Malware Generation with Specific Behaviors to Improve Machine Learning-based Detection.
IEEE BigData(2021)
摘要
We describe efforts in generating synthetic malware samples that have specified behaviors that can then be used to train a machine learning (ML) algorithm to detect behaviors in malware. The idea behind detecting behaviors is that a set of core behaviors exists that are often shared in many malware variants and that being able to detect behaviors will improve the detection of novel malware. However, empirically the multi-label task of detecting behaviors is significantly more difficult than malware classification, only achieving on average 84% accuracy across all behaviors as opposed to the greater than 95% multi-class or binary accuracy reported in many malware detection studies. One of the difficulties in identifying behaviors is that while there are ample malware samples, most data sources do not include behavioral labels, which means that generally there is insufficient training data for behavior identification. Inspired by the success of generative models in improving image processing techniques, we examine and extend a 1) conditional variational auto-encoder and 2) a flow-based generative model for malware generation with behavior labels. Initial experiments indicate that synthetic data is able to capture behavioral information and increase the recall of behaviors in novel malware from 32% to 45% without increasing false positives and to 52% with increased false positives.
更多查看译文
关键词
generative models,machine learning,deep learning,malware detection
AI 理解论文
溯源树
样例
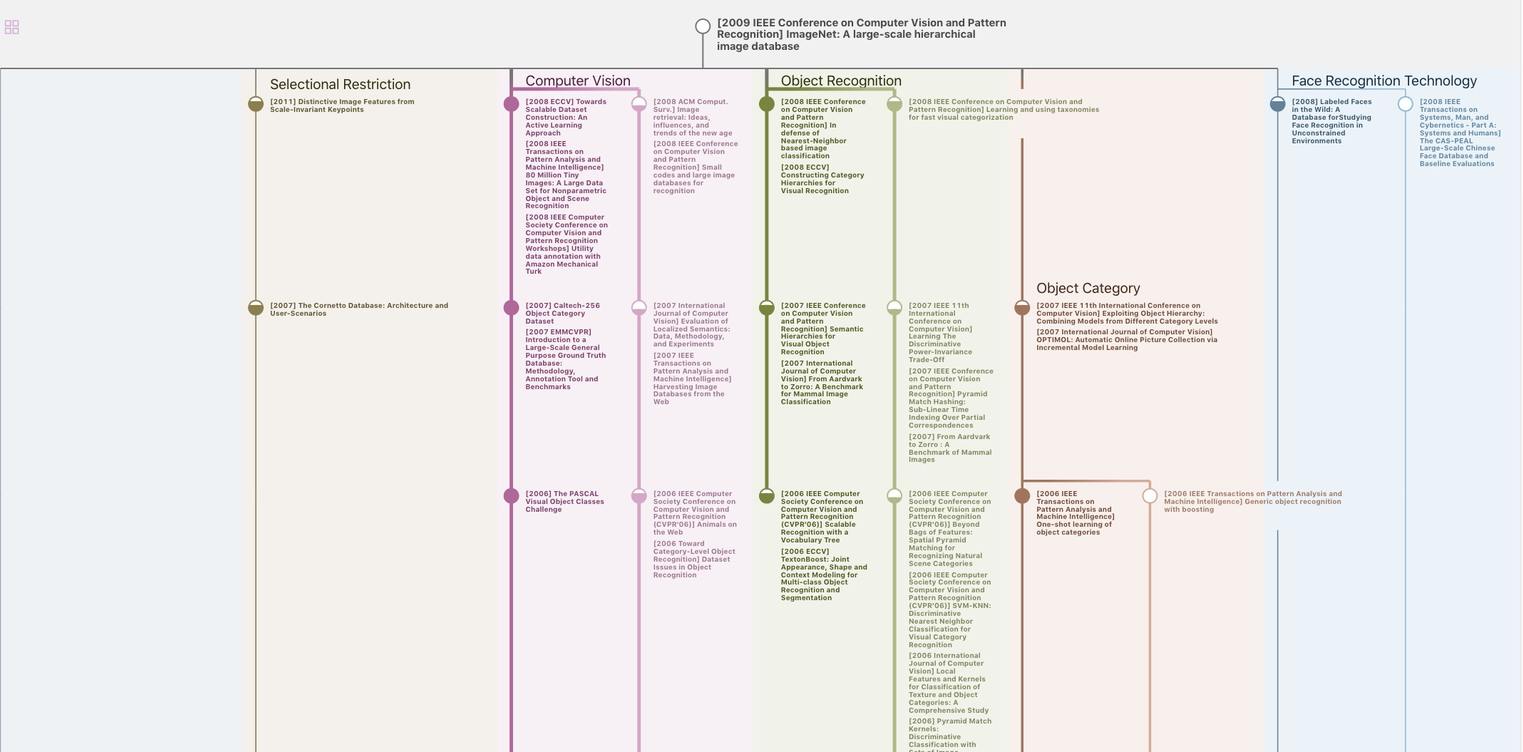
生成溯源树,研究论文发展脉络
Chat Paper
正在生成论文摘要