CPQN: Central Product Quantization Network for Semi-supervised Image Retrieval
IEEE BigData(2021)
摘要
The hash method or product quantization based on deep learning has achieved great success in image retrieval. But most deep hash methods are designed for supervised scenes. They only use semantic similarity information and ignore the underlying data structure. Moreover, a large amount of manual label information is expensive and time-consuming, which is not in line with the actual application scenario. In order to tackle this problem, we propose a novel quantization-based semi-supervised image retrieval network: Central Product Quantization Network (CPQN). We design a novel central similarity strategy to preserve the semantic similarity and underlying data structure in labeled data, and generalize it to unlabeled data through consistent regularization to tap the potential of unlabeled data. We also propose a novel semi-supervised loss algorithm to achieve effective hashing by reducing quantization noise and minimizing the empirical error of labeled data and the embedding error of unlabeled data. Experiments on public benchmark dataset clearly show that our proposed method is superior to the most advanced hash method.
更多查看译文
关键词
image retrieval,product quantization,deep hashing,semi-supervised
AI 理解论文
溯源树
样例
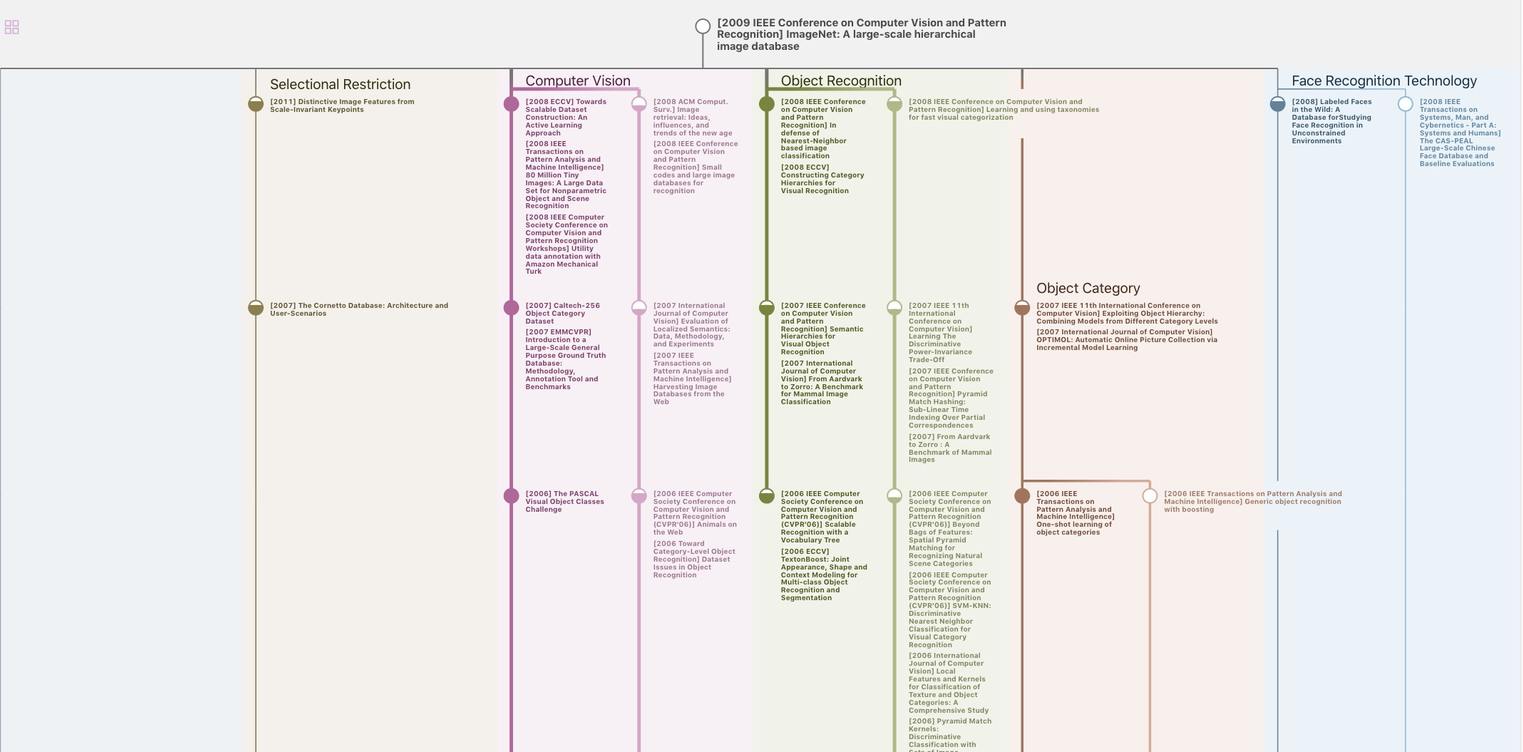
生成溯源树,研究论文发展脉络
Chat Paper
正在生成论文摘要