PIDLNet: A Physics-Induced Deep Learning Network for Characterization of Crowd Videos
2021 17th IEEE International Conference on Advanced Video and Signal Based Surveillance (AVSS)(2021)
Abstract
Human visual perception regarding crowd gatherings can provide valuable information about behavioral movements. Empirical analysis on visual perception about orderly moving crowds has revealed that such movements are often structured in nature with relatively higher order parameter and lower entropy as compared to unstructured crowd, and vice-versa. This paper proposes a Physics-Induced Deep Learning Network (PIDLNet), a deep learning framework trained on conventional 3D convolutional features combined with physics-based features. We have computed frame-level entropy and order parameter from the motion flows extracted from the crowd videos. These features are then integrated with the 3D convolutional features at a later stage in the feature extraction pipeline to aid in the crowd characterization process. Experiments reveal that the proposed network can characterize video segments depicting crowd movements with accuracy as high as 91.63%. We have obtained overall AUC of 0.9913 on highly challenging publicly available video dataset. The method outperforms existing deep-learning frameworks and conventional crowd characterization frameworks by a notable margin.
MoreTranslated text
Key words
deep learning,physics-induced
AI Read Science
Must-Reading Tree
Example
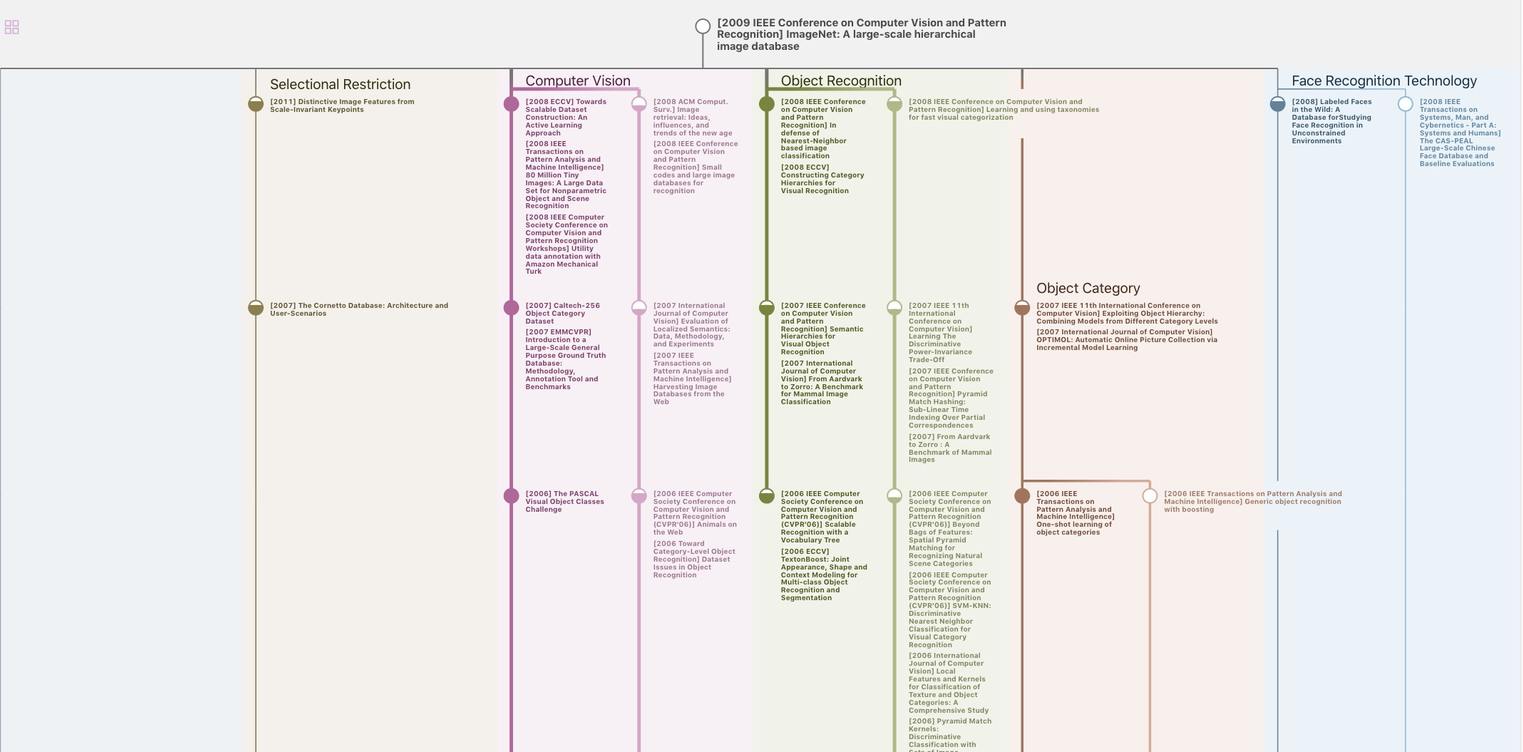
Generate MRT to find the research sequence of this paper
Chat Paper
Summary is being generated by the instructions you defined