An Ensemble Method for the Heterogeneous Neural Network to Predict the Remaining Useful Life of Lithium-ion Battery.
SMC(2021)
摘要
With the large-scale application of lithium-ion batteries (LIB), using deep neural networks to predict the remaining useful life (RUL) of LIB has gradually become a hotshot in recent years. RUL prediction method based on deep neural network can avoid studying electrochemical phenomena and manual extracting the features in battery. But single neural network has the different prediction accuracy and features extraction on different dataset. In this study, an ensemble method for the heterogeneous neural network is proposed, which integrates the prediction results of multiple heterogeneous neural networks with the adaptive weight. The weight of the neural network is higher with the closer correlation to the majority prediction results, vice versa. Furthermore, the weight of the neural network is adjusted via the predicting results for neural network on the different dataset, so that the computed weight of the neural network is adapted to the various dataset, and the effects of poor predictions of certain neural networks can be reduced sufficiently. The effectiveness of the ensemble method is verified on MIT-Stanford LIB degradation dataset, and the results show that the proposed method has higher accuracy than the existing ensemble methods for neural network.
更多查看译文
关键词
Ensemble Method,Neural Network,Remaining Useful Life of Lithium-ion Battery,Adaptive Weight Computing
AI 理解论文
溯源树
样例
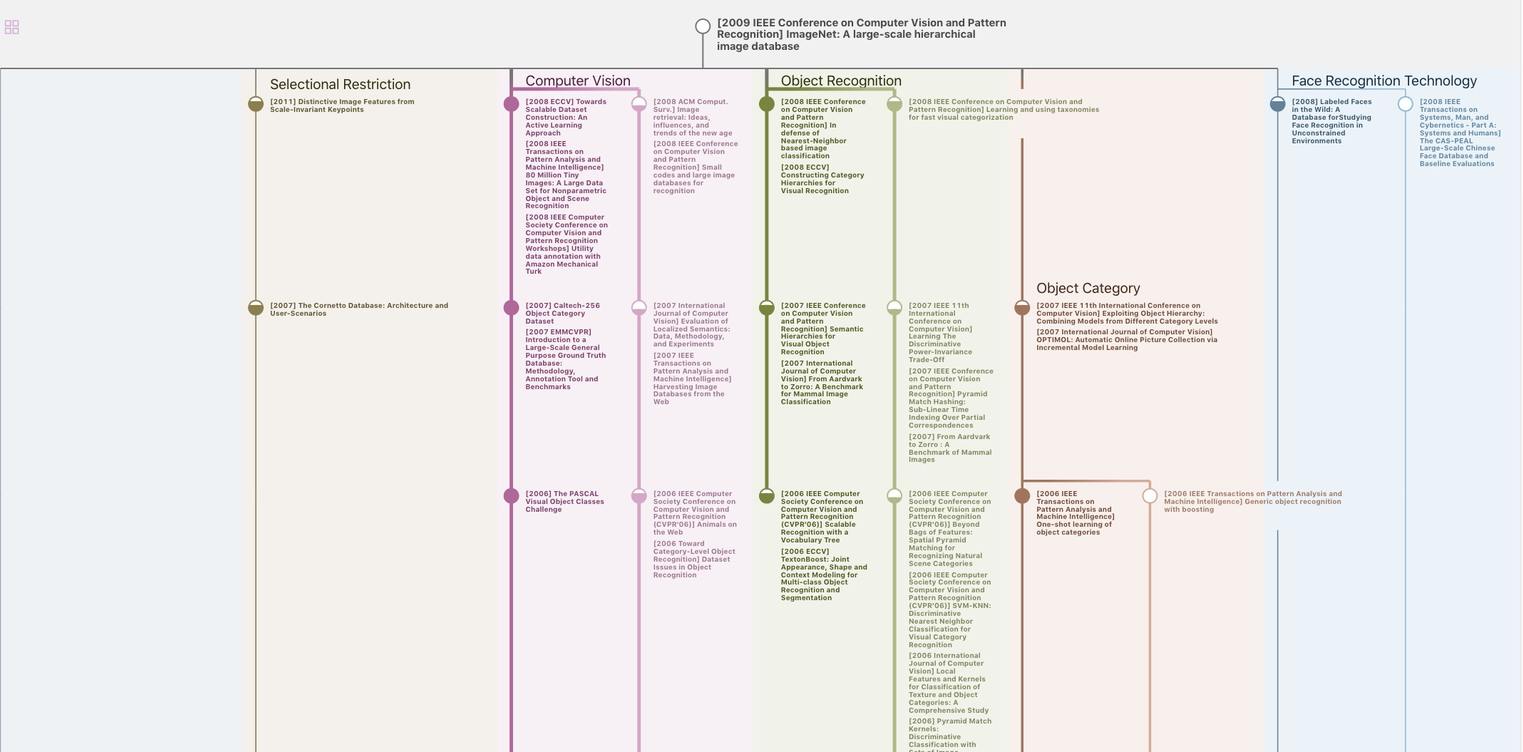
生成溯源树,研究论文发展脉络
Chat Paper
正在生成论文摘要