed method obtains the improvement of classification accuracies by 0.11 and 0.09 on DCASE-2019 and DCASE-2017 respectively compared to the baseline system. Code is available: https://github.com/hokachon/Two-scale-Agg.
MoreTranslated text
Key words
acoustic scene classification,two-scale deep embedding,convolutional neural network
AI Read Science
Must-Reading Tree
Example
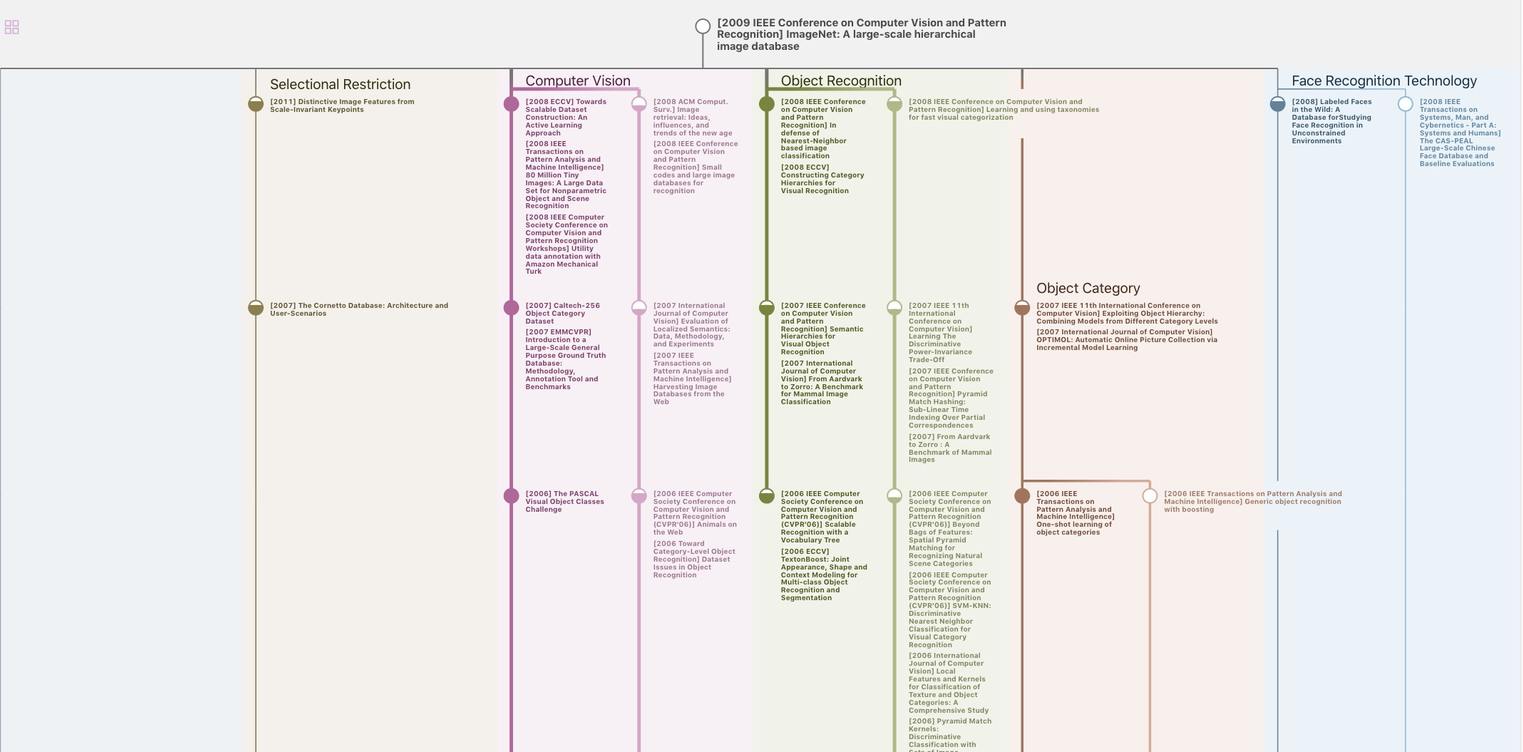
Generate MRT to find the research sequence of this paper
Chat Paper
Summary is being generated by the instructions you defined