Provenance as a Substrate for Human Sensemaking and Explanation of Machine Collaborators.
SMC(2021)
摘要
Building and evaluating explainable Artificial Intelligence (AI) systems that accommodate human cognition remains a challenge for Human-Computer Interaction (HCI) and the need for practical solutions increases with our reliability on machines to extract, classify, and process information. Recent work has proposed triggers and metrics for explainable AI based on human mental models and psychological explanation quality. We complement this previous work by (1) extending and supporting these triggers and metrics with existing directives for information integrity, transparency, and rigor, (2) outlining a provenance-based framework for recording human-machine collaboration, and (3) demonstrating that a provenance-based approach address many of these explainable AI triggers and metrics. We show that provenance-based analyses help address questions of foundations, alternatives, necessity vs. sufficiency, sensitivity (e.g., what-if analyses), impact, and rationale, and we provide concrete evidence using an implemented human-machine analytic workspace. We outline ways to empirically measure the ability of these additional interpretation strategies to improve human understanding.
更多查看译文
关键词
human sensemaking,machine collaborators,human cognition,human-computer interaction,information processing,human mental models,psychological explanation quality,information integrity,provenance-based framework,human-machine collaboration,provenance-based analyses,human-machine analytic workspace,human understanding,information extraction,information classification,explainable AI
AI 理解论文
溯源树
样例
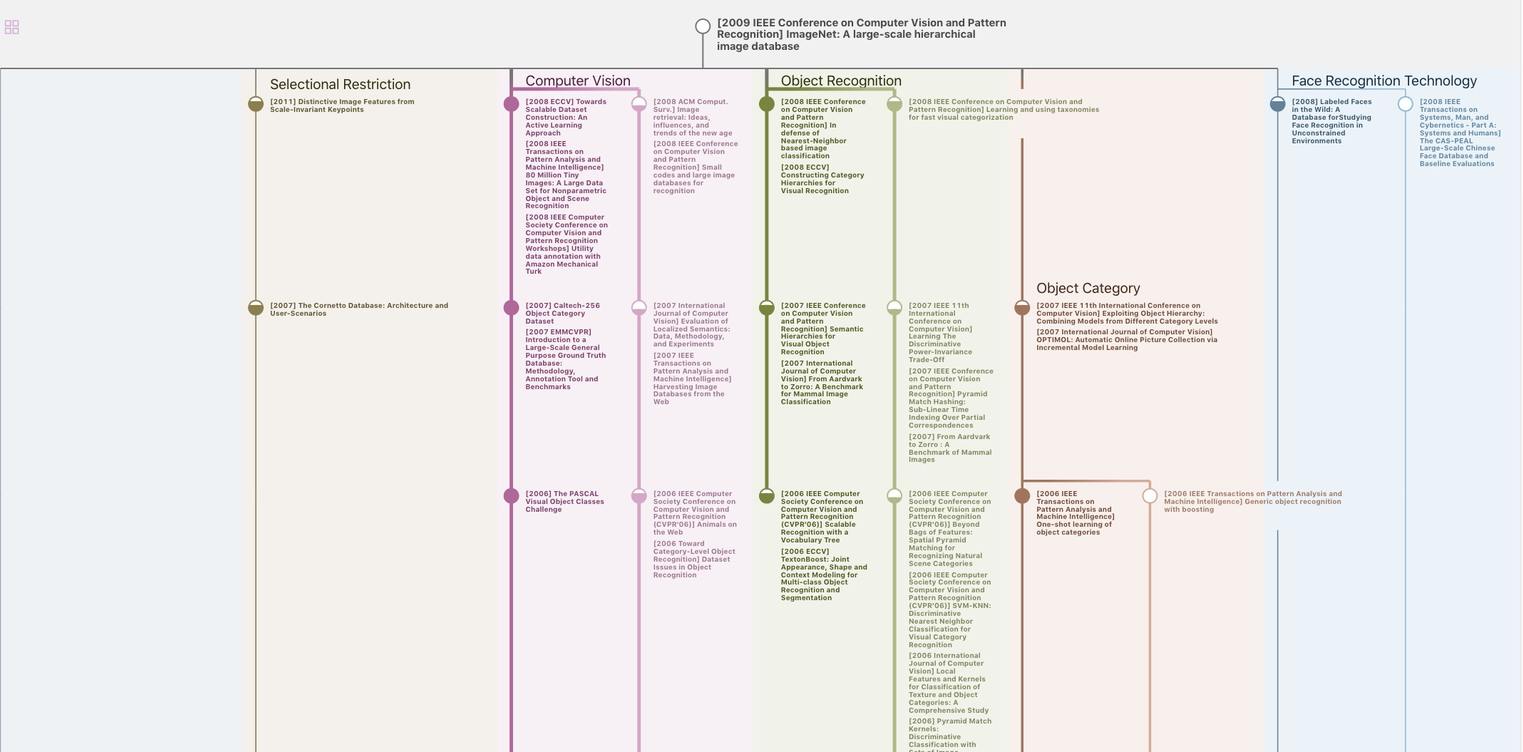
生成溯源树,研究论文发展脉络
Chat Paper
正在生成论文摘要