An Optimized Segmentation Scheme for Ambiguous Pixels Based on Improved FCN and DenseNet
CIRCUITS SYSTEMS AND SIGNAL PROCESSING(2021)
摘要
These past six years have witnessed that segmentation algorithms make a breakthrough due to the development of deep-based semantic segmentation networks, which can realize classification from image-level to pixel-level. However, when segmenting a small-batch, complex-features and obvious-gap database, these semantic segmentation networks will lead to segmentation ambiguities in some boundaries between foreground (i.e. the main segmentation body) and its background with limited image detail expression. To solve such problem, this paper proposes an optimized segmentation scheme for ambiguous pixels based on Atrous-ResFCN and MFR-DenseNet, namely ARMD. Firstly, masking algorithm and adaptive box mechanism are applied in pre-processed KITTI segmentation database to construct the classification database, which will be used to train ambiguities judgment model. In addition, Atrous-ResFCN-8s and Atrous-ResFCN-16s are proposed and further combined to determine segmentation ambiguities with different-level segmentation abilities. Finally, MFR-DenseNet is further migrated to optimize these ambiguous pixels with effective threshold selection. Experimental results demonstrate that our proposed ARMD algorithm is beneficial to improve segmentation accuracy, where the highest MIoU and PA are able to reach 88.18% and 95.88%, respectively.
更多查看译文
关键词
Semantic segmentation, Image classification, Region of interest, Ambiguous pixels, Atrous-ResFCN, MFR-DenseNet
AI 理解论文
溯源树
样例
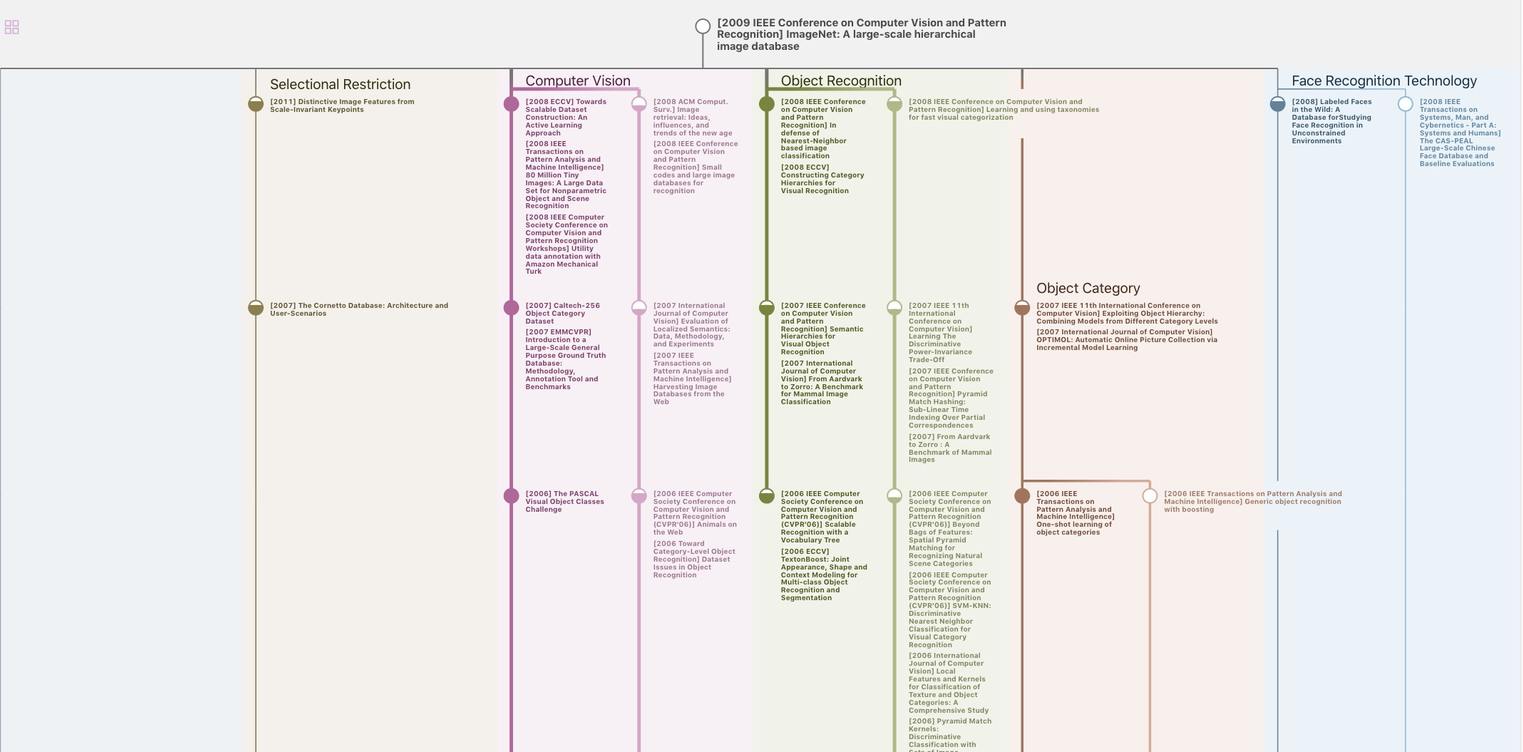
生成溯源树,研究论文发展脉络
Chat Paper
正在生成论文摘要