ECNN - One Online Deep Learning Model for Streaming Ocean Data Prediction.
ICEA(2021)
摘要
Despite been extensively explored, current techniques in sequential data modeling and prediction are generally designed for solving regression tasks in a batch learning setting, making them not only computationally inefficient but also poorly scalable in real-world applications, especially for real-time intelligent ocean data quality control (QC), where the data arrives sequentially and the QC should be conducted in real time. This paper investigates the online learning for ocean data streams by resolving two main challenges: (i) how to develop a deep learning model to capture the complex ocean data distribution that could evolve dynamically, namely tackling the 'concept drift' problem for non-stationary time series; (ii) how to develop a deep learning model that can dynamically adapt its structure from shallow to deep with the inflow of the data to overcome under-fitting problem, namely tackling the 'model selection' problem. To tackle these challenges, we propose one Evolutive Convolutional Neural Network (ECNN) that dynamically re-weighting the sub-structure of the model from data streams in a sequential or online learning fashion, by which the capacity scalability and sustainability are introduced into the model. The experiments on real ocean observation data verify the effectiveness of our model. As far as we know, it is the first work that introduce online deep learning techniques into ocean data prediction research.
更多查看译文
AI 理解论文
溯源树
样例
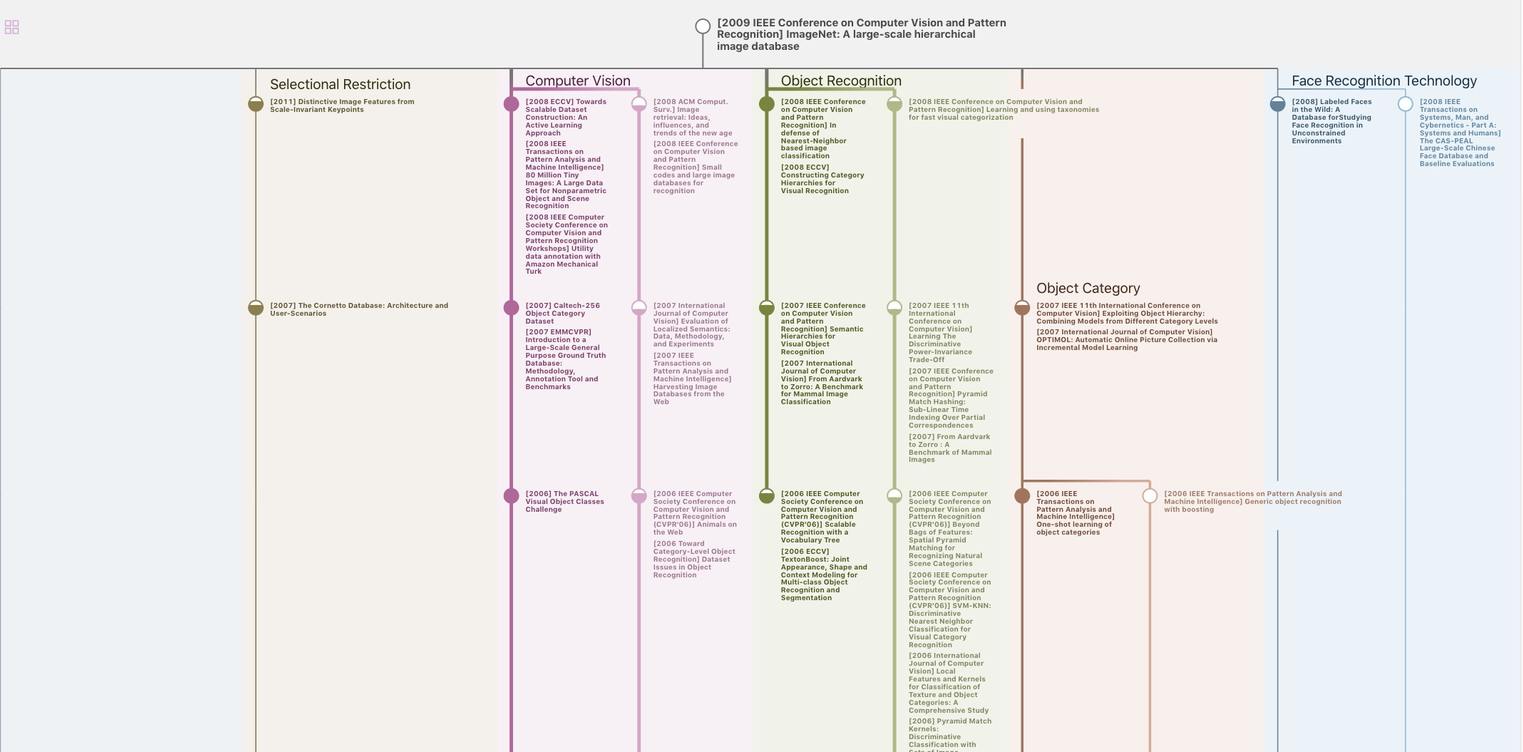
生成溯源树,研究论文发展脉络
Chat Paper
正在生成论文摘要