Stacked LSTM Based Wafer Classification.
IEEE BigData(2021)
Abstract
The sensors are used to analyze the quality of wafers in wafer manufacturing industries. The data from sensors is very helpful in framing solutions to predict the pass or fail status of the wafer by classifying high dimensional sensor data using machine learning techniques. In this work, we propose a stacked long short term memory (LSTM) approach i.e., a seq2seq architecture suitable for time-series data. We perform an exhaustive empirical analysis of the proposed model on the Seagate soft sensing dataset. The evaluation metric used is ROC-AUC score. The proposed stacked LSTM approach gave a best ROC-AUC score of 0.7445 on validation data and 0.729 on test data, which is significantly better than the baseline models.
MoreTranslated text
Key words
Soft Sensing,Multivariate Time Series Classification,LSTM,Regularization,Wafer Manufacturing
AI Read Science
Must-Reading Tree
Example
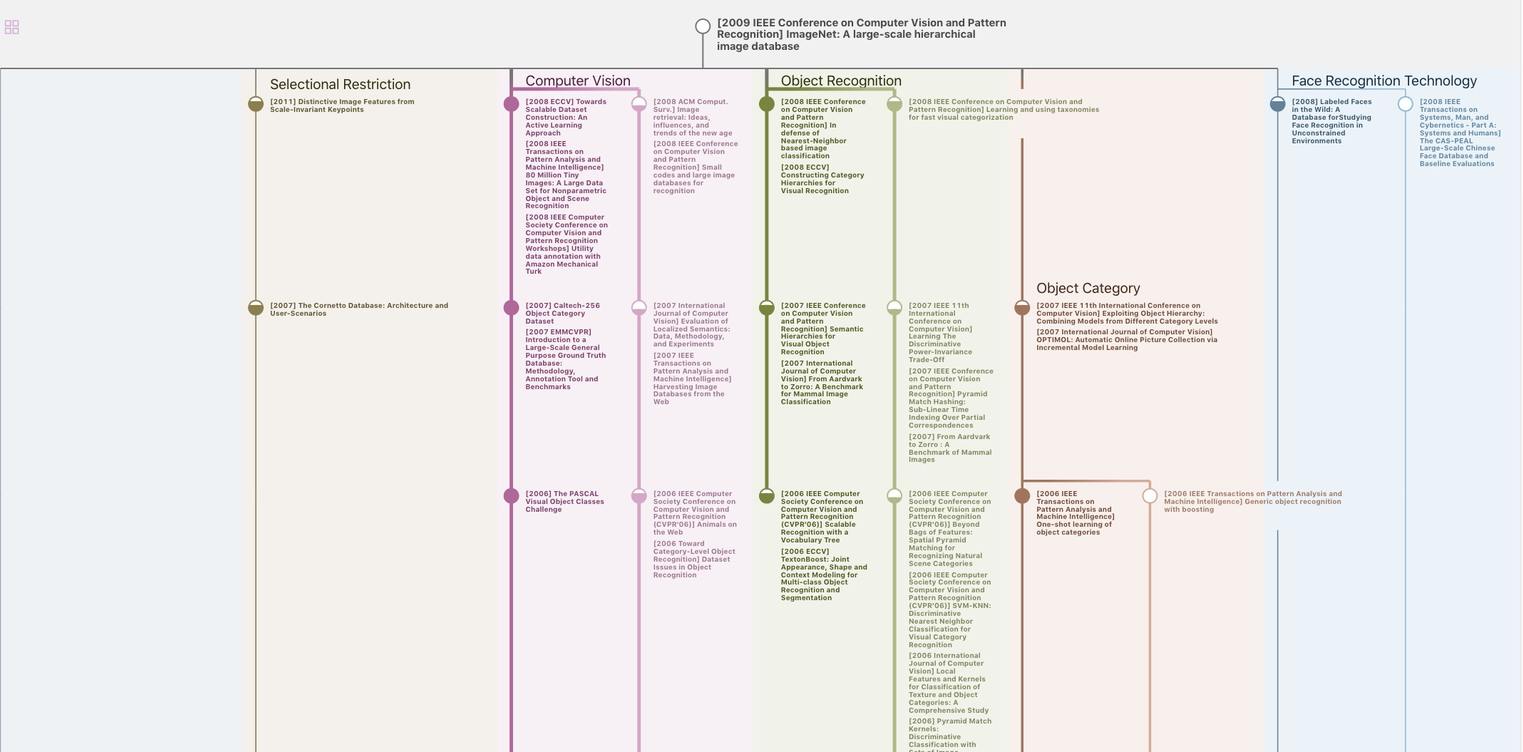
Generate MRT to find the research sequence of this paper
Chat Paper
Summary is being generated by the instructions you defined