CTHGAT - Category-aware and Time-aware Next Point-of-Interest via Heterogeneous Graph Attention Network.
SMC(2021)
摘要
Location-based recommendation has become a significant method to help people locate fascinating and appealing points of interest (POIs) with the rapid popularity of smart mobile devices and the prevalence of location-based social networks (LBSN). However, the sparsity of the user-POI matrix and the cold-start issue have generated serious challenges, resulting in a substantial decrease in collaborative filtering methods' recommendation results. In reality, location-based recommendation demands spatiotemporal context awareness. In order to overcome these challenges, we develop an embedding model based on the heterogeneous graph attention network. Geographic influence, social relation and historical check-in influence are captured in a unified way by constructing a user-POI heterogeneous graph. Subsequently, we use the LSTM-based model to learn the category weight of the next POI to select. We are developing a score function to recommend the next POI for users by integrating category weights, user preferences and time impact. We conduct experiments on existing large-scale datasets to evaluate the performance of our model. The results demonstrate our proposal is superior to other rivals. Additionally, our method has been significantly improved compared with other competitive approaches in terms of recommending cold-start POI.
更多查看译文
关键词
POI,recommendation,social network,LSTM,heterogeneous graph attention network
AI 理解论文
溯源树
样例
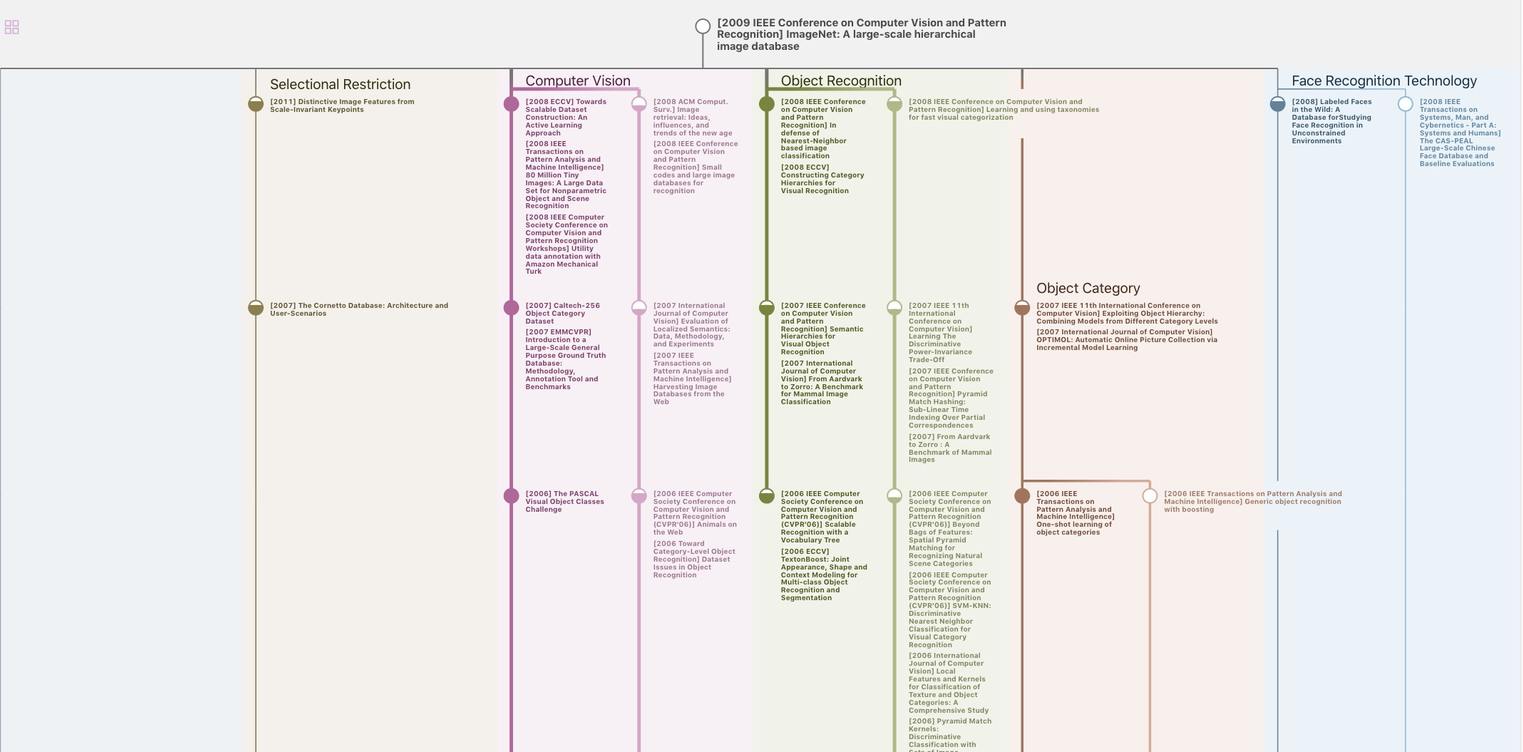
生成溯源树,研究论文发展脉络
Chat Paper
正在生成论文摘要