A New Approach for Mining Correlated Frequent Subgraphs
ACM TRANSACTIONS ON MANAGEMENT INFORMATION SYSTEMS(2022)
摘要
Nowadays graphical datasets are having a vast amount of applications. As a result, graph mining-mining graph datasets to extract frequent subgraphs-has proven to be crucial in numerous aspects. It is important to perform correlation analysis among the subparts (i.e., elements) of the frequent subgraphs generated using graph mining to observe interesting information. However, the majority of existing works focuses on complexities in dealing with graphical structures, and not much work aims to perform correlation analysis. For instance, a previous work realized in this regard, operated with a very naive raw approach to fulfill the objective, but dealt only on a small subset of the problem. Hence, in this article, a new measure is proposed to aid in the analysis for large subgraphs, mined from various types of graph transactions in the dataset. These subgraphs are immense in terms of their structural composition, and thus parallel the entire set of graphs in real-world. A complete framework for discovering the relations among parts of a frequent subgraph is proposed using our new method. Evaluation results show the usefulness and accuracy of the newly defined measure on real-life graphical datasets.
更多查看译文
关键词
Association rules,branching factor,confidence measures,correlation discovery,data mining,graph mining
AI 理解论文
溯源树
样例
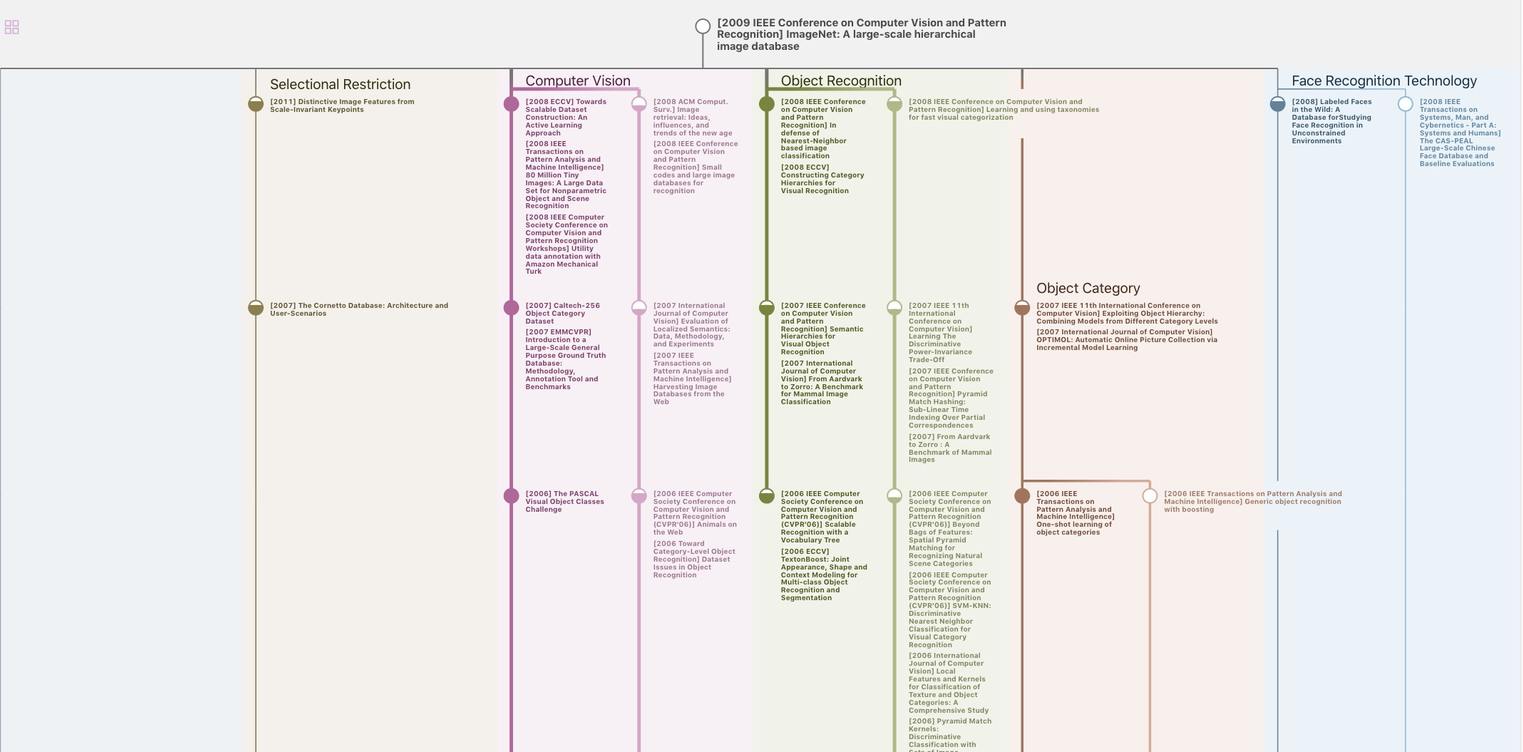
生成溯源树,研究论文发展脉络
Chat Paper
正在生成论文摘要