A Hierarchical Attention Graph Convolutional Network for Traffic Incident Impact Forecasting.
IEEE BigData(2021)
摘要
Predicting the impact of traffic i ncidents b ased on traffic s ensor d ata i s a n e ssential r esearch t opic i n t he fi eld of Intelligent Transportation Systems (ITS). Tackling the problem of estimating the durations of incidents from their early stages is a challenge due to the variable nature of such incidents and the complex structure of modern road networks. Existing studies on forecasting the incident duration from sensor data are mostly incapable of modeling 1) the spatiotemporal correlations of traffic s ensors a nd a rterial r oads a nd 2) t he hierarchical topology of the traffic sensor and road networks. In this paper, we propose the Hierarchical Attention-based Spatiotemporal Graph Convolutional Network model (HastGCN) to solve the incident duration forecasting problem by formulating the spatiotemporal correlation and traffic p atterns o n b oth t he s ensor l evel and the road level in their natural hierarchical manner. At the sensor level, we propose a spatiotemporal attention mechanism followed by graph convolutions to model the local correlations and patterns between traffic s ensors o n t he s ame a rterial road. At the road level, a connectivity-aware attention mechanism is designed to learn the global spatial relatedness between each arterial road. Traffic-condition a ware g raph c onvolutions are then applied to understand the target incident representation for the incident duration forecasting.
更多查看译文
关键词
component,formatting,style,styling,insert
AI 理解论文
溯源树
样例
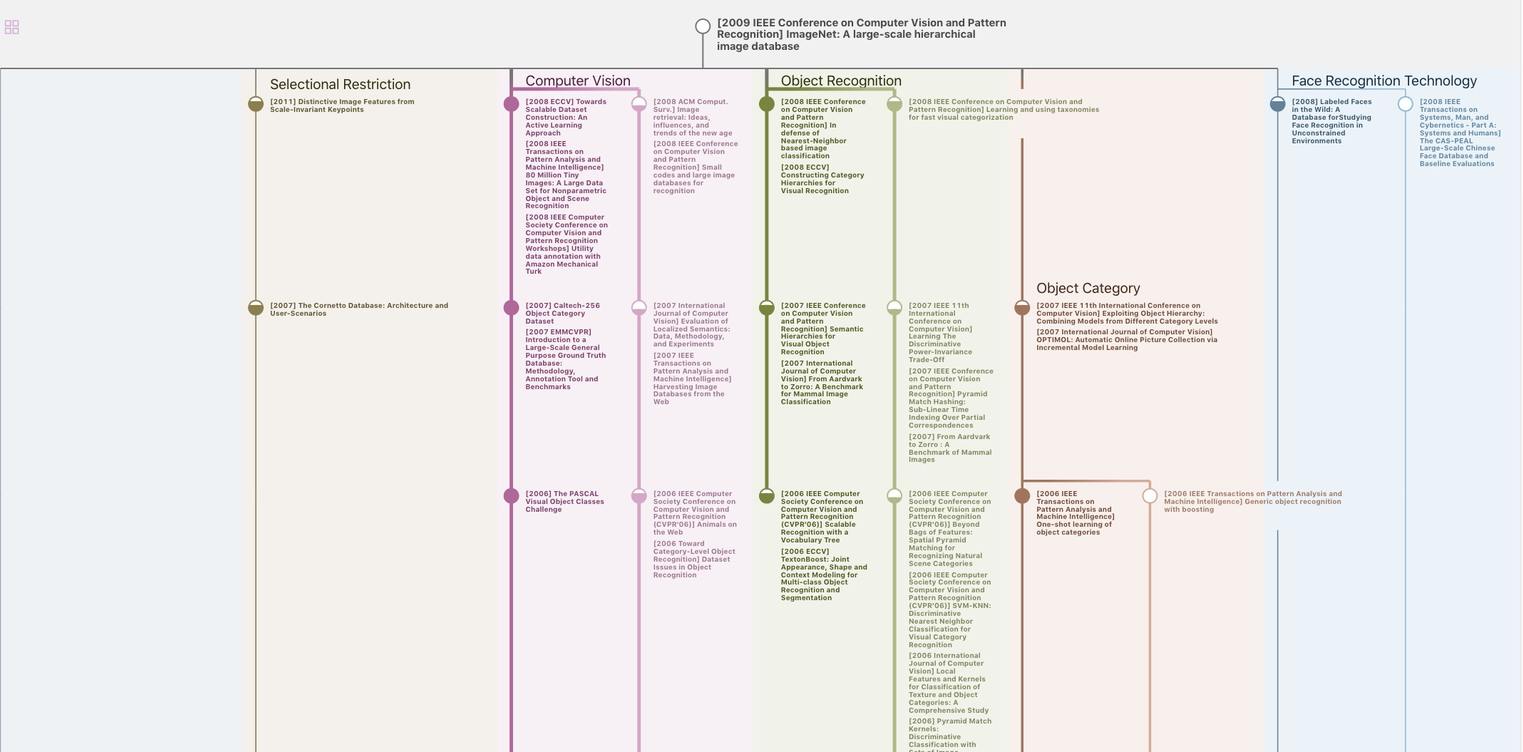
生成溯源树,研究论文发展脉络
Chat Paper
正在生成论文摘要