Evaluation of Compressed Residential Energy Forecasting Models.
SMC(2021)
摘要
Embedded energy gateways are increasingly being implemented for monitoring and control tasks in smart energy applications in buildings, cities, and localized electrical grids. These leverage state-of-the-art computational intelligence models for forecasting and anomaly detection. We present a study comparing various neural network model implementations for residential energy forecasting, both full-size models and compressed models using weight quantization and network pruning techniques. The evaluation is carried out on publicly available data consisting of 23 homes from the reference Pecan Street database. We analyse the optimal trade-off between accuracy, in terms of MSE quantitative metrics, and model complexity as reflected by network memory footprint for resource constrained embedded platforms. Using a pre-determined MSE threshold for residential energy forecasting, we achieve higher than 50% memory footprint reduction compared to the baseline scenario, using a combination of weight pruning and quantization, for both individual homes and global models that are trained across time series.
更多查看译文
关键词
forecasting,models,energy
AI 理解论文
溯源树
样例
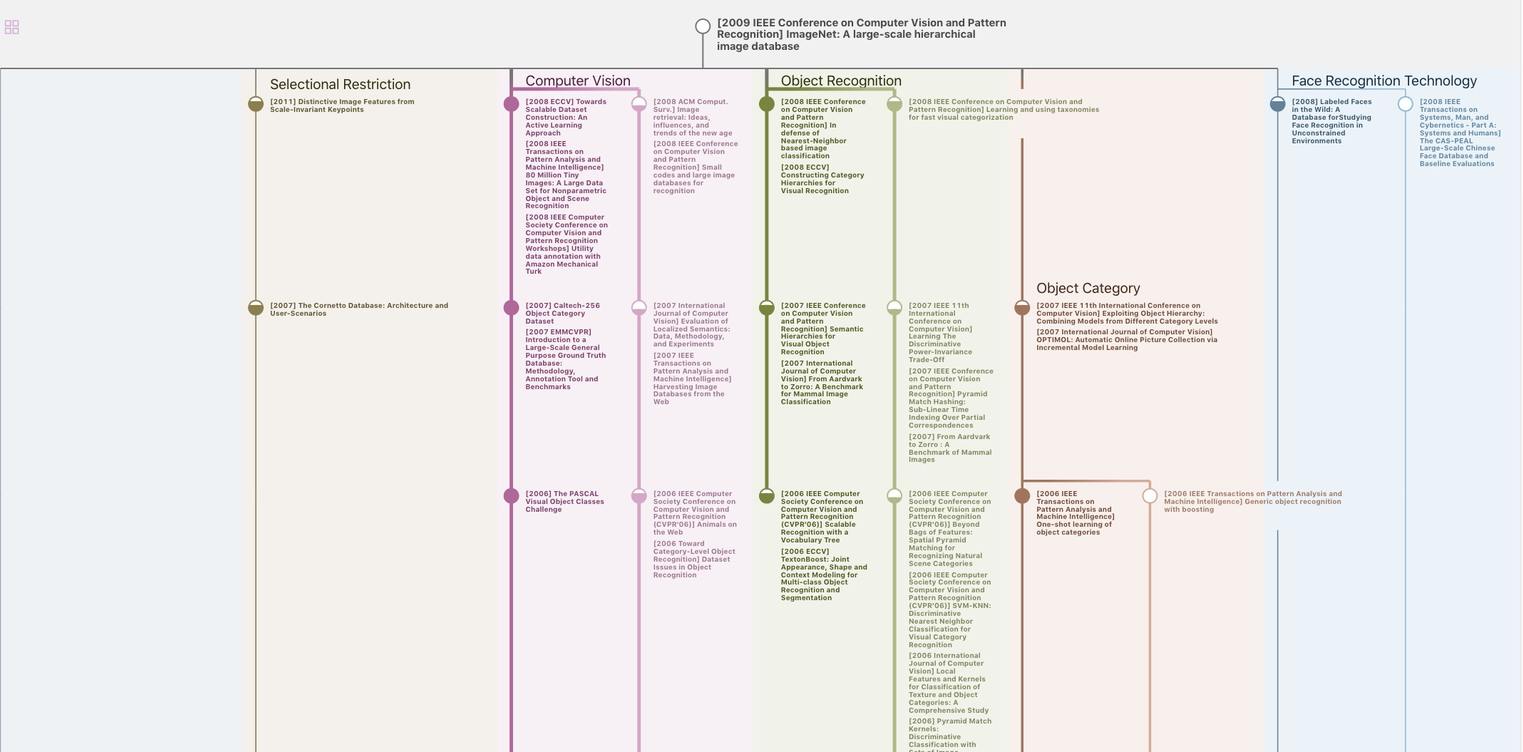
生成溯源树,研究论文发展脉络
Chat Paper
正在生成论文摘要