Multi-Task Deep Learning Based Spatiotemporal Arctic Sea Ice Forecasting.
IEEE BigData(2021)
Abstract
Critical natural resources and processes in the Arctic depend heavily on sea ice. Thus, accurate and timely predictions of Arctic sea ice changes is important. Arctic sea ice forecasting involves two connected tasks: predicting sea ice concentration (SIC) at each pixel and predicting overall sea ice extent (SIE). Instead of having two separate models for these two forecasting tasks, in this paper we study how to use multi-task learning techniques and leverage the connections between ice concentration and ice extent to improve accuracy for both forecasting tasks. Because of the spatiotemporal nature of the data, we designed two novel multi-task learning models based on the CNN and ConvLSTM, respectively. Further, in conjunction with multi-task models, we developed custom loss functions which train the models to ignore land pixels and optimize for both concentration and extent when making predictions. Our experiments show that multi-task models provide better accuracy for a 1-month lead time than models that predict sea ice extent and concentration separately. Our accuracies are better than or comparable to results in related state-of-the-art studies. Our best model in SIC prediction outperformed the best existing SIC prediction model in the literature with 1.78% less error, and our best model in SIE prediction outperformed the best existing SIE prediction model with 0.283 million km(2) less error.
MoreTranslated text
Key words
arctic sea ice forecasting,convolutional neural network (CNN),convolutional long short-term memory (ConvL-STM) network,multi-task deep learning,custom loss function
AI Read Science
Must-Reading Tree
Example
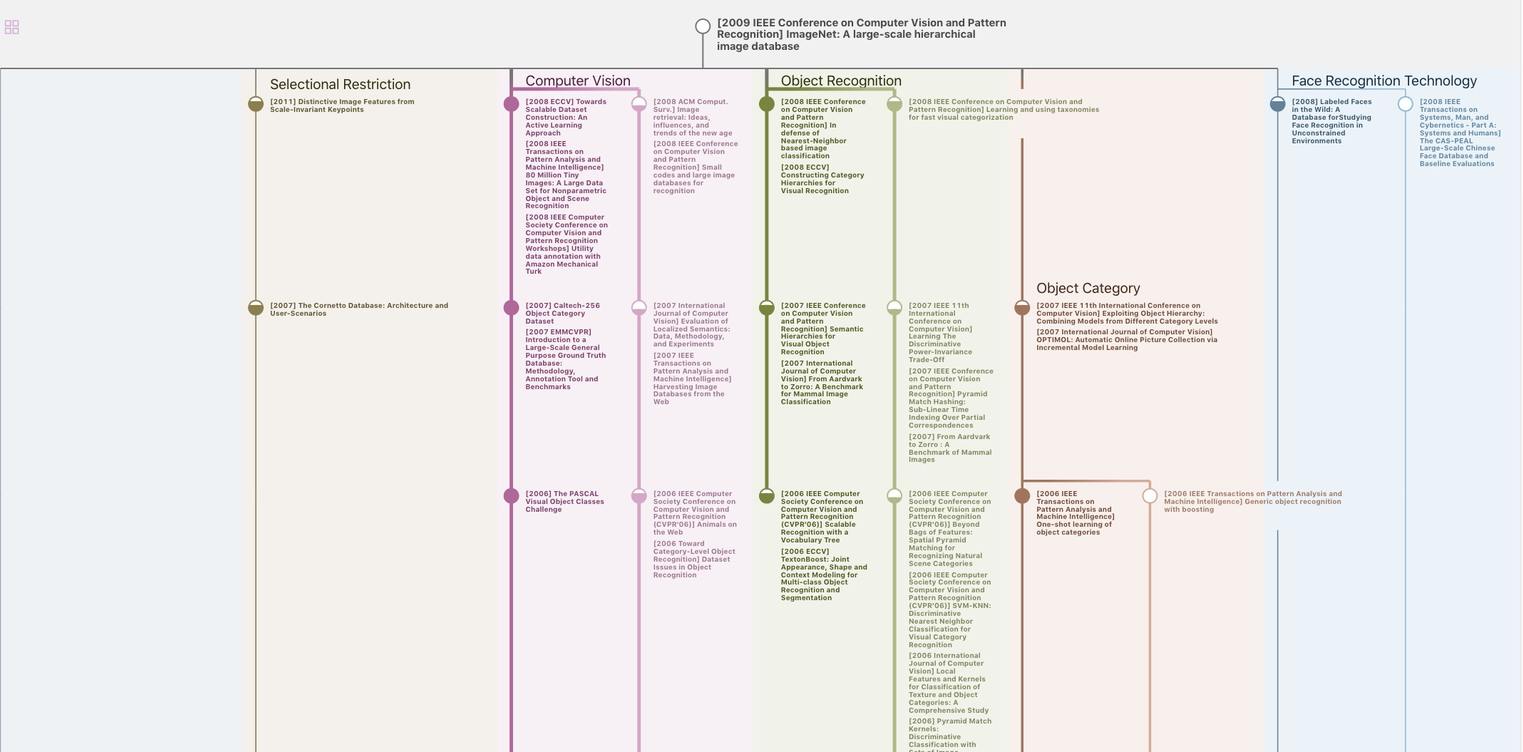
Generate MRT to find the research sequence of this paper
Chat Paper
Summary is being generated by the instructions you defined