HyperASPO - Fusion of Model and Hyper Parameter Optimization for Multi-objective Machine Learning.
IEEE BigData(2021)
摘要
Current state of the art methods for generating Pareto-optimal solutions for multi-objective optimization problems mostly rely on optimizing the hyper-parameters of the models (HPO - hyper-parameter Optimization). Few recent, less studied methods focus on optimizing over the space of model parameters, leveraging the problem specific knowledge. We present a generic first-of-a-kind method, referred to as HyperASPO, that combines optimization over the spaces of both hyper-parameters and model parameters for multi-objective optimization of learning problems. HyperASPO consists of two stages. First, we perform a coarse HPO to determine a set of favorable hyperparameter configurations. In the second step, for each of these configurations, we solve a sequence of weighted single objective optimization problems for estimating Pareto-optimal solutions. We generate the weights in the second step using an adaptive mesh constructed iteratively based on the metrics of interest, resulting in further refinement of Pareto frontier efficiently. We consider the widely used XGBoost (Gradient Boosted Trees) model and validate our method on multiple classification datasets. Our proposed method shows up to 20% improvement over the hypervolumes of Pareto fronts obtained through state of the art HPO based methods with up to 2x reduction in computational time.
更多查看译文
关键词
Hyperparameter optimization,Model parameters,XGBoost,HyperASPO,Pareto Optimization
AI 理解论文
溯源树
样例
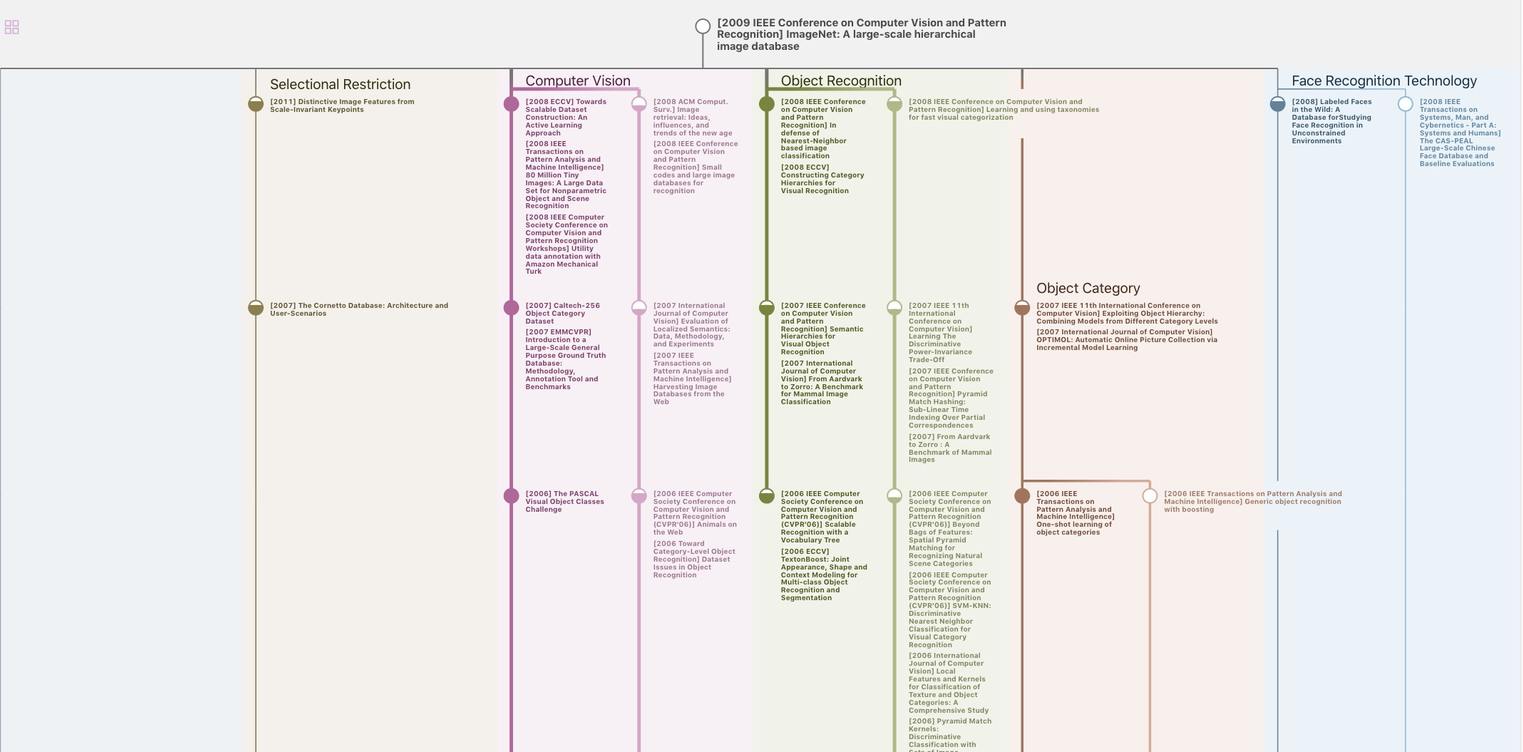
生成溯源树,研究论文发展脉络
Chat Paper
正在生成论文摘要